Inspur NF5468M5 GPU Performance
Frameworks change rapidly in the deep learning space, as do software optimizations. As a result, we are looking for two main factors in our GPU performance figures. First, can the system perform well with the NVIDIA Tesla V100 GPUs. Second, can the system keep the GPUs properly cooled to maintain performance over time.
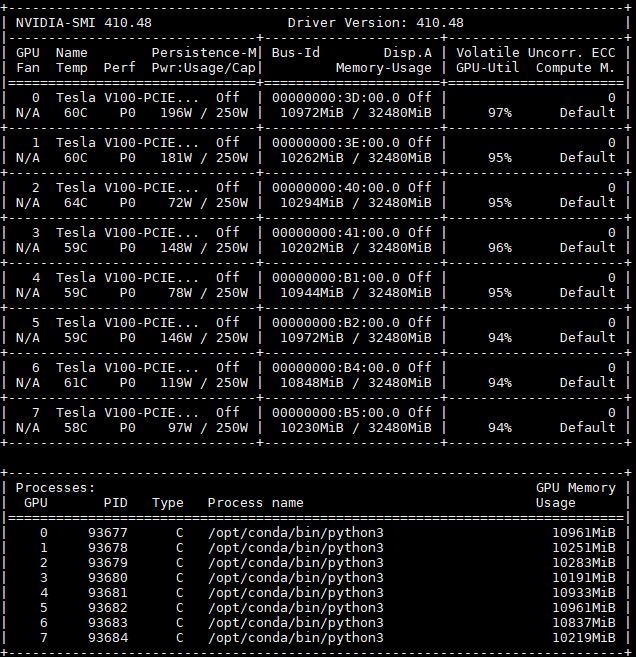
Inspur NF5468M5 GPU-to-GPU Performance
With our system, we have the ability to do peer-to-peer GPU-to-GPU transfers over PCIe. For our testing, we are using 8x NVIDIA Tesla V100 32GB PCIe modules.
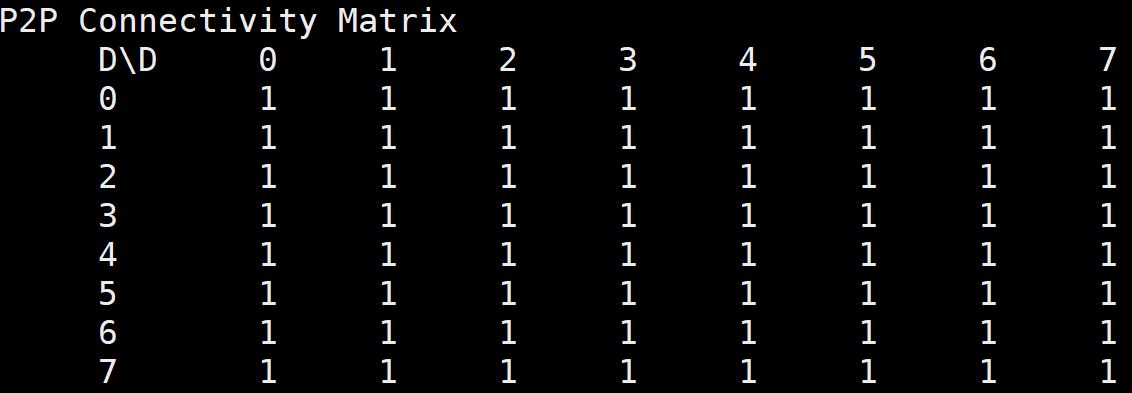
First off, we wanted to show that our 8x NVIDIA Tesla V100 32GB PCIe in the Inspur Systems NF5468M5 This is important since we saw with the system topology that the GPUs are attached to different CPUs.
NVIDIA Tesla V100 PCIe P2P Testing
We wanted to take a look at what the peer-to-peer bandwidth looks like. For comparison, we have DeepLearning10, a dual root Xeon E5 server, and DeepLearning11 a single root Xeon E5 server, and DeepLearning12 a Tesla P100 SXM2 server.
