As we close on 2020, I wanted to get some insights into the AI and machine learning market. Instead of giving my view, I wanted to highlight a view of some of the larger players supplying the market with hardware and software. To that end, today we have an interview with Vangel Bojaxhi from Inspur, one of the largest AI systems vendors in the world (and by far the largest in China) along with Paresh Kharya at NVIDIA. As part of this interview, I asked a series of questions to both and simply wanted to present their responses.
This dual interview is organized into three main sections. First, we have some background. We are then going to focus on edge inference as that has been exploding as we move into applying trained models to create value in the field. We are then going to look at the data center market. Finally, we are going to conclude with a look into what 2021 has to offer.
As a quick housekeeping note, I added the images to this interview based on what was being covered. Since we cover the hardware and software being discussed, I wanted to give our readers some visual depiction. If there is an image placed in error, that is my doing.
Background on our Interviewees
Patrick Kennedy: Can you tell our readers about yourself?
Vangel Bojaxhi: I joined Inspur about four years ago, and currently holding the position of Global AI & HPC Director. Prior to that, I served in the capacity of Principal IT Solution Architect at Schlumberger for over twenty years. I focused on the design of the large HPCs and high-end systems for seismic data processing, reservoir modeling/simulation, and interpretation in the Oil and Gas industry. In addition to my role with Inspur Co., I am helping the Asia Supercomputer Community (ASC) as its Executive Director.
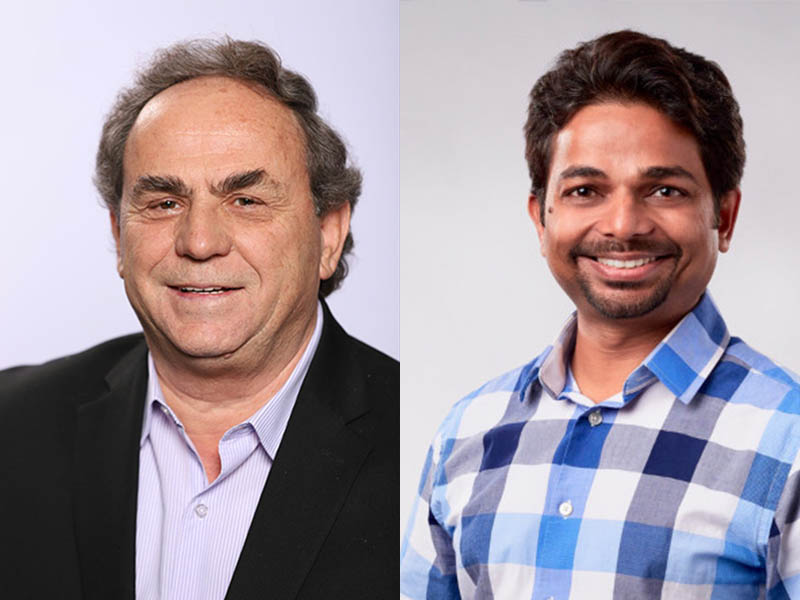
Paresh Kharya: I have been a part of NVIDIA for more than 5 years. My current role is senior director of product management and marketing for accelerated computing at NVIDIA. I am responsible for the go to market aspects including messaging, positioning, launch and sales enablement of NVIDIA’s data center products, including server GPUs and software platforms for AI and HPC.
Previously, I held a variety of business roles in the high-tech industry, including product management at Adobe and business development at Tech Mahindra. I have an MBA from the Indian Institute of Management and a BS in computer science from the National Institute of Technology, India.
Patrick: How did you get involved in the AI hardware industry?
Vangel Pojaxhi: Given my background in Computer Science, I learned about Artificial Intelligence’s initial definitions and theories many years ago. My first practical contact with AI was in using Neural Networks for Seismic HPC applications by correlating rock properties, measured from well logs, with the related geophysical attributes of the seismic signal, to make projections for optimal processing of the entire surrounding geophysical volume. In continuation of my HPC experience, I later joined Inspur HPC division which had AI in its chart. It eventually was renamed AI&HPC department to better reflect AI as a primary activity.
Paresh Kharya: Throughout my career, I’ve been fortunate to have had the opportunity to work on several innovative technologies that have transformed the world.
My first engineering job was to create a mobile Internet browser just as the combination of 3G and smartphones were about to revolutionize personal computing.
After that, I had the opportunity to work on Cloud Computing and applications taking advantage of it. We created products for web conferencing and any-device enterprise collaboration that were delivered with the then-new software as a service.
And now I’m very excited to be working at NVIDIA just as modern AI and Deep Learning ignited by NVIDIA’s GPU accelerated computing are driving the greatest technological breakthroughs of our generation.
Edge Inference
Patrick: What are the biggest trends in edge AI inferencing hardware deployments? Are there certain form factors or features that are becoming common today?
Vangel Pojaxhi: The biggest trends in AI inferencing hardware deployments in edges is the increased complexity, physical size reduction, scattered distribution, and the extra difficulties for management.
Edge AI boxes have become a common form factor for edge AI inference hardware. The EIS1 box developed by Inspur for edge AI is the first to support 5G, is quite small and easy to deploy.
