Building DeepLearning12 in the Gigabyte G481-S80
The Gigabyte G481-S80 is a 4U server, but it consumes a large amount of hardware. We had 2x Intel Xeon Gold 6138 CPUs, 12x 16GB (later 24x 16GB) DDR4-2666 RAM modules, multiple SSDs (NVMe and SATA), 8x NVIDIA Tesla P100 SXM2 GPUs, and ten massive heatsinks. For networking, we used four 100GbE/ EDR Infiniband Mellanox ConnectX4 NICs, a 40GbE ConnectX-3 Pro NIC, and a dual 25GbE OCP mezzanine card.
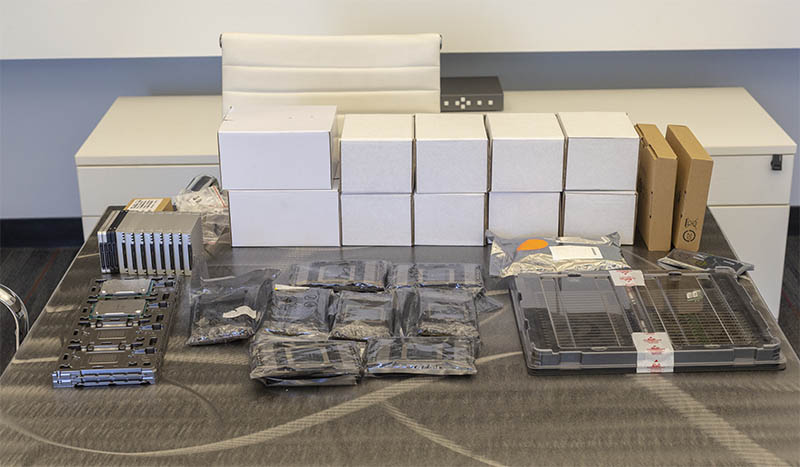
The CPU and RAM area was extremely easy to work on. Once the top cover was off, it felt like almost any other Intel Xeon Scalable CPU and memory installation. There are a total of 12x DDR4 DIMM slots per CPU for 24x total. We used 192GB and 384GB configurations, but that is on the lower end of what you will see in a Gigabyte G481-S80.
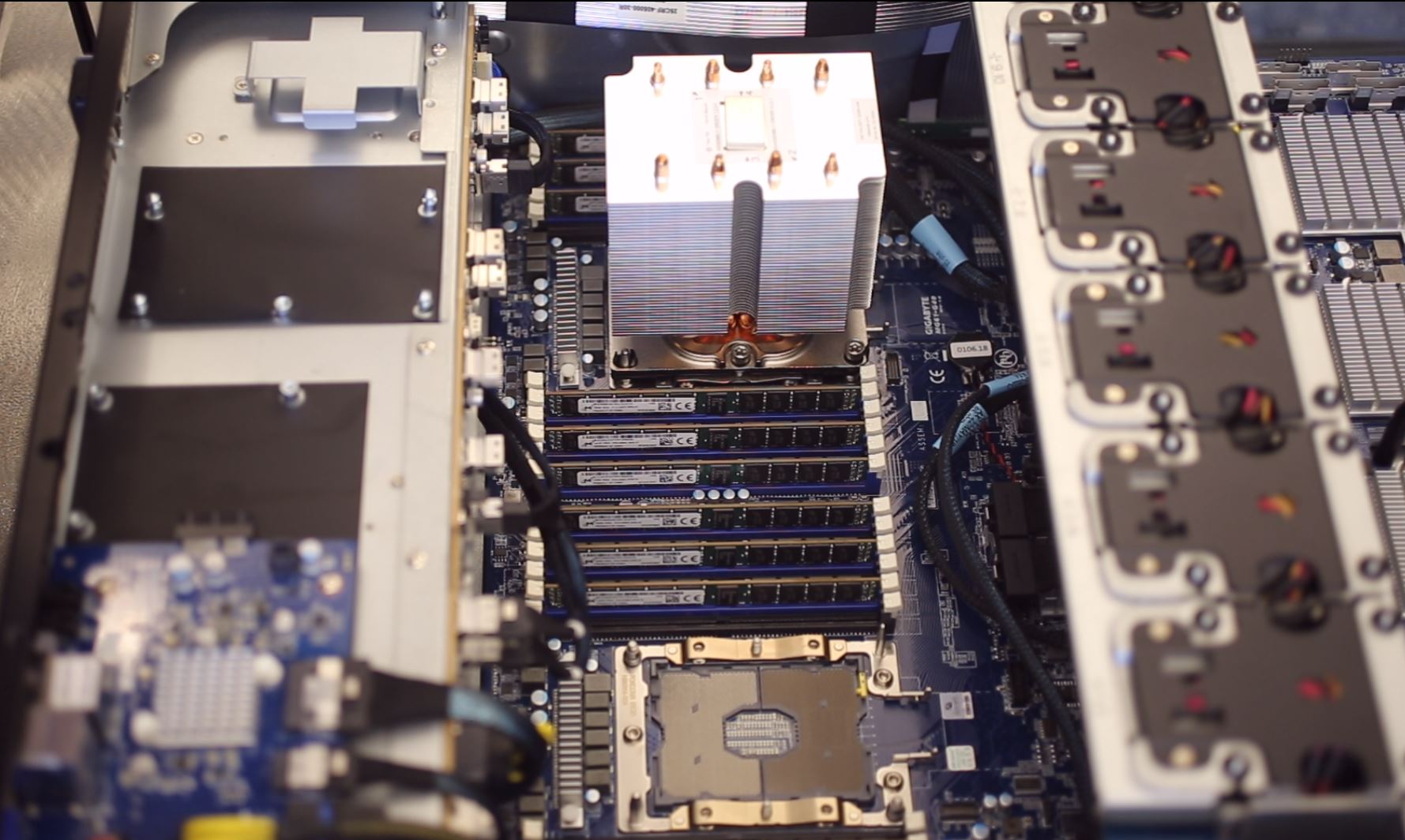
The main area of the Gigabyte G481-S80 is where the NVIDIA Tesla SXM2 modules go, but there is more. There are slots for four 100Gbps networking cards, one for every two GPUs that sit on the same PCIe root for GPUDirect RDMA. Here, just use Mellanox ConnectX-4 or ConnectX-5 and do not bother with any other configuration.
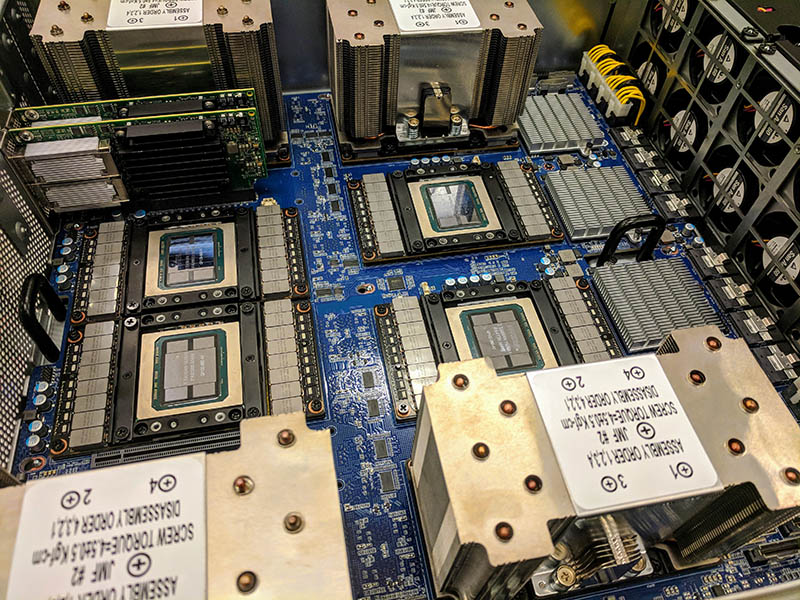
We have an installation video that you can see here. We installed the SXM2 modules ourselves. After having done it, we highly recommend having these installed at the factory. We spoke to NVIDIA about it and several vendors and the general consensus is that this is a difficult installation with a high-risk for damaging expensive GPUs. This is an area where PCIe installation is much easier.
Once the GPUs. are installed, one is left with what we call a SXM2 “heatsink forest.” Front and rear GPUs have different size heatsinks but the array is absolutely menacing. Then again, these heat pipe coolers need to dissipate heat from 300W TDP NVIDIA Tesla SXM2 modules. The DGX-1 class SXM2 modules are up to 300W. The DGX-2 GPUs can hit 350W we are told. That is certainly a difference between the two. Compared to a PCIe cooling solution, you can see how this is much more efficient. You can also see why Gigabyte put water cooling hose holes in the rear of the chassis as these are systems that one can easily see getting water cooling treatment.
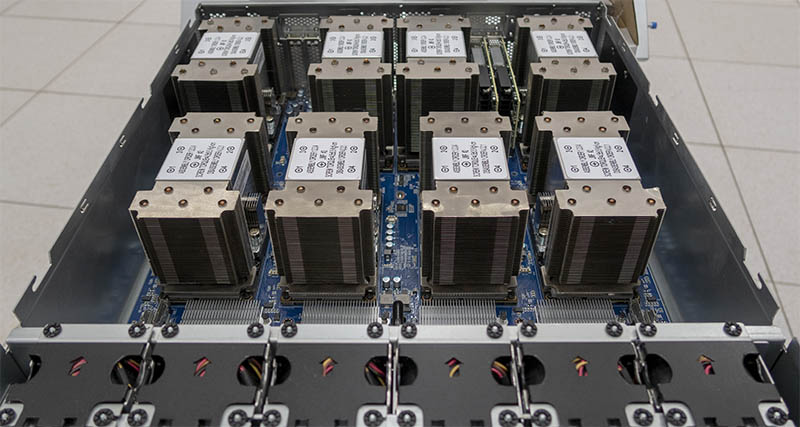
For some perspective, the area depicted is pre-heated by the two Intel Xeon Scalable CPUs, RAM, and storage. It then has to handle cooling around 2500W worth of components including the 8x SXM2 GPUs, PCIe switches, and 100Gbps networking.
We did not get a great photo of this, but in the rear of the chassis, there is a curious pull-out option next to the power supplies. Inside this pop-out area is a Gigabyte CLBGM10 module. It is a fairly large PCB that ultimately allows for the addition of an OCP networking module. In this case, we have a dual 25GbE Broadcom OCP mezzanine card installed.
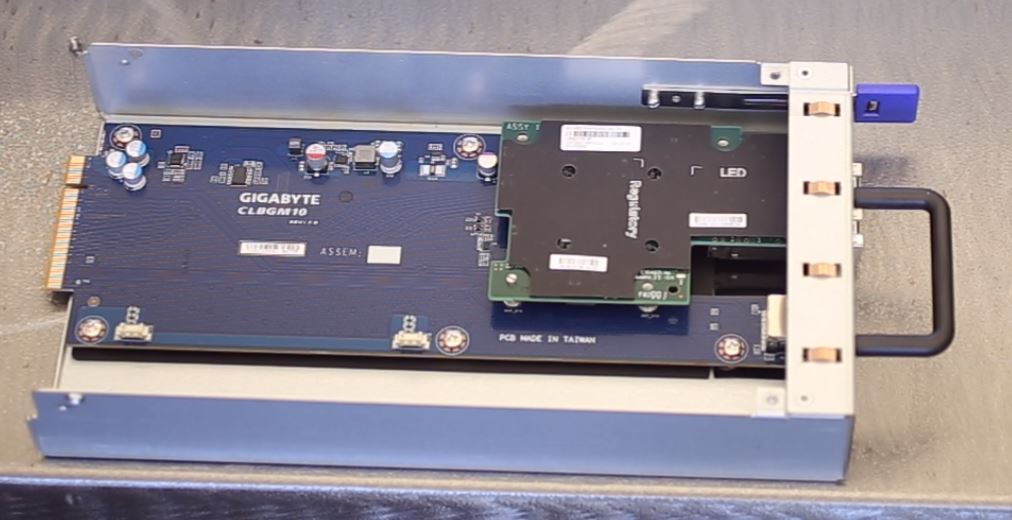
We have a video talking about the hardware in detail that is worth checking out.
This is, by far, the most difficult server I have ever built. 2U 4-node servers, blade servers, and even 8x and 10x GPU PCIe servers are much easier. The design of the Gigabyte G481-S80 facilitated installation, it simply took a long time. We strongly recommend having someone else build your SXM2 GPU server.
In terms of racking and stacking, my current deadlift is around 400lbs/ 181kg and I was able to rack the system hip height without too much trouble. In the lab, we keep lower U’s for large and heavy chassis such as this one. Anything higher than hip height and I would have had to use our server lift. The rail system for the G418-S80 is “L” shaped shelves which makes installation very easy. These boxes can weigh 100-200lbs. If you want to deploy racks of these, you may also want to ensure that you have the power, cooling, and floor support capacity to handle such a deployment.
Next, we are going to look at the Gigabyte G481-S80 topology. In the deep learning/ AI space, system topology is a big deal. There is a reason you want this type of architecture over a PCIe architecture, and we will show why.
Gromacs would be a nice benchmark to see.
Thanks for doing more than AI benches. I’ve sent you an e-mail through the contact form on a training set we use a Supermicro 8 GPU 1080 Ti system for. I’d like to see comparison data from this Gigabyte system.
Another thorough review
It’s too bad that NVIDIA doesn’t have a $2-3K GPU for these systems. Those P100’s you use start in the $5-6K each GPU range and the V100’s are $9-10K each.
At $6K per GPU that’s $48K per GPU, or two single root PCIe systems. Add another $6K for Xeon Gold, $6K for the Mellanox cards, $5K for RAM, $5K for storage and you’re at $70K as a realistic starting price.
Regarding the power supplies, when you said 4x 2200W redundant, it means that you can have two out of the four power supplies to fail right?
I’m asking this because I’m might be running out of C20 power socket in my rack and I want to know if I can plug only two power supplies.
Sorry, my question’s reply is in the marketing video.
They are 2+N power supplies.
Interested if anyone has attempted a build using 2080Tis? Or if anyone at STH would be interested. The 2080 Ti appears to show much greater promise in deep-learning than it’s predecessor (1080Ti), and some sources seem to state the Turing architecture is able to perform better with FP16 without using a single many tensorcores as former Volta architecture. Training tests on tensorflow done by server company lambda also show great promise for the 2080Ti.
Since 2080Ti support 2-way 100Gb/sec bidirectional NVlink, I’m curious if there are any 4x, 8x (or more?) 2080ti builds that could be done by linking each pair of cards with nvlink, and using some sort of mellanox gpu direct connectX device to link the pairs. Mellanox’s new connectX-5 and -6 are incredibly fast as well. If a system like that is possible, I feel it’d be a real challenger in terms of both compute-speed and bandwidth to the enterprise-class V100 systems currently available.
Cooling is a problem on the 2080 Ti’s. We have some in the lab but the old 1080 Ti Founder’s edition cards were excellent in dense designs.
The other big one out there is that you can get 1080 Ti FE cards for half the price of 2080 Ti’s which in the larger 8x and 10x GPU systems means you are getting 3 systems for the price of two.
It is something we are working on, but not 100% ready to recommend that setup yet. NVIDIA is biasing features for the Tesla cards versus GTX/ RTX.