The MLPerf 0.7 results for inferencing are out. This is a new major release. Unlike benchmarks such as SPEC’s CPU2006 then CPU2017, MLPerf is swapping component tests on a regular basis. As a result, we have new benchmarks in this test. We still see NVIDIA and Intel featured heavily in the result list, but we now have partner OEMs joining. In this article, we are going to discuss some of the broad storylines.
MLPerf 0.7 Inference: The Test Mix Change
MLPerf 0.7 has added a few new benchmarks. Industry observers have noted that a benchmark where primarily only NVIDIA, Google, and Intel are playing, with NVIDIA dominating as they did in MLPerf 0.7 Training Results When Winners Only Apply, that the benchmark can lose meaning.
For this round on the MLPerf 0.7 Inferencing side, the group added a number of new benchmarks. Instead of just having Google, NVIDIA, and Intel pick the benchmarks, customer focus groups of a half-dozen or so customers were asked to give input on new benchmarks. This is still not necessarily a perfect set, nor a perfect benchmark, but there are efforts being made to make it representative of real customer workloads.
MLPerf 0.7 Inference’s NVIDIA Chorus
Something else has changed with this generation. Specifically, we see a number of new entries. First, there are a number of OEMs joining. Dell EMC, Inspur, QCT, Lenovo, Fujitsu, and Gigabyte all joined the effort. Cisco and Atos did as well. This is a big change over the previous iterations where it was mostly Intel, NVIDIA, and Google submitting for the data center and much of the Edge Closed areas.
When one drills down into the numbers, a few things become clear. It seems as though NVIDIA heard its criticism around the lack of OEM systems in MLPerf and went through an effort to rally OEMs to submit results. There are some differences in systems, but the key takeaway is that the same number of GPUs in a system generally leads to results +/- 5% or so of the average even with different systems Xeon and sometimes AMD EPYC CPUs used.
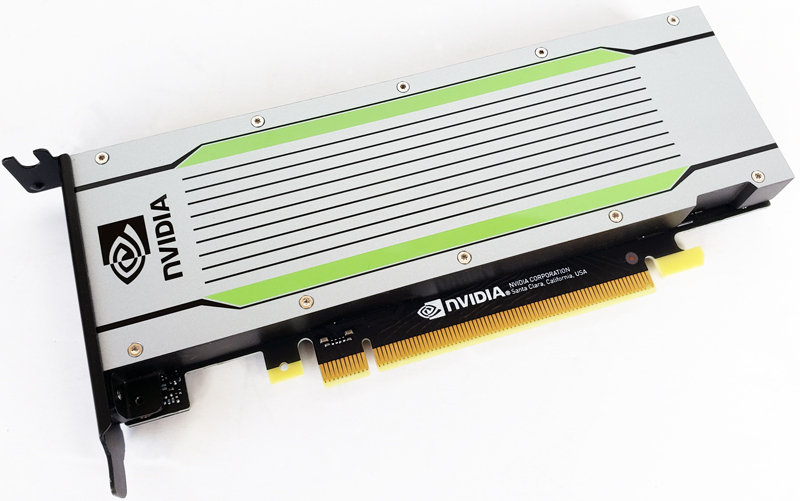
Since we focus on servers, and most of the data center results utilized NVIDIA GPUs, the key takeaway is basically buying the server with the most GPUs you can at the lowest price possible. That is somewhat similar to what we see with SPEC CPU2017 where vendors tend to be fairly close. The other key takeaway is that the new NVIDIA A100 is better than the much less expensive and lower power NVIDIA T4.
Perhaps the strangest part about MLPerf is that the key learning is that NVIDIA’s OEMs are not differentiating strongly on performance with NVIDIA hardware, so NVIDIA is the one creating the value for performance, the OEMs are simply a delivery mechanism. It is fairly hard to use the results to get to a different conclusion.
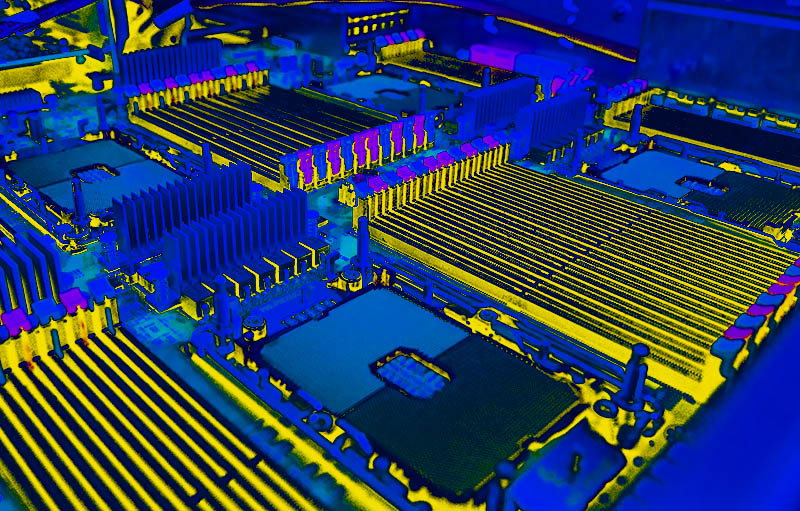
Intel added a few results for systems such as 4-socket and 8-socket “Cooper Lake” or 3rd Generation Intel Xeon Scalable processors. NVIDIA is generally faster which they are heavily marketing. Intel’s position is more that one gets their inferencing for “free” in existing servers. It is certainly asymmetric marketing angles on display.
