A few weeks ago we covered the latest MLPerf Training v1.0 results. After the results were released we published our Inspur NF5488A5 review, which was one of the systems featured in the latest round of results. We got a number of questions from our readers around the review and MLPerf results and reached out to Inspur to get some insights that we can share. Specifically, I was able to chat with Gavin Wang, Senior AI Product Manager at Inspur Information to get some answers to the questions we had. Instead of doing this as a verbatim interview, I wanted to summarize the discussion into a few notes for our readers.
Notes from MLPerf Training v1.0 Results Discussion with Inspur
The first questions that we had for Gavin were around the hardware used in the results. Specifically, I saw not just the Inspur NF5488A5, but also a 500W variant as well an Intel Xeon Ice Lake variant dubbed the NF5488M6.
One of the first things I noticed was that results were submitted for an 8x 500W GPU solution. We are going to have more on liquid cooling v. air cooling for these 8x NVIDIA A100’s and 400W v. 500W in the next few days. Some vendors require that 500W 8x NVIDIA A100 SXM4 GPU servers are liquid-cooled. Gavin told me that Inspur can air cool the 500W A100 solution. Just for some frame of reference, the Dell EMC PowerEdge XE8545 can air cool 500W A100’s in 4U, but it requires lower ambient data center temperatures and Dell is only cooling four 500W GPUs in 4U instead of eight. This is a big differentiator for Inspur.
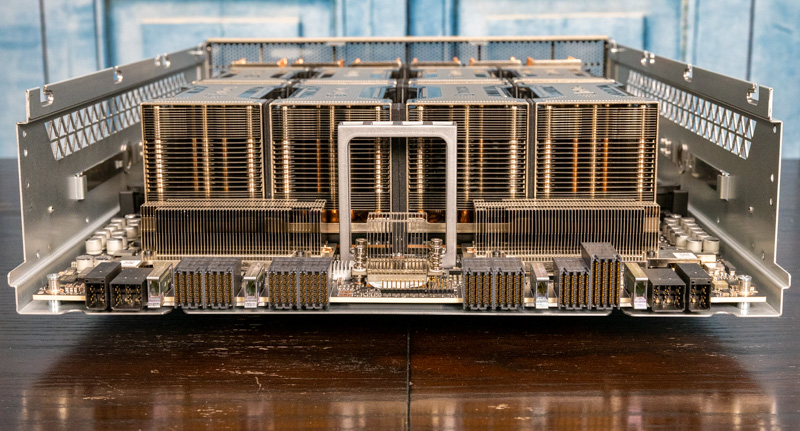
I asked around why customers may choose the Intel v. AMD solution. The answer was a bit surprising. Intel has a single monolithic die in the Ice Lake Xeon series, perhaps the last one we will see in the space which is why we titled our launch piece Intel Xeon Ice Lake Edition Marks the Start and End of an Era. For some workloads, the single die approach provides latency benefits and that is why Intel can be a preferred solution. Other workloads required more pre-processing and often the AMD EPYC-based solution with up to 64 cores / 128 threads per socket has more cores to handle the pre-processing required in different workloads. For example, for language-related workloads, latency may be more important but for video/ image workloads pre-processing often occurs to get images in the correct format to be used by the training solution.
Since this was a big topic in our Graphcore MLPerf Training v1.0 piece, I asked why someone may choose an Inspur 8x GPU system instead of a NVIDIA DGX system. Inspur highlighted its ability to create custom configurations and its ability to deliver systems to hyper-scale size deployments as well as smaller organizations. It also has both AMD and Intel-based solutions mentioned above. Price, especially given configuration options, can also be a factor. Finally, Inspur has solutions like Inspur AIStation for AI cluster operations management and a support organization to ensure that customers can maintain and operate systems. Often these systems are part of larger solutions Inspur builds for its customers where it is providing storage and CPU/ FPGA compute servers as well whereas NVIDIA focuses on the training server market.
I asked about why we are still seeing mostly NVIDIA results in MLPerf Training. I am not going to quote this exactly, but Inspur told me it works with a number of different AI vendors. MLPerf Training requires an extreme amount of effort to tune just to get a publishable result. NVIDIA spends a lot of time working with its partners to do this tuning. Aside from being the leader in the market, NVIDIA also offers good support for its partners working on MLPerf Training. It can do so because it is such a large vendor in the space. Smaller or other vendors are putting resources towards tuning customer performance instead of MLPerf. We may see other results in the future but my impression here is that NVIDIA is out-investing its competition in showing MLPerf Training results to the point that we are not seeing other vendors do the same.