Inspur NF5468M5 P2P Bandwidth
Here is the Unidirectional P2P bandwidth on the dual root PCIe server:
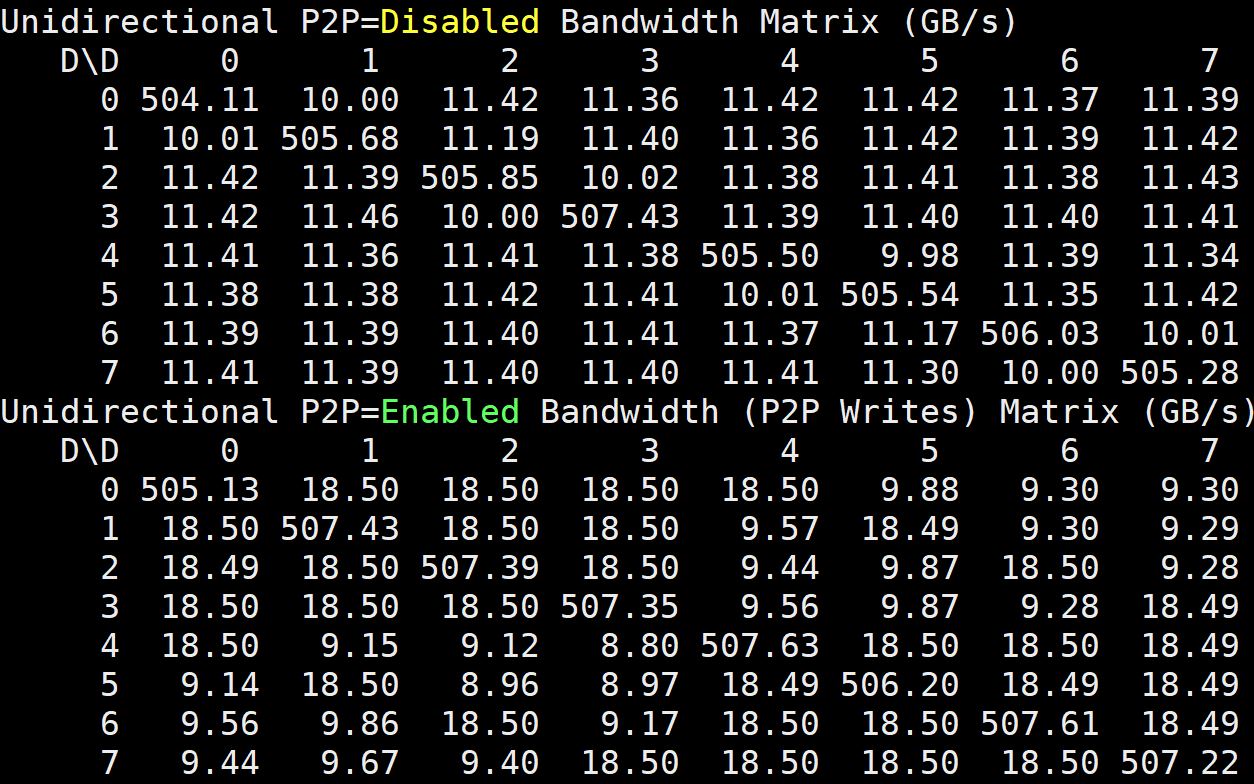
Here is the Inspur Systems NF5468M5 bidirectional bandwidth matrix with P2P disabled and enabled.