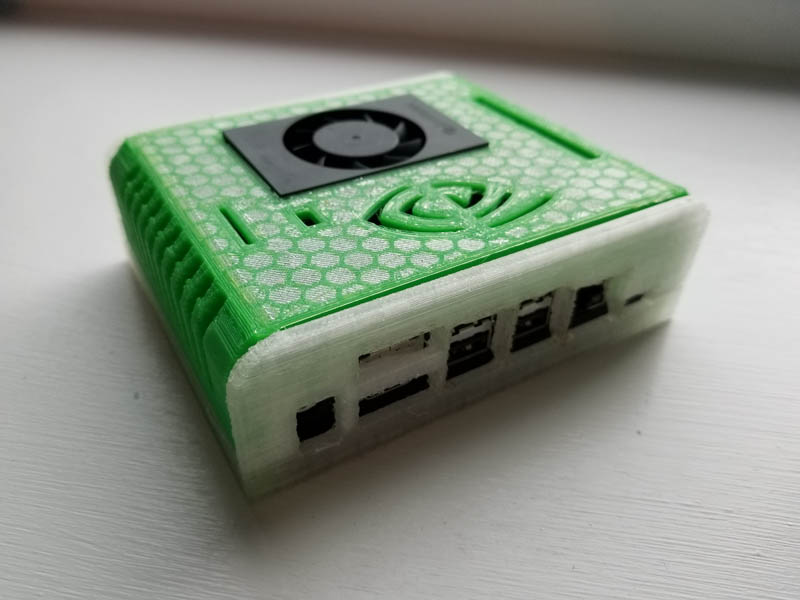
Google for its part was absent. As were other hyper-scalers. The one surprise was the number of Xilinx offerings. Xilinx had a few FPGA partners including Dell EMC showing off FPGA solutions and the Alveo U250 was featured a few times. The performance was not astounding, but it was included which is a step forward. We also saw the Centaur x86 inferencing cores make a debut that put in performance figures just beyond a NVIDIA Jetson Xavier NX.
We also had a number of entries on the “Edge Open” categories such as Raspberry Pi 4 and Firefly-RK3399 Rockchip DK3399 platforms on the list. There were also three mobile phones. Frankly, given how many chips and solutions are doing some kind of inferencing at the edge, it feels a bit strange that we have so many data center results and so few results from all of the hordes of AI edge processor solutions. Even Intel did not offer a MobileEye solution for benchmarking. NVIDIA added many Jetson numbers but we know the market is much bigger.
On the notebook side, Intel submitted the only result for both the closed and open categories so we are not sure how useful that is.
Final Words
MLPerf is still primarily an NVIDIA, Intel, and Google benchmark, albeit Google is absent in this round. The effort to include partners is good in this edition, but the partners are clearly being driven by NVIDIA, not their own agnostic push to show all of the available technologies. We did not see the plethora of Intel FPGAs, Habana Labs products, a teaser of the Xe GPU. We did not see any AMD solutions aside from the processors nor did we see some of the lower-end Xilinx parts. Companies such as Broadcom and NXP are not present. Dell EMC has a large investment in Graphcore and a number of other AI chip companies but they are not present. There are a ton of AI inferencing companies out there working on problems such as excelling at frames per watt rather than raw performance yet they are absent. We also do not have any Huawei or other inferencing AI chips present in this release despite AI inferencing being a huge investment area in China.
MLPerf is growing slowly, and this was a step in the right direction. Still, it still feels like a NVIDIA project with some participation from Intel and Google. It is not that we are seeing one or two holdout companies, it is the vast majority of the industry declining to participate.