Going Forward Trends in AI Training
I asked a few questions about what the future holds for AI training clusters as well. Today’s NVIDIA A100 GPUs are already starting to push practical limits, so the question is what is next for the market.
One of the biggest trends is clearly the need for more cooling as well as power delivery. Many organizations are looking at liquid cooling, but even delivering enough power to systems can be a challenge. Gavin seemed to think that next-generation systems would have to focus more on how to get power all the way to the higher-power accelerators as well as how systems can be cooled.
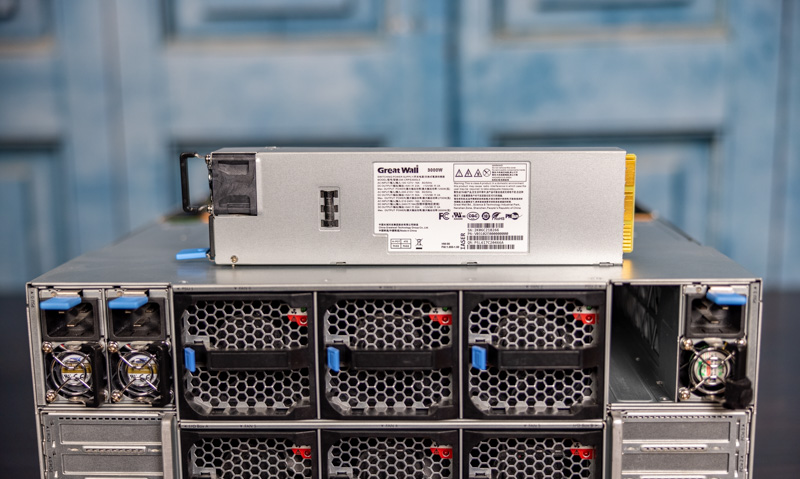
He also noted that it is not just the GPUs/ AI accelerators that next-generation installations will look to liquid cool. It is also the next-generation server CPUs that will generate more heat and use more power than the current generation. This is a trend that has been happening for some time and will continue.
On the power and cooling side, Gavin also mentioned that customers are not just looking at this at a system level. Instead, the next-generation systems will use enough power that operators are looking at the PUE (power usage effectiveness) of their data center facilities to ensure that operations are efficient. It seems like more effort will be going into looking at the total system/ cluster impact rather than just the machine.
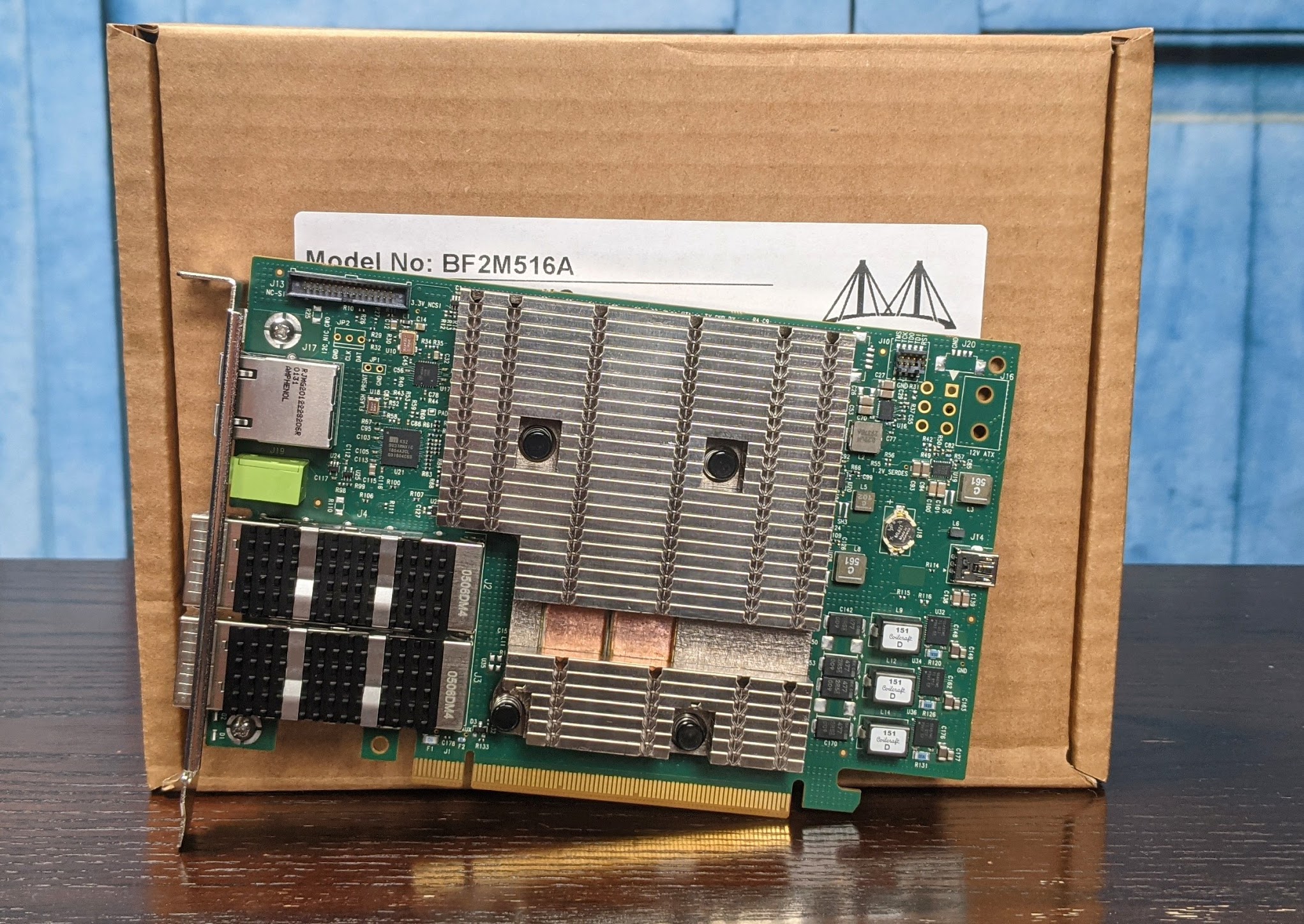
I asked about DPUs as well. For those who missed our coverage, we have What is a DPU? A Data Processing Unit Quick Primer as this is becoming a major trend.
Since the industry is still working to standardize on terminology, we have our DPU vs SmartNIC and the STH NIC Continuum Framework as a reference guide.
Inspur said that NVIDIA is pushing the DPU initiative. It seems as though Inspur is already working with customers on DPU initiatives. One of the big reasons it seems like customers are looking at DPUs is for cluster security. Speaking with Inspur, it seems like DPUs are set to be a bigger part of next-generation AI/ HPC clusters than they are today.
Final Words
I just wanted to say thank you to Gavin and Inspur for taking a few minutes to answer questions. When it comes to getting context around the market, it helps to hear from participants. Inspur put up some excellent numbers in the latest MLPerf Training v1.0 results taking the top single-node spot in about half the tests.
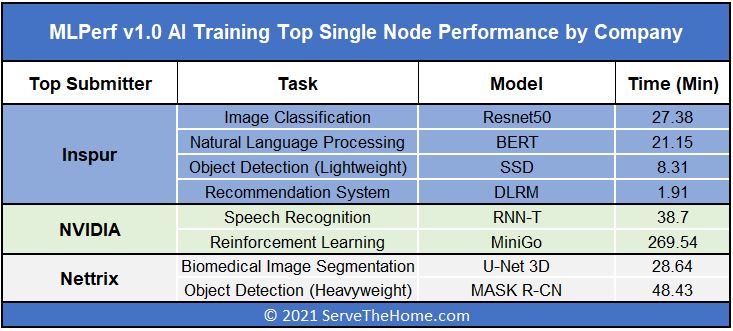
Hopefully, this helps answer many of the questions our readers asked us. We did not get to everything, but these were the big items we heard consistent questions on.
“We may see other results in the future but my impression here is that NVIDIA is out-investing its competition in showing MLPerf Training results to the point that we are not seeing other vendors do the same.”
I think you just got the PR line. Inspur is representing these AI chips as well, after all. There is one MLPerf and it’s visible to all potential customers and investors. I can’t have the wool pulled over my eyes to believe that startups out of stealth mode aren’t almost always desperately looking to be noticed and hyped. There wouldn’t need to be results from multiple vendors, inspur, Dell, etc, as Nvidia does. There would just need to be a result for each test from a vendor. There is no way under creation that an AI chip company that could beat NVIDIA in the mlperf results would not prioritize that effort to kick a hole in the sky and turn their name to gold. Not hiring better PR people who won’t just try to sell the same nonsense for years, however, I can understand.
MLPerf just released the v1.0 results for some of the fastest SuperComputers:
https://mlcommons.org/en/news/mlperf-hpc-v1/
https://mlcommons.org/en/training-hpc-10/