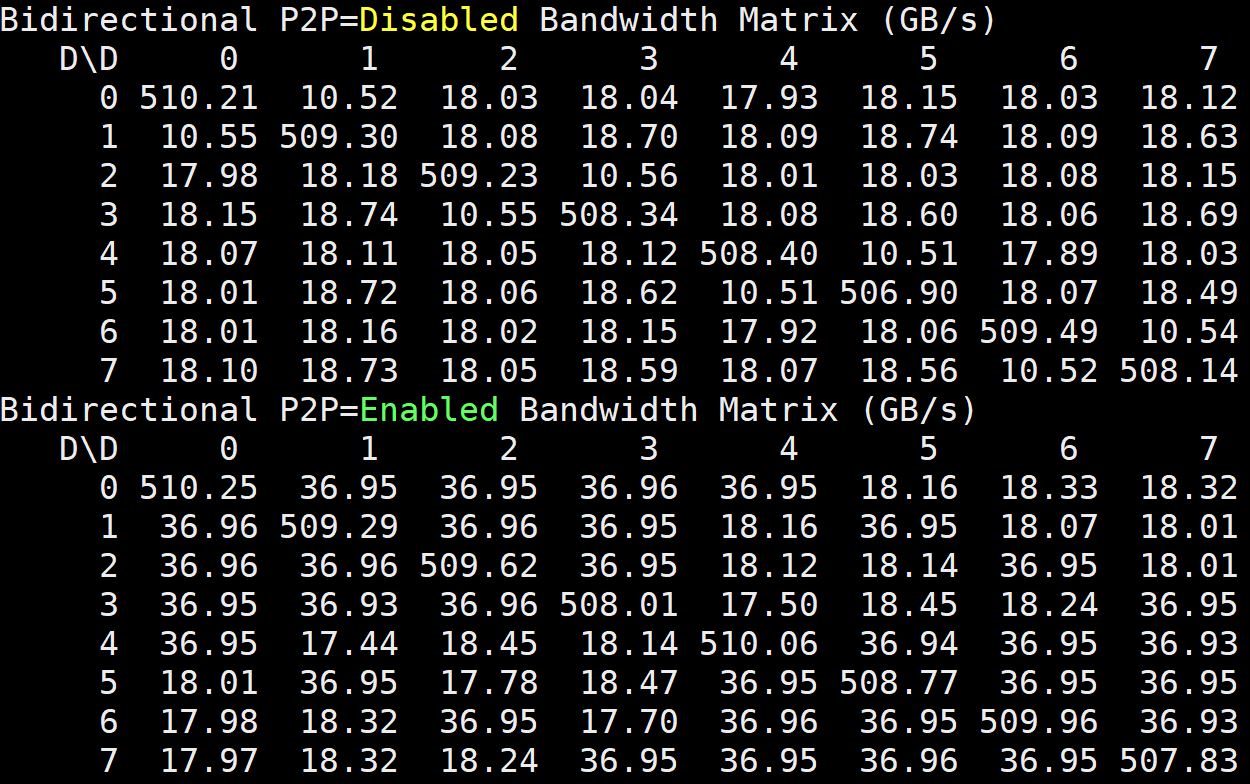
You can clearly see the difference when traversing the UPI link between CPUs. Bandwidth significantly increases. The key takeaway here is how the topology impacts the general bandwidth between GPUs and across the two PCIe switch complexes.
Inspur NF5468M5 Latency
Beyond raw bandwidth, we wanted to show Inspur Systems NF5468M5 GPU-to-GPU latency. Again, see links above for comparison points:
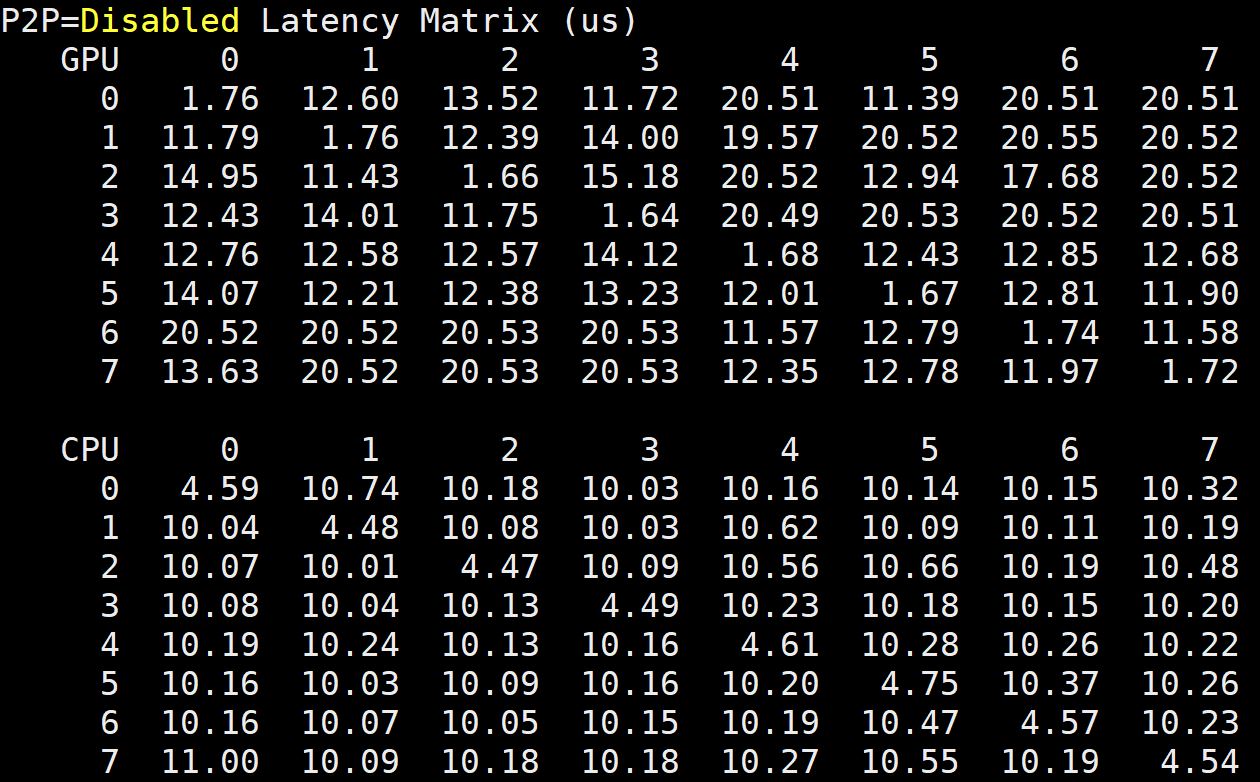
Comparing these to the dual root server’s P2P results, you can see a huge latency jump.
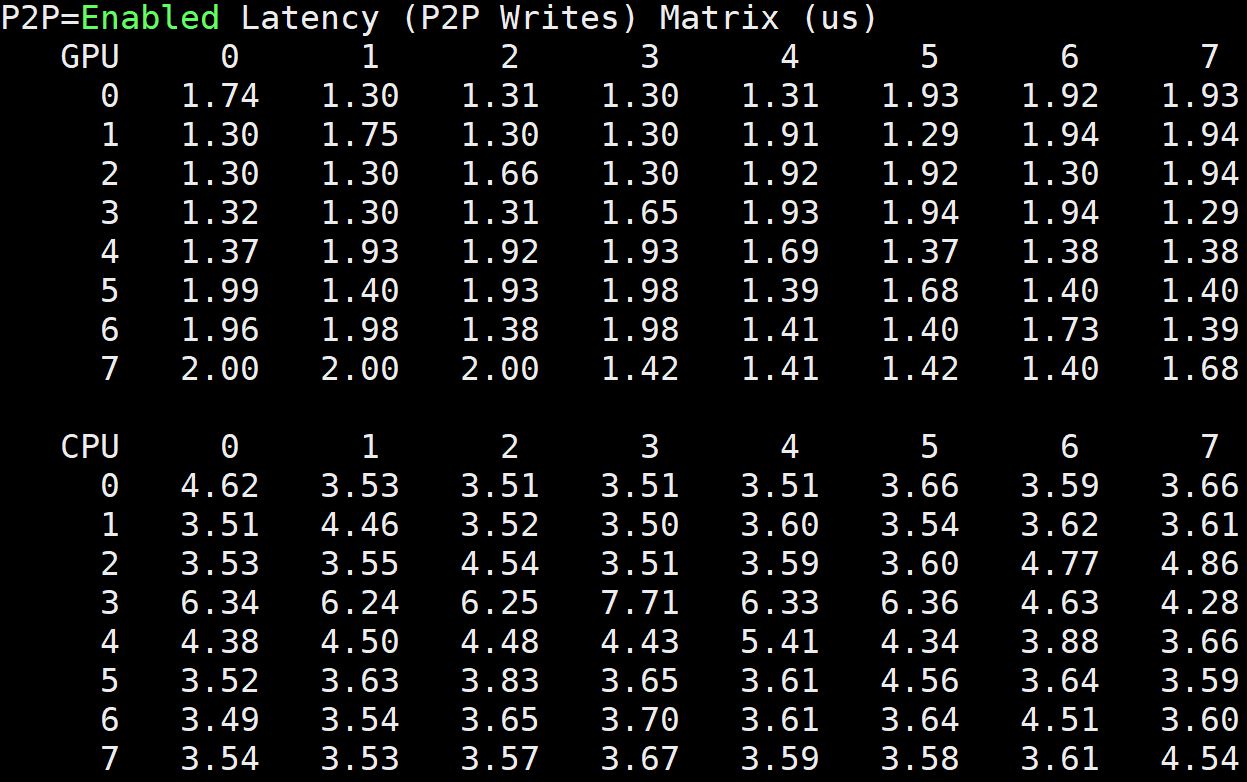
This is a fairly excellent result and is better than what we saw on our Supermicro Intel Xeon E5 V4-based dual root server. Inspur’s solution with Intel Xeon Scalable provides significant benefit.
The key here is that the solution is vastly improved over the PCIe solutions, and that is a major selling point over single root PCIe systems. Looking at this, you can clearly see why NVLink users tout GPU-to-GPU latency benefits.
Inspur NF5468M5 TensorFlow Resnet-50 GPU Scaling