Inspur has also been profoundly involved in the development of Edge AI technologies based on open computing standards like OpenEdge and OTII. One of Inspur’s recent accomplishments for this is the launch the of the edge server NE5260M5 based on the open computing standard OTII (Open Telecom IT Infrastructure) specifications.
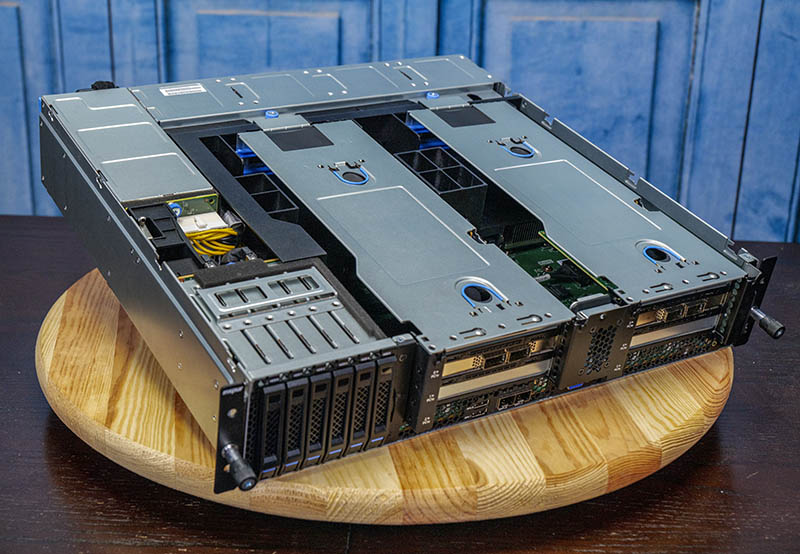
Paresh Kharya: Artificial intelligence (AI) and cloud-native applications, IoT and its billions of sensors, and 5G networking is now making large-scale AI at the edge possible. A scalable and fully programmable accelerated platform is necessary to drive decisions in real-time and allow every industry—including retail, manufacturing, healthcare, autonomous vehicles, and smart cities—to deliver intelligent automation to the point of action.
Edge AI computing is broad and spans from small devices to powerful edge servers. NVIDIA addresses the full spectrum. Starting with 5W Jetson nano for devices bringing the power of NVIDIA AI platform for use cases such as drones, delivery vehicles to industrial robots. Powerful edge servers powered by the efficient 70W NVIDIA T4 or powerful NVIDIA A100 PCIe with 3rd generation of Tensor Cores. And converged accelerators that combine powerful computing performance of NVIDIA GPUs with security and networking capabilities of NVIDIA BlueField DPUs.
NVIDIA EGX stack delivers a cloud-native software platform for deploying GPU-accelerated AI at the edge and broadly in the enterprise data centers optimized for NVIDIA certified servers.
- The biggest trends in AI inferencing hardware deployments in edges is the increased complexity, physical size reduction, scattered distribution, and the extra difficulties for management.
- Edge AI boxes have become a common form factor for edge AI inference hardware. The EIS1 box developed by Inspur for edge AI is the first to support 5G, is quite small and easy to deploy.
- Inspur has also been profoundly involved in the development of Edge AI technologies based on open computing standards like OpenEdge and OTII. One of Inspur’s recent accomplishments for this is the launch of the edge server NE5260M5 based on the open computing standard OTII (Open Telecom IT Infrastructure) specifications.
Patrick: What are the most common applications you are seeing customers use edge AI inferencing for in different regions?
Vangel Pojaxhi: Common edge AI applications around the world include autonomous driving, object detection, smart retail, and etc.
Edge AI application scenarios in China are similar, but in addition would mention the automatic sorting of express parcels, and intelligent management of residential properties, which are developing rapidly.
Recently, Inspur released AIStation Version3 with the following enhancements:
- Fully supports NVIDIA Ampere architecture innovative fine-grained, and flexible sharing of multi-instance GPUs (MIG). Administrators can now dynamically allocate slices of GPU computing power according to different scenarios.
- More elastic provisioning capabilities. Developers can dynamically adjust the ratio of CPU versus GPU resources according to the serial/parallel portion of the workflows, and further improve the granularity and efficiency of AI development, training, and inferences.
- Full integration of AIStaiton with OpenStack. Now the enterprise users can quickly and efficiently develop and deliver scale-out AI services in cloud edges. As the result, OpenStack cloud users can take full advantage of the AI station management suite, which remarkably improves the efficiency of large-scale AI application commercialization in Edges.
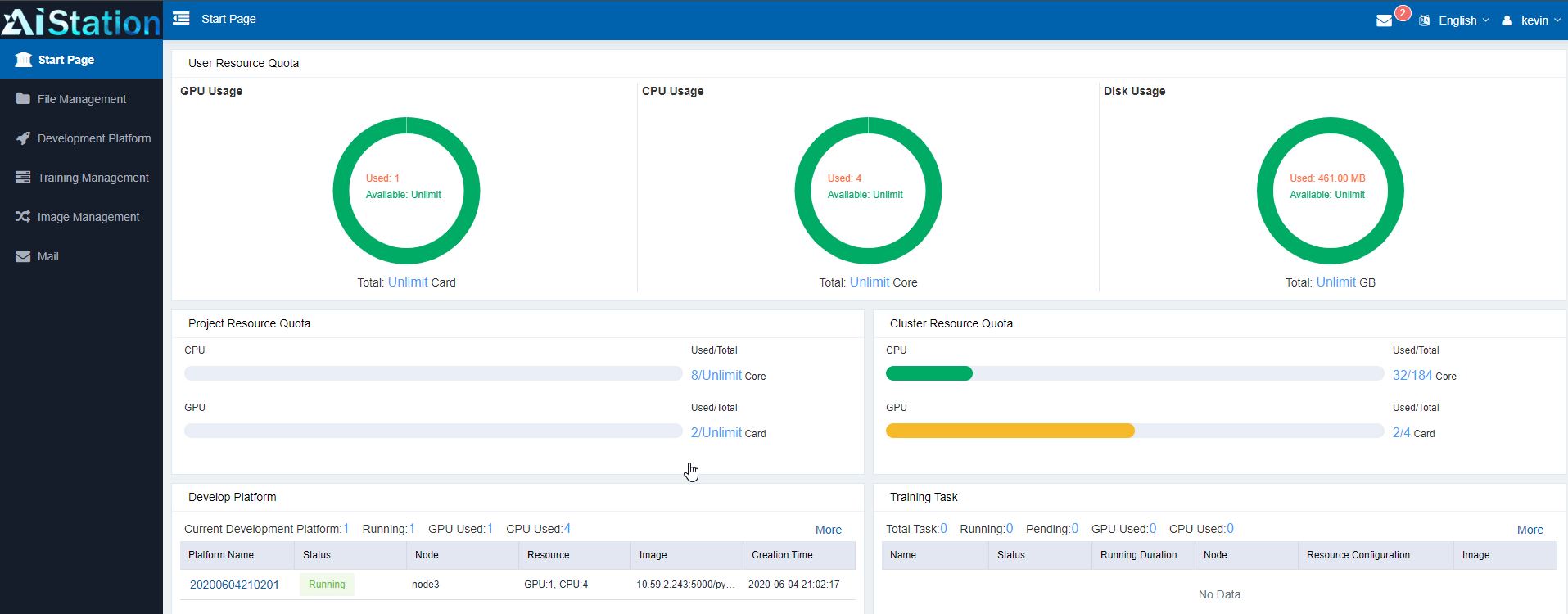
Paresh Kharya: Every industry is getting transformed by AI. Inference at the edge is critical for sensing the inputs, planning, and taking real-time actions.
Common use cases include radiology and patient care in healthcare, automation and quality control in manufacturing, predictive maintenance for industrial machines, fraud prevention and recommendations in finance, autonomous vehicles in transportation, inventory optimization and reducing shrinkage in retail, safety, and efficiency in smart cities and customer support across many consumer-facing industries and so on.
Patrick: How are customers managing AI deployments at the edge from an orchestration and infrastructure management standpoint?
Vangel Pojaxhi: With so many AI devices now at the edge, the uniform management, scheduling efficiency, and maintenance becomes a pain point for customers. In addition, because of the limited storage capacity of edge AI devices, it is necessary to automate their deployment, operation, upgrade, and maintenance. Lightweight OTA, for example, is an option.
Inspur’s AIStation enables the agile deployment and management of inferencing resources. It reduces the time required for model deployment from days to minutes by supporting the unified scheduling of multi-source models. It effectively helps enterprises easily deploy AI inferencing services, thus improving the efficiency of AI deployment and management.
Paresh Kharya: Edge computing presents a unique set of challenges. With distributed deployments installing hardware, ensuring connectivity, deploying software, maintaining upgrades, ensuring security and data privacy at each individual location is a daunting task.
To solve these challenges, NVIDIA has several tools available to organizations looking to manage AI deployments across their edge infrastructure. NVIDIA Fleet Command is a hybrid cloud platform for securely deploying, managing, and scaling AI at the edge. From one control plane, anyone with a browser and internet connection can deploy applications, update software over the air, and monitor location health. Fleet Command combines the benefits of edge computing with the ease of software-as-a-service.
Patrick: In the future, how will the DPU and future BlueField products that combine DPUs and GPUs impact the edge market for AI inferencing?
Vangel Pojaxhi: DPUs will first be deployed in a data center before moving to the edge. We anticipate that the BlueField products that combine DPUs and GPUs, like BlueField-2X, will improve the efficiency, speed, accuracy, and security of Deep Neural Network real-time inferencing, by increasing the network security, lowering inferencing latency, and increasing the hardware deployment density.
As of now, it is still rather difficult to assess the demands for DPUs in the edge scenarios.
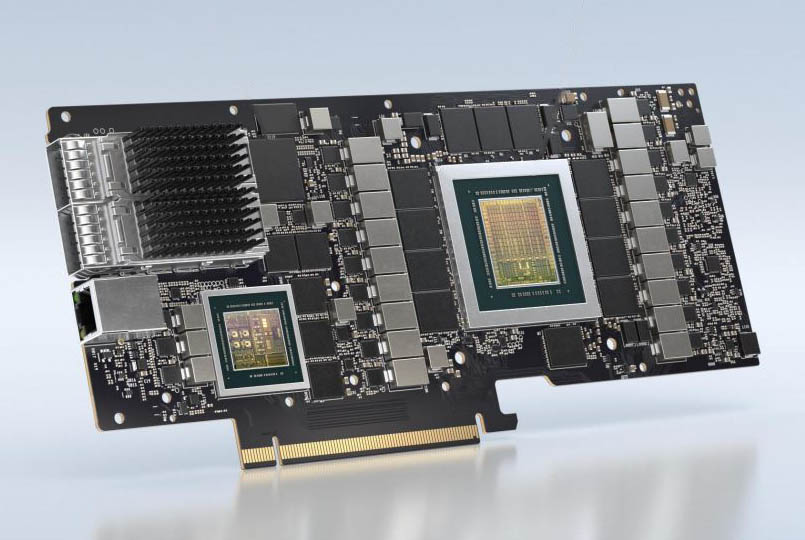
Paresh Kharya: AI at the edge needs acceleration, as well as security and safety since some of the data they will process from healthcare devices to industrial automation to home devices, involves information that needs to be handled with care and sensitivity. And because these devices are spread out they are going to need fast reliable connectivity to function and coordinate.
NVIDIA EGX edge AI platform combines our GPUs to enable real-time AI inference with advanced models across various use cases and our BlueField DPUs to enable isolated security domains for robust threat protection while enabling low latency communications. And combined with our EGX stack and software including NVIDIA fleet management enables production deployment and management of edge AI infrastructure.
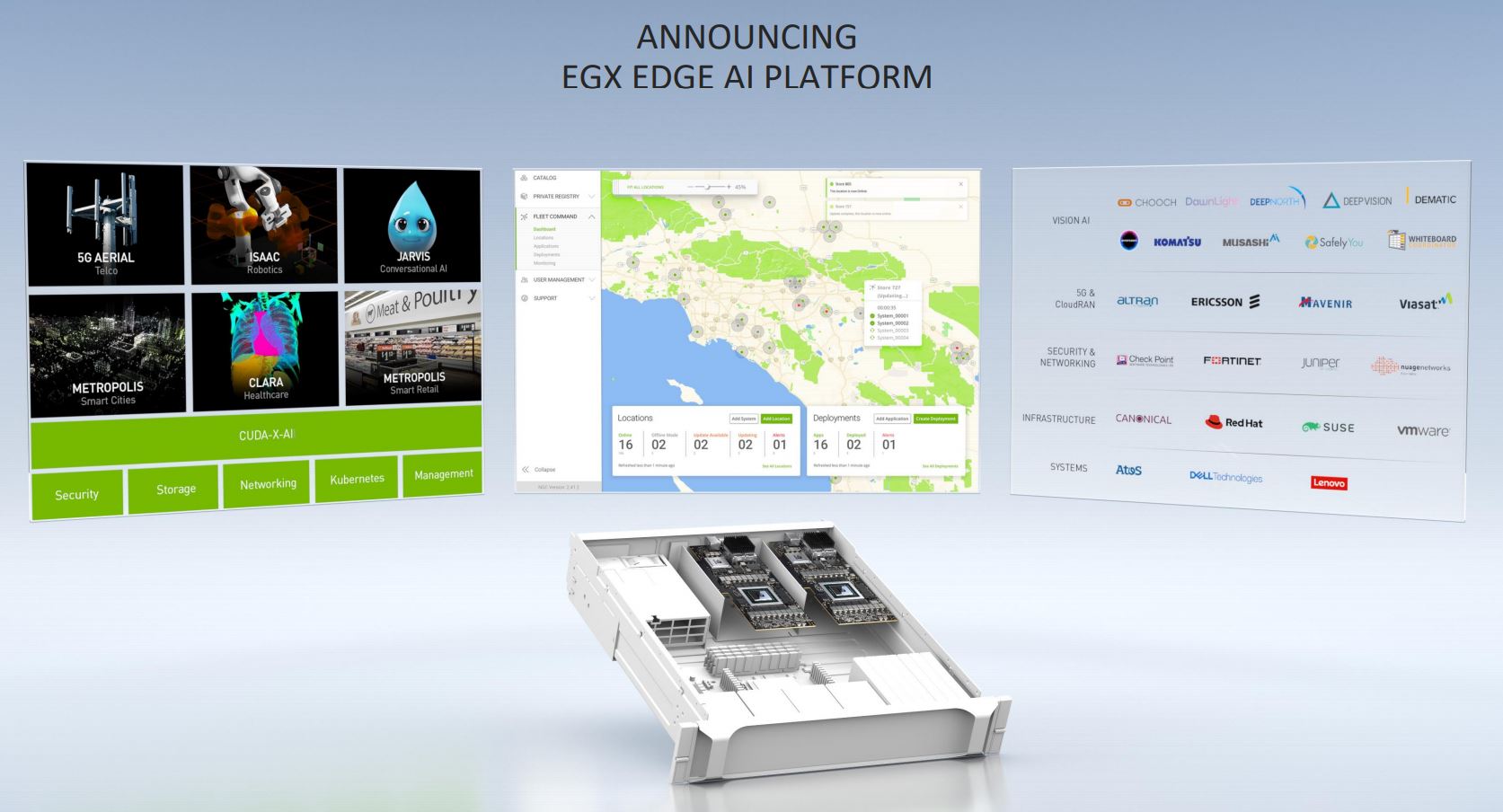
Data Center
Patrick: How have AI training clusters in the data center changed between the Volta and Ampere launches?
Vangel Pojaxhi: During the past few years, AI technologies and applications have developed rapidly, along with the complexity of neural network models. NLP models, such as Bert/GPT2/GPT3, and large-scale recommendation models, such as DLRM, have emerged. Among them, GPT3 has more than 170 billion parameters. Higher demands on the computing platform require further expansion of AI training clusters and faster interconnection among clusters.
To meet these demands, NVIDIA has launched NVIDIA Ampere architecture, the next-generation platform, which doubles GPU performance, improves TF32 performance by nearly 20 times, and significantly increases cluster communication speed by fully supporting HDR 200G.
Inspur AI servers fully support the NVIDIA Ampere architecture, enabling AI training clusters to achieve higher performance and faster network communication. For example, the Inspur record-breaking product NF5488A5 is tested to deliver 234% performance increase in comparison with the previous server generation. This server also set 18 world records in the MLPerf 0.7 AI benchmark performance test including executing 549,000 inferences per second in ResNet50 inference task test. This is three times higher than the best server performance record of 2019.
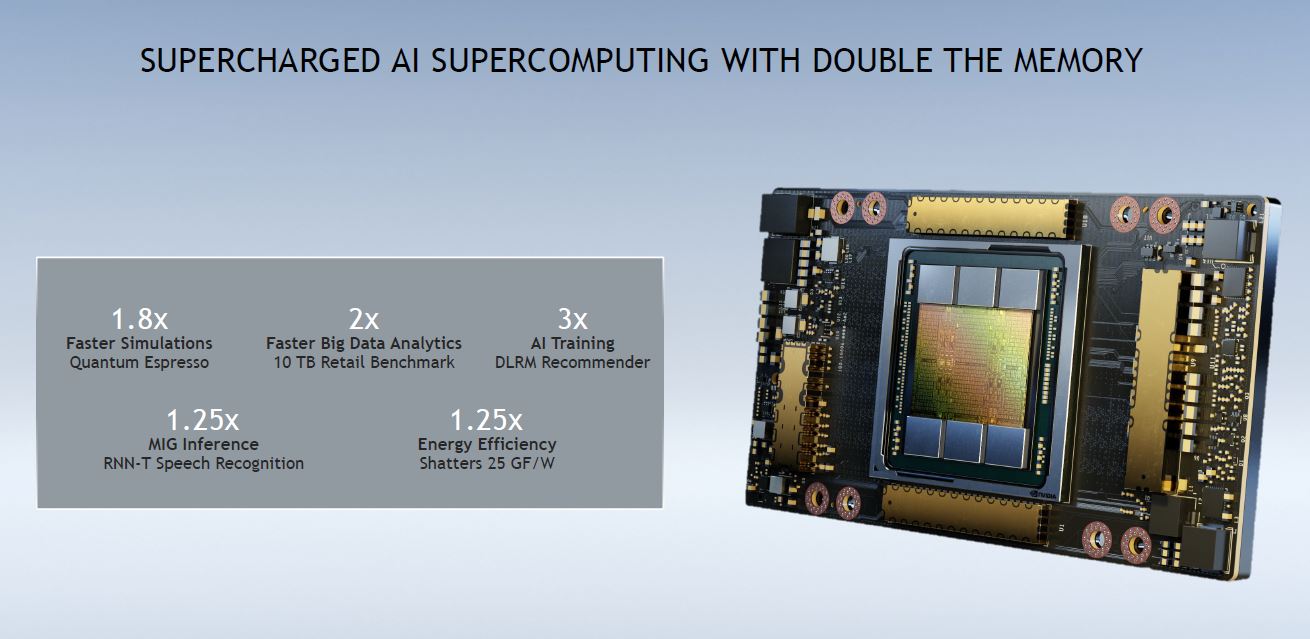
Paresh Kharya: The biggest trends in AI are the tremendous growth in size and complexity of deep learning models and data sets. We’ve seen the computing requirements to train advanced AI models increase 30,000 times since the launch of Volta – from computer vision model ResNet-50 then to natural language understanding models like GPT-3 today. Recommendation systems, the engines of the internet today frequently involve data sets that are in tens to hundreds of terabytes.
So the AI training clusters have scaled to thousands of GPUs interconnected by extremely high bandwidth networking fabric.
NVIDIA has enabled this transformation with a range of new technologies combined with a productive and programmable platform. 3rd generation tensor cores that provide an up to 20x higher peak performance. World’s first 2 TB/s DRAM bandwidth with large 80GB memory. 3rd generation of NVlink and NVSwitch that interconnect every GPU with every other in a server with 600 GB/s. Mellanox Infiniband networking to enable efficient scaling to thousands of GPUs in a cluster. Magnum IO software to enable efficient scaling with GPU-direct RDMA and GPU-direct Storage technologies.
Patrick: What is different about the software orchestration and infrastructure management of data center AI clusters today versus when Volta was first announced?
Vangel Pojaxhi: AI clusters in data centers require more fine-grained resource scheduling to improve resource utilization and meet the requirements of multi-task training and development scenarios. Also, the deep learning framework parallel scaling across cluster nodes is required to meet the training needs of large-scale models.
The MIG technology can divide a single A100 into up to seven independent GPU instances, each of them executes different computing tasks. Through more granular allocation of GPU resources, it can provide users with more efficient accelerated calculations and increase GPU utilization to an unprecedented level.
With the memory size twice as large as its predecessor’s, A100 80GB GPU can be used to train the large models that involve more parameters, such as Bert. It can avoid the slow and time-consuming operations across nodes in the traditional training.
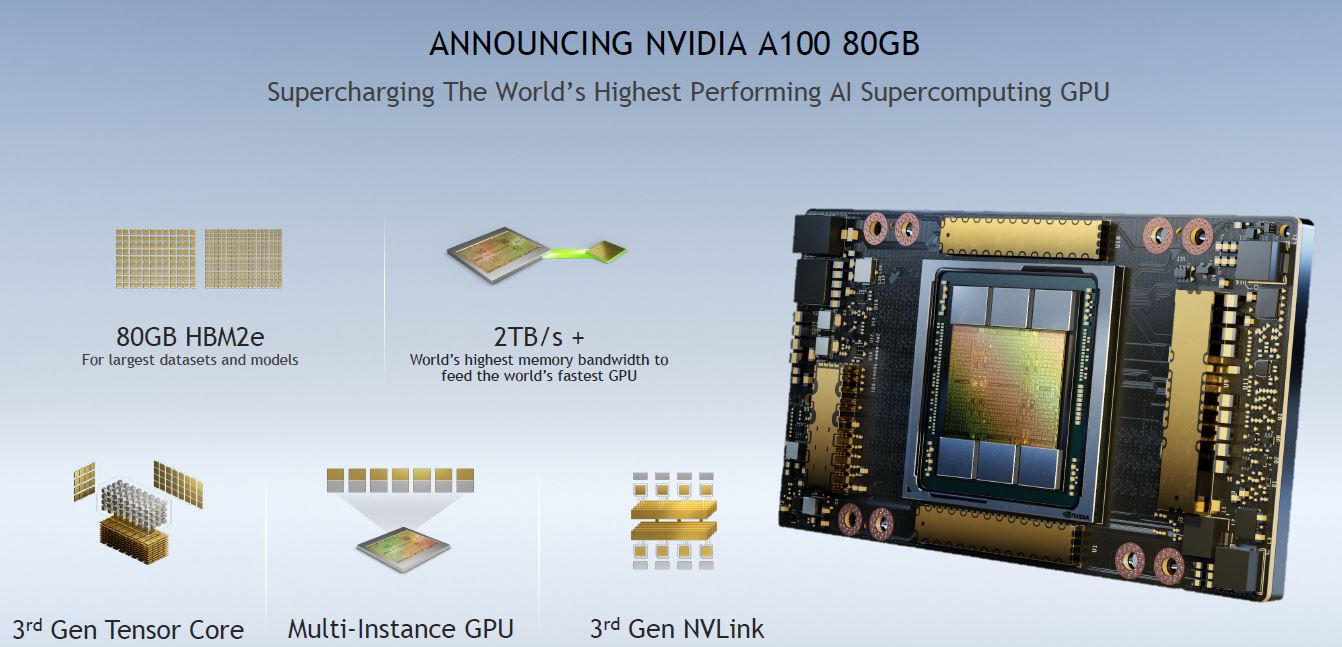
Paresh Kharya: AI workloads are everywhere. So all enterprise data centers now need to be prepared to run AI. From specialized scale-up clusters used for training large AI models to mainstream enterprise data centers and edge infrastructures running AI-infused applications.
NVIDIA is working with our ecosystem and partners to enable this transformation and making GPU accelerated servers operate in existing standard IT operational frameworks. GPU acceleration is natively supported in Kubernetes and we are working with partners such as VMware & RedHat to make NVIDIA GPU acceleration first-class citizens on their platforms.
Patrick: The latest MLPerf 0.7 Inference results had a heavy focus on NVIDIA’s server inference acceleration. How is the AI accelerator in-server market growing (perhaps looking at the last year and projections for the next year for context)?
Vangel Pojaxhi: The demands for networking and intelligence have been increasing during the COVID-19 pandemic. To improve the online experience of short videos, live streaming, distance learning, and online shopping, CSPs have made every effort to develop AI applications, driving the growth of AI-accelerated chips.
Over 50% of enterprise-generated data will be created and processed at the edge by 2022, while the percentage is currently less than 10%. Inferencing chips will grow rapidly in the AI-driven scenarios, such as telemedicine, autonomous robots, intelligent manufacturing, and automated driving.
IDC predicted the AI inferencing will continue to grow faster and will exceed AI training market by 2022. Inspur aligns more closely with this trend and will integrate the latest GPU/DPU technologies from NVIDIA to deliver to our customers a richer AI ecosystem and AI product portfolio of super-hybrid server architecture designs particularly for inferencing.
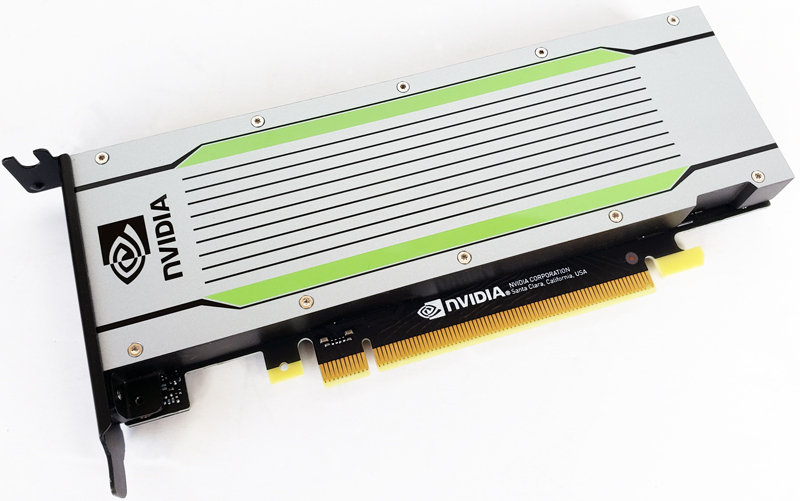
Paresh Kharya: AI is expanding from the cloud to enterprises. AI and data-driven applications are transforming every industry. Enterprises are moving from a few experimental AI applications to a future where every enterprise application will be AI-infused and constantly improved with deeper data-driven insights.
For AI acceleration we continue to see explosive growth in AI model complexity, data set sizes, and types of different AI models required for different use cases. This affects both training and inference, driving the need for ever-increasing amounts of versatile AI acceleration.
Hardware needs to be easily programmable with performant and productive software to accelerate a widening range of models and use cases, from images to fraud detection, to conversational AI to recommenders and beyond.
Many of the AI services being built now make use of an ensemble of networks, where over 10 networks must complete their inference computing and return an answer in less than a second. And while it’s readily understood that AI training requires acceleration, these trends make AI inference acceleration a must-have as well.
Patrick: In the future, how will the DPU and future BlueField products that combine DPUs and GPUs impact the data center?
Vangel Pojaxhi: DPUs will process the data traffic that flows into a cloud data center in an intelligent way. Hence, the processing of data traffic from the network, storage, virtualization, or other sources will be offloaded from hosts to DPUs. It will substantially release the computing resources of CPUs and significantly improve the overall processing capabilities and project turnaround time in data centers.
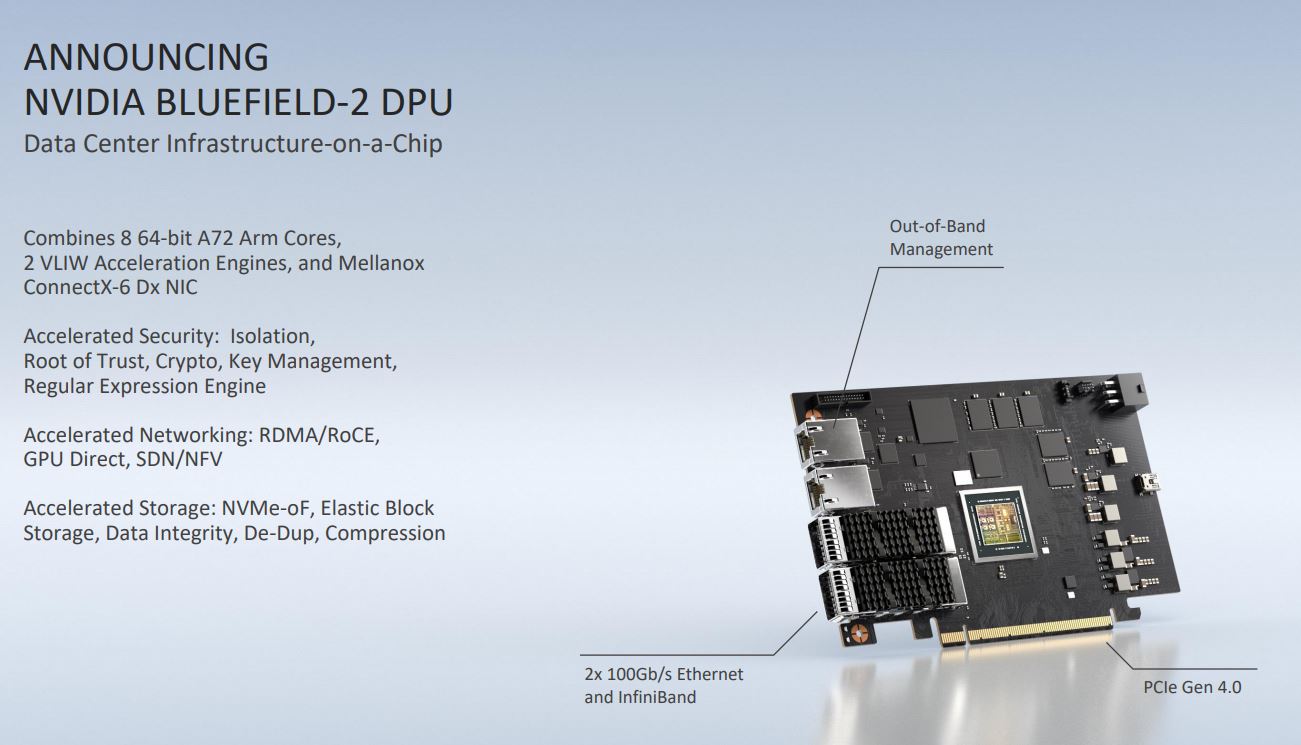
Paresh Kharya: Modern, secure, and accelerated data center enterprise infrastructure will be built with the three major pillars of computing: CPU, GPU, and the Data Processing Unit (DPU).
The data processing unit (DPU) is a new accelerated computing element that improves the performance, efficiency, scalability, and security of enterprise workloads.
NVIDIA BlueField-2 is the world’s most advanced data processing unit (DPU), combining the networking power of the ConnectX-6 Dx SmartNIC with programmable Arm cores plus offloads for traffic inspection, storage virtualization, and security isolation. The NVIDIA DPU frees up CPU cycles for applications while enhancing, security, efficiency, and manageability of every host.
NVIDIA DPU is being tightly coupled with the NVIDIA GPU in the NVIDIA EGX platform to deliver unmatched capabilities. GPUs enable the highest throughput processing for parallel applications, accelerating AI, HPC, data analytics, and graphics. DPUs enable isolated security domains for robust threat protection while accelerating networking, storage, and security.
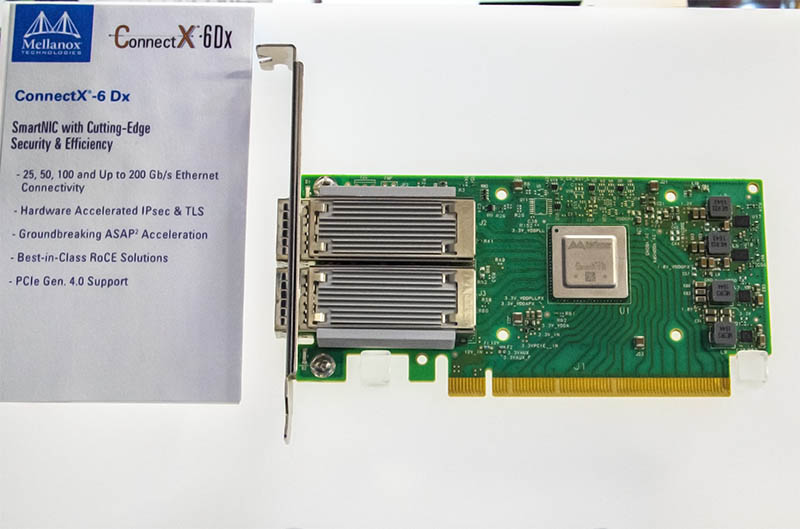
Looking to the Future
Patrick: For a company looking to embark on a new AI hardware project today, what are the key aspects they should be focusing on in the data center (compute/ networking/ storage)?
Vangel Pojaxhi: Computing power is the most crucial factor. While there is still plenty of room for innovation in new hardware chips, cards, servers, and networks, the focus remains to increase the productivity of the entire system. Emphasis should be placed on how to uniformly manage and schedule AI computing resources, centrally manage data and streamline the development of AI models.
For example, AIStation and AutoML suite developed by Inspur can help users to build an agile and effective AI resource platform, increase the efficiency of developing AI models, accelerate the research, development, and innovation of AI technologies and enhance the competitiveness of their products.
Paresh Kharya: Enterprises need to modernize their IT infrastructure so they can keep up with the demands of new applications, and still be able to operate within existing standard IT operational frameworks. The next generation of datacenter will need to be built with the ability to run modern applications natively, alongside their existing enterprise applications, and flexible enough to power a diversity of workloads, including the ones not yet invented. The platform should also be able to do this in a cost-effective and scalable way.
The NVIDIA Platform provides a way for customers to run many diverse modern applications on a single highly-performant, cost-effective, and scalable infrastructure. It brings together compute acceleration and high-speed secure networking onto an enterprise datacenter platform. This platform includes a vast set of software that enables users to become productive immediately and can be easily integrated into industry-standard IT and DevOps frameworks, allowing IT to remain in full control.
Accelerated computing requires accelerated input/output (IO) to maximize performance. NVIDIA Magnum IO is the architecture for parallel, asynchronous IO, maximizing storage and network IO performance for multi-GPU, multi-node acceleration. Magnum IO supports NVIDIA CUDA-X libraries and makes the best use of a range of NVIDIA GPