In a series we have been doing for some time now, I wanted to take a quick moment and check in on the state of AI with Inspur and NVIDIA. This year instead of just covering the MLPerf Training v1.1 results, I wanted to take the opportunity to cover the release in a bit of a different format. I sent a few questions to Inspur and NVIDIA to get their take on the latest results and some of the trends they are seeing. Due to the pandemic, I was not able to get to visit Inspur this year, so it is always good to get insight from one of the largest server vendors in the world.
For this installment, we have Dr. Shawn Wu of Inspur along with Paresh Kharya of NVIDIA. Paresh, STH readers may remember, we also talked to last year in our AI in 2020 and a Glimpse of 2021 with Inspur and NVIDIA piece. As a fun one, normally in interviews, we use the initials of folks as we go through the interview. Paresh and I share “PK” so we are going to use first names instead. This is a break from format due to a very small percentage chance that this would happen.
Talking MLPerf Training V1.1 with Inspur and NVIDIA
- Patrick Kennedy: Can you tell our readers about yourself?
Shawn: I am Dr. Shawn Wu, Chief Researcher at the Inspur Artificial Intelligence Research Institute. My research is in large-scale distributed computing, AI algorithms, deep learning frameworks, compilers, and etc.
Paresh: I have been a part of NVIDIA for more than 6 years. My current role is senior director of product management and marketing for accelerated computing at NVIDIA. I am responsible for the go-to-market aspects including messaging, positioning, launch, and sales enablement of NVIDIA’s data center products, including server GPUs and software platforms for AI and HPC. Previously, I held a variety of business roles in the high-tech industry, including product management at Adobe and business development at Tech Mahindra.
- Patrick: How did you get involved in the AI hardware industry and MLPerf?
Shawn: I joined Inspur Information upon receiving my Ph.D. from Tsinghua University. Inspur is a leader in server technology and innovation. This led to my research focus in high-performance computing (HPC) and AI. MLPerf has quickly established itself as the industry benchmark for AI performance, making it a natural fit for applying the world-class AI research being done at the Inspur Artificial Intelligence Research Institute. We have been participating in MLPerf since 2019 and have been setting new performance records ever since.
Paresh: Throughout my career, I’ve been fortunate to have had the opportunity to work on several innovative technologies that have transformed the world. My first job was to create a mobile Internet browser just as the combination of 3G and smartphones were about to revolutionize personal computing. After that, I had the opportunity to work on Cloud Computing and web conference applications taking advantage of it at Adobe. And now I’m very excited to be working at NVIDIA just as modern AI and Deep Learning ignited by NVIDIA’s GPU accelerated computing platform are driving the greatest technological breakthroughs of our generation.
MLPerf Training v1.1
- Patrick: MLPerf has been out for some time, what were your big takeaways from MLPerf Training v1.1?
Shawn: It is an honor to be competing among so many outstanding companies at MLPerf, which has been key to the vigorous development of AI benchmarking and resulted in continuous performance improvements. Since Inspur began participating in MLPerf, more and more companies have joined, and with more competition has come more enthusiasm for the benchmark results. The mission of MLPerf is not static, it follows the developments and focus of the industry to continuously update the tested models and scenarios accordingly, and helps communicate industry-wide development trends. Through competitive benchmarks like MLPerf, the ability to optimize different types of models has been improved, and experience gained in how to select components for different models in order to better utilize the performance advantages of Inspur equipment. The actual application of mainstream software frameworks has also had an enlightening effect on our framework selection and optimization process.
Paresh: MLPerf represents real-world usages for AI applications and so customers ask for MLPerf results. This motivates participation from solution providers which continues to be excellent. There were 13 submitters to this round of MLPerf training which had 8 benchmarks representing applications from NLP, speech recognition, recommender systems, reinforcement learning, and computer vision.
NVIDIA AI with NVIDIA A100, and NVIDIA IB Networking Set Records Across All benchmarks delivering up to 20x more performance in 3 years since the benchmarks started and 5x in 1 Year alone with software innovation.
NVIDIA ecosystem partners submitted excellent results on their servers with Inspur achieving the most records for per server performance with NVIDIA A100 GPUs.
Benchmark | Time to Train (min) |
BERT | 19.39 |
DLRM | 1.70 |
Mask R-CNN | 45.67 |
ResNet-50 | 27.57 |
SSD | 7.98 |
RNN-T | 33.38 |
3DUnet | 23.46 |
- Patrick: What is your sense in terms of the percentage that new hardware impacts training performance versus new software and models? NVIDIA often cites how rapidly the performance gains accrue with new software generations.
Shawn: Great hardware will always be the foundation of great performance. But software improvements have a huge impact as well. Smart algorithms can reduce the number of calculations required, and help release the full power of hardware. Likewise, reasonable scheduling can reduce hardware bottlenecks to improve efficiency, granting greater performance.
Paresh: Accelerated computing requires full-stack optimization from GPU architecture to system design to system software to application software and algorithms. Software optimizations are vital in delivering end application performance. Over the last 3 years of MLPerf NVIDIA AI Platform has delivered up to 20x higher performance per GPU with the combination of full-stack optimizations over the 3 architecture generations – Volta, Turing, and NVIDIA Ampere – provided up to 5x higher performance on our NVIDIA Ampere architecture alone with software and higher scalability afforded by software innovation including SHARP and MagnumIO.
- Patrick: The NVIDIA A100 has been in the market for some time now, are we starting to hit the point of maximum performance from a software perspective?
Shawn: From a software perspective, there is still room for improvement. There is still a major gap between actual computing power and the theoretical maximum based on the hardware. We use software to tap into this otherwise unrealized hardware potential, and continue to optimize software to bring us closer to that theoretical maximum. Consequently, algorithm optimization remains a major tool for advancing performance.
Paresh: I think we’ll continue to see improvements. We increased performance in every round of MLPerf that A100 has participated in since MLPerf v0.7. We’ve increased performance over 5x since v0.7 on Ampere architecture GPUs. This is similar to how our Volta GPUs performance increased continuously for its entire lifespan. With over 75% of our engineers dedicated to software we continue to work hard to find additional optimizations.
- Patrick: Inspur had both Intel Xeon and AMD EPYC servers in this round of MLPerf, are there types of customers or workloads/ models that favor one architecture over the other? If so, what are they?
Shawn: In Training v1.1, Inspur submitted two models, NF5488A5 using AMD CPUs and NF5688M6 using Intel CPUs. NF5488A5 was top-ranked in SSD and ResNet50 tasks and NF5688M6 was top-ranked in DLRM, Mask R-CNN, and BERT tasks.
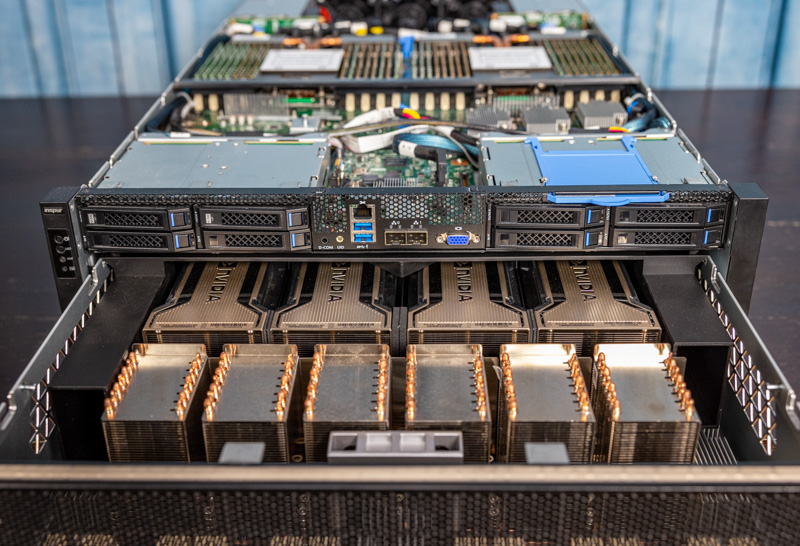
- Patrick: What trends have you seen over the past year regarding the uptake of the NVIDIA A100 SXM versus the traditional PCIe version?
Shawn: In Training v1.0, there were 5 models using PCIe and 11 using NVIDIA A100 SXM. In Training v1.1, there were 4 models using PCIe and 12 using NVIDIA A100 SXM. So we can see that there was a slight uptick in NVIDIA A100 SXM usage, but it was already the overwhelmingly choice over traditional PCIe.
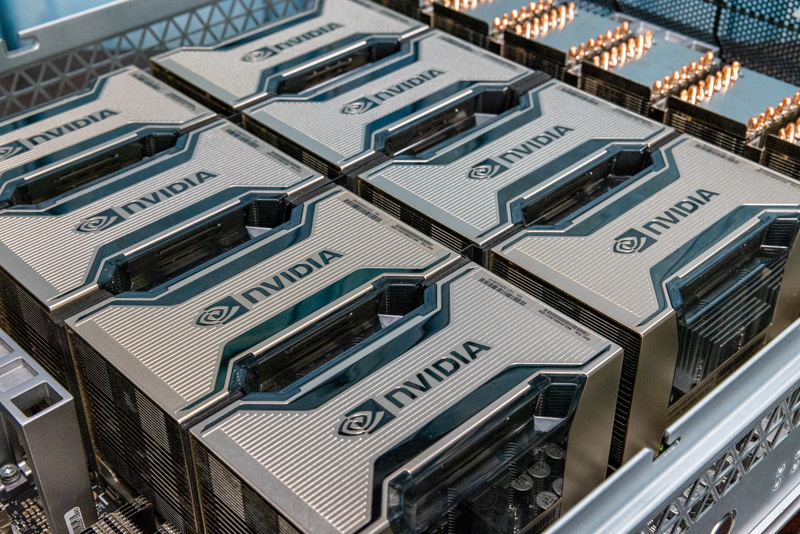
Paresh: Our A100 GPU in SXM form factor is designed for the highest performing servers and provides 4 or 8 A100 GPUs interconnected with 600 GBps NVLink Interconnect. Customers looking for training AI and highest application performance choose A100 GPUs in SXM form factor. A100 is also available in the PCIe form factor for customers looking to add acceleration to their mainstream enterprise servers.
- Patrick: What are the big trends you see in training servers in 2022?
Shawn: A big trend will be the overall optimization from the server to the cluster system. For bottleneck IO transmission, high-load multi-GPU collaborative task scheduling, and heat dissipation issues, the coordination and optimization of software and hardware can reduce communication latency to ensure stable operation and improve AI training performance.
A greater variety of AI chips will be used in training tasks; models will be equipped with more powerful GPUs; 8 or more accelerators in a single node will become mainstream. There will also be more and more large-scale models, and cluster training will receive further attention and development.
Paresh: Training AI is going mainstream in enterprises powered by broadly applicable use cases like NLP and conversational AI. This is changing the application workload mix of enterprise data centers.
AI training requires data center scale optimizations and clusters are being designed with faster networking and storage for scalability. Scale is important for training larger models that provide higher accuracy as well as improving the productivity of data scientists and application developers by enabling them to iterate faster.
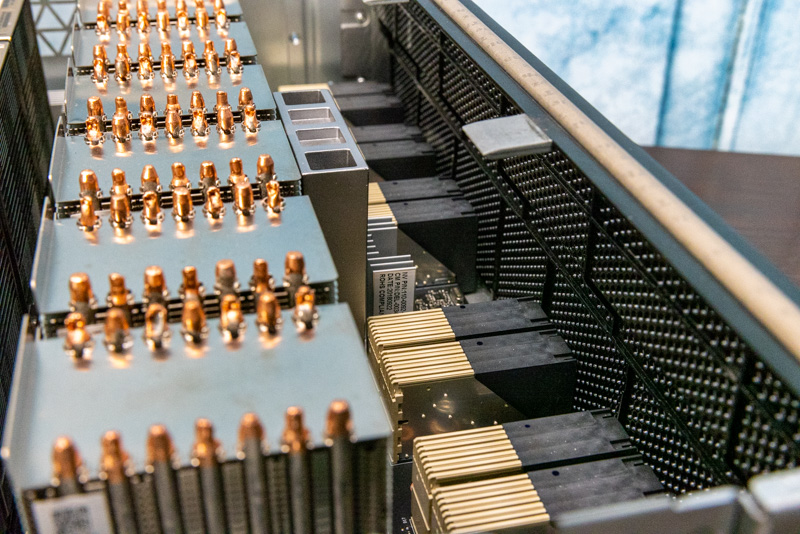
- Patrick: For those looking to deploy training clusters in 2022, is there one network fabric that you think will be dominant? Why?
Shawn: CLOS architecture is recommended for the network fabric to ensure non-blocking communication throughout the network. The most commonly used today is spine-leaf architecture, which allows the network to expand horizontally on the premise of non-blocking communication.
Infiniband is recommended for the network type, Infiniband or RoCE. These two networks support RDMA. We feel that using Infiniband in AI and HPC scenarios, especially in large-scale clusters, has advantages in latency and stability.
Network bandwidth will continue to improve. For large-scale models such as GPT3 and Inspur Yuan 1.0, it will involve data parallelism, model parallelism, and pipeline parallelism in 3-dimensional parallelism. Among these, model parallelism has the largest amount of communication and will have the greatest impact on future cluster development. The training of large models is now 8-way model parallelism, plus multiple pipelines in parallel. We believe that when node bandwidth becomes larger, it will no longer be limited by the 8-way model parallelism.
Paresh: The NVIDIA Quantum InfiniBand fabric will undoubtedly be the dominant networking solution for training clusters for several reasons. Its end-to-end bandwidth, ultra-low latency, GPUDirect, and in-network compute capabilities are exceptionally important when training models; especially as the complexity of models increases. Models today are growing into hundreds of billions to even trillions of parameters. InfiniBand’s In-Network Computing capabilities are especially important for deep learning; offloading collective operations from the CPU to the network and eliminating the need to send data multiple times between endpoints is a real game-changer in reducing overall training time.
- Patrick: Are there plans for Inspur to show other accelerators in the future aside from NVIDIA cards?
Shawn: NVIDIA is one of our most important partners. Every generation of Inspur AI servers will complete NVIDIA GPU product testing and certification. This fundamental partnership covers 90% of the application scenarios in the market. In regards to AI technology, some specific scenarios have emerged, such as video decoding and encoding accelerated processing, where Inspur has released accelerators for these specific scenarios. These accelerators do not conflict with NVIDIA’s main direction.
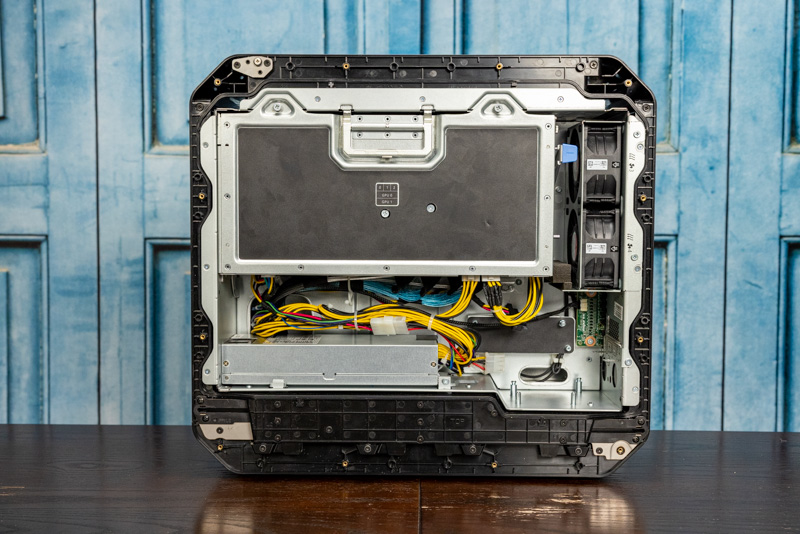
- Patrick: How has storage for training servers evolved in the past year?
Shawn: In training tasks over the past year, storage read speed has had a greater impact on models with large training data in the initial stage. Most manufacturers use the faster read speed NVME drives, and through the formation of a RAID 0 disk array, that data can be read in parallel to further increase read speeds.
Paresh: Training AI has evolved over the last few years from datasets on local storage being sufficient for most use cases to dedicated fast storage subsystems being vital today. Giant models and larger datasets today require fast storage subsystems for overall at scale performance of training clusters. AI training involves large numbers of read operations as well as fast writes once in a while for checkpointing models and experimenting with hyperparameters. For this reason NVIDIA’s Selene supercomputer is architected with the high performance storage system that offers over 2TB/s of peak read and 1.4TB/s of peak write performance. This is complemented by the caching for fast local access to data by the GPUs in a node. In addition to the storage subsystems software tools are needed, for giant language models for instance NVIDIA Megatron which leverages NVIDIA Magnum IO can take advantage of data being fed at more than 1TB/s
Final Words
I just wanted to take a moment to thank both Shawn and Paresh for answering a few of my questions around MLPerf in 2021 and looking into 2022. Hopefully, we can resume doing in-person interviews next year as we get to the next generations of hardware and MLPerf results.