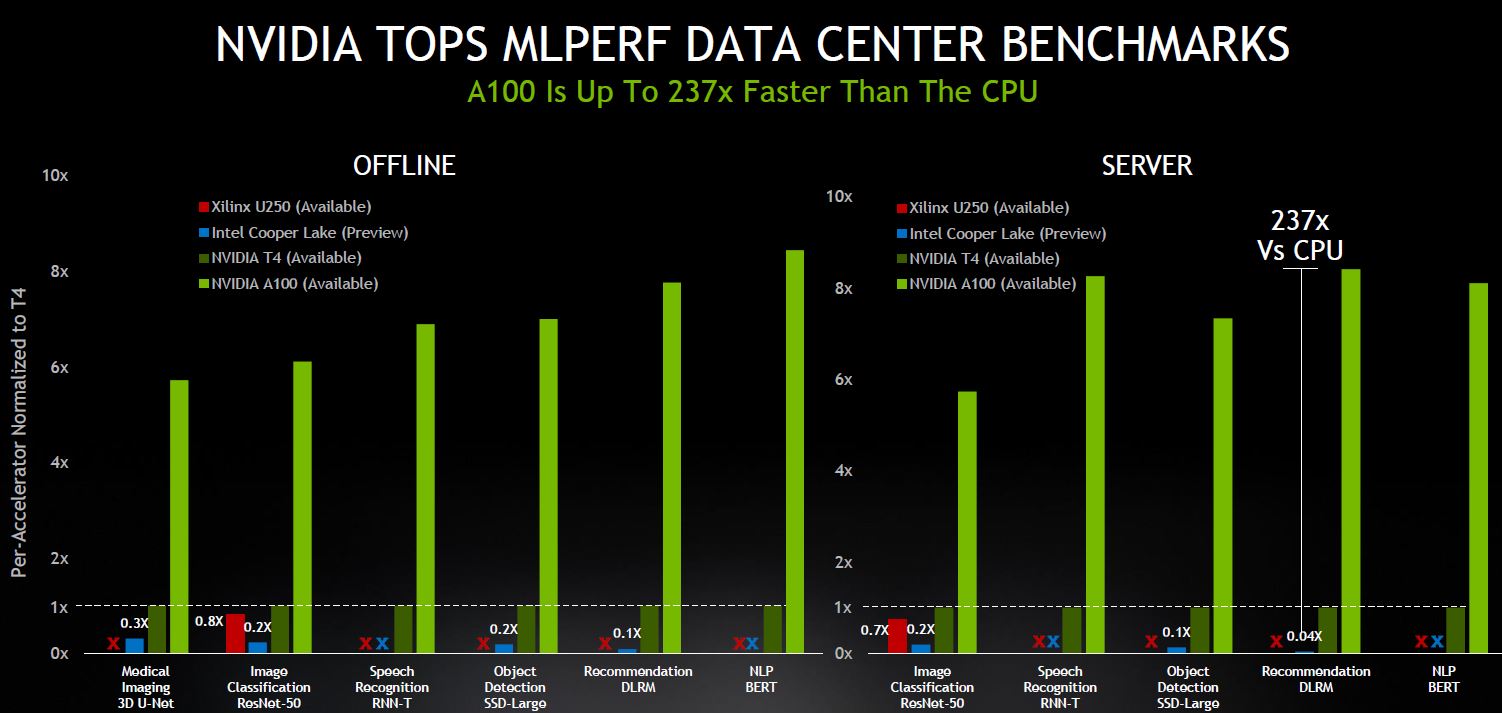
When NVIDIA invests heavily in MLPerf and the results look like the above, one can see why others would not want to play. Here NVIDIA is showing its 250-400W TDP GPUs versus what one effectively gets as “free” performance with Intel CPUs or in 225W cards with dual 100GbE interfaces that do not need host systems with the Xilinx U250. NVIDIA will argue that this is completely valid since they are showing the same workloads, however, they are comparing against solutions with different features. At some point, these charts are just going to keep other companies out of the project.

Again, this is just part of the process with any new benchmark, but we need to start seeing NVIDIA lose or these workloads to be the primary RFP criteria in the industry before others will join. Getting OEMs to demonstrate they provide little to no value or differentiation was a good step to get more involved, but for a Dell EMC customer, this effectively proved that it is cheaper to just get lower margin Inspur-NVIDIA solutions for AI (see chart above.) That may be a marketing win for NVIDIA, but at some point, someone at Dell EMC marketing will realize that helping NVIDIA show more participation with this effort did them no favors. Hopefully, that realization includes bringing solutions such as Graphcore from Dell’s portfolio or Intel Habana Labs solutions to the mix. We also hope to see the edge cases show more IoT/ edge rather than small 5G radio data center edge locations.
The process is long, but at least there is a concerted effort out there to provide something useful to the market.
MLPerf should be going after those IoT processors and devices not data center stuff. NVIDIA will change this once the Arm acquisition goes through. I’m shocked there’s so much DC stuff.