We wanted to give some sense of performance using one of the TensorFlow workloads that we utilized. Here, we are increasing the number of GPUs used while training Resnet50 on Imagenet data.
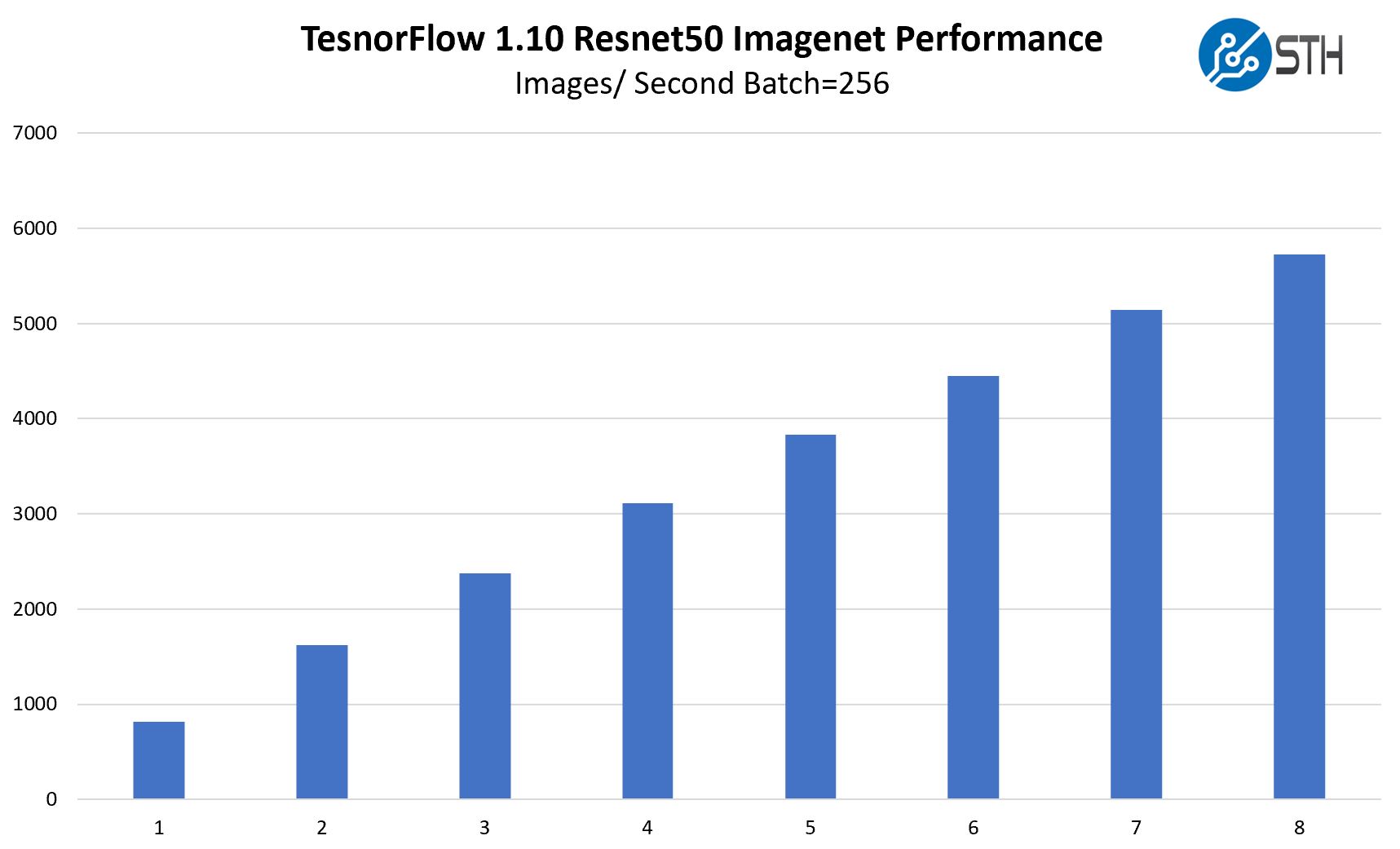
One can see that there is some impact moving beyond four GPUs. For those consistently training on 8x or even 16x GPUs, Inspur has the AGX-2 and AGX-5 NVLink solutions. We showed Inspur’s 16x GPU solution in our piece Inspur AGX-5 and Our SC18 Discussion with the Company. PCIe solutions are popular, but the company also has NVLink and NVSwitch solutions for greater GPU-to-GPU performance.
Inspur NF5468M5 with 8x NVIDIA Tesla V100 32GB GPU Linpack Performance
One of the other advantages of a solution like this is the double-precision compute performance. While many in the deep learning community are focusing on lower precision, there are HPC applications, and indeed many deep learning applications that still want the extra precision that dual precision offers. Linpack is still what people use for talking HPC application performance. NVIDIA’s desktop GPUs like the GTX and RTX series have atrocious double precision performance as part of market de-featuring. We are instead using some HPC CPUs for comparison from Intel, AMD, and Cavium.
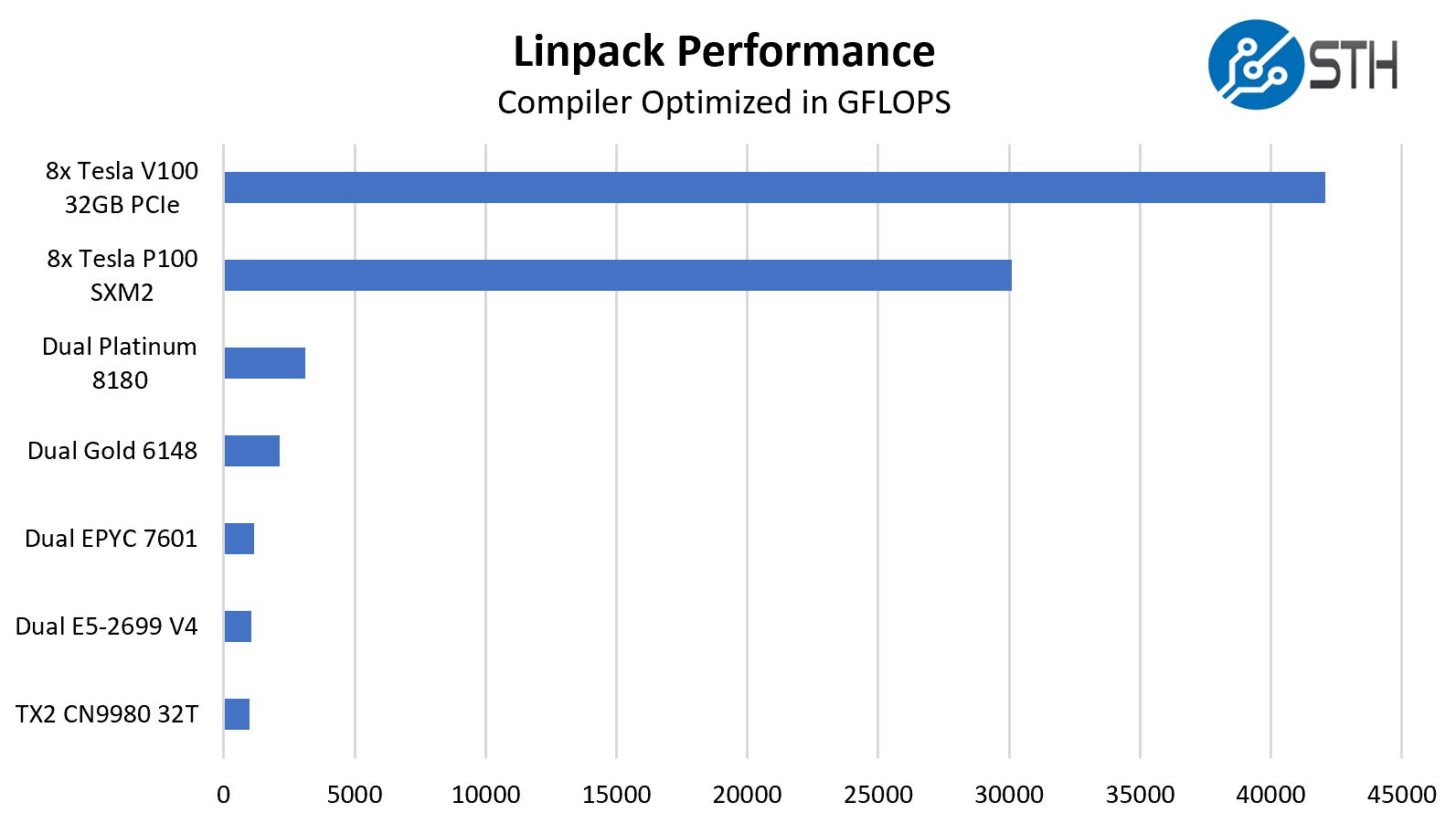
There are teams that are getting higher HPL performance than we are with more optimized setups, however the increase in performance both from CPU to GPU and the NVIDIA Tesla P100 to V100 generations are clearly on display.
OTOY OctaneBench 4.00
We often get asked for rendering benchmarks in our GPU reviews, so we added OctaneBench to the test suite recently.
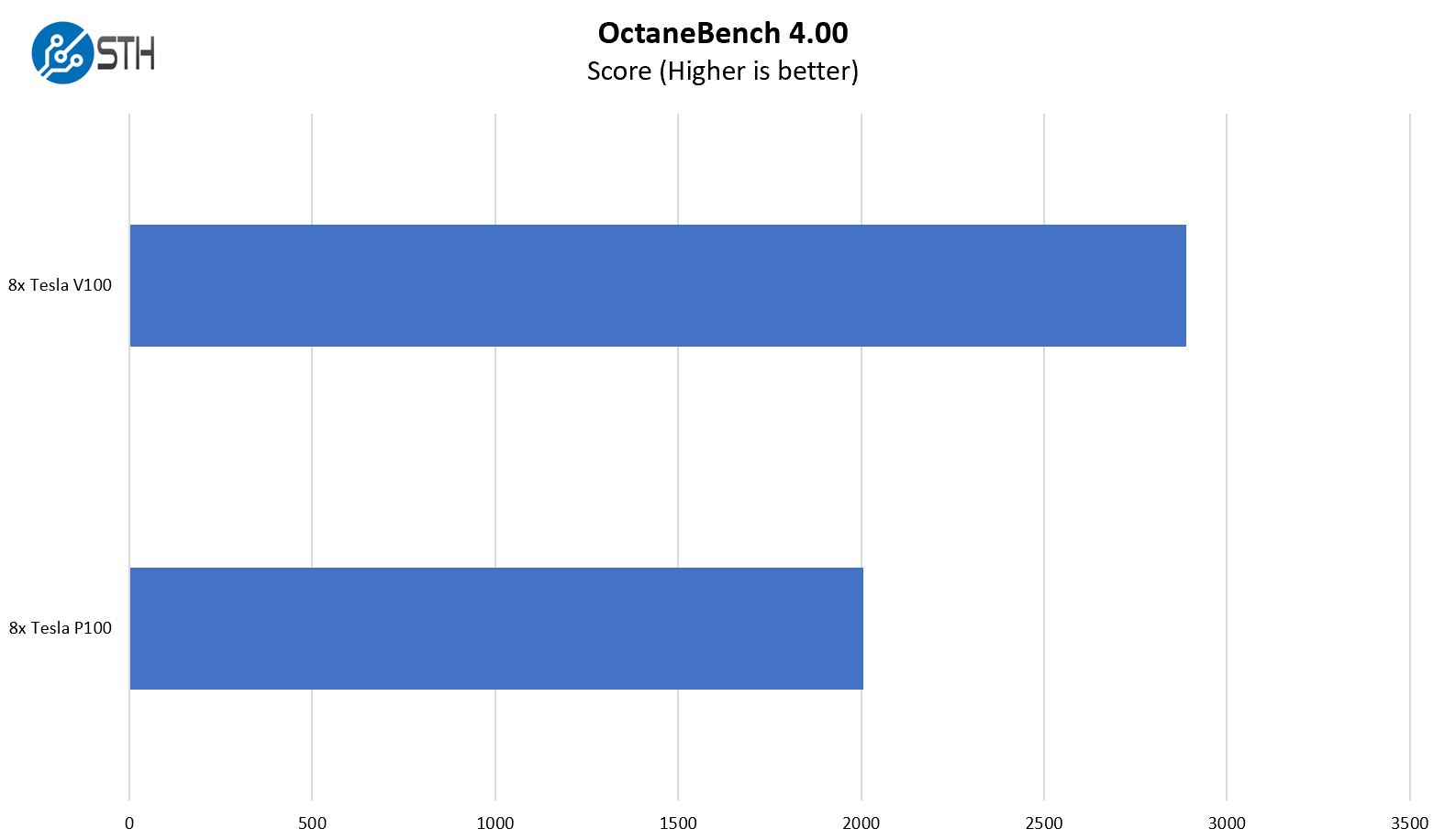
The newer parts show more performance and we will add data points as we get them using the new version of OctaneBench.
Next, we are going to take a look at the Inspur Systems NF5468M5 GPU performance before moving on to storage and networking performance.
Ya’ll are doing some amazing reviews. Let us know when the server is translated on par with Dell.
How wonderful this product review is! So practical and justice!
Amazing. For us to consider Inspur in Europe English translation needs to be perfect since we have people from 11 different first languages in IT. Our corporate standard since we are international is English. Since English isn’t my first language I know why so early of that looks a little off. They need to hire you or someone to do that final read and editing and we would be able to consider them.
The system looks great. Do more of these reviews
Thanks for the review, would love to see a comparison with MI60 in a similar setup.
Great review! This looks like better hardware than the Supermicro GPU servers we use.
Can we see a review of the Asus ESC8000 as well? I have not found any other gpu compute designer that offers the choice in bios between single and dual root such as Asus does.
Hi Matthias – we have two ASUS platforms in the lab that are being reviewed, but not the ASUS ESC8000. I will ask.
How is the performance affected by CVE‑2019‑5665 through CVE‑2019‑5671and CVE‑2018‑6260?
P2P bandwidth testing result is incorrect, above result should be from NVLINK P100 GPU server not PCIE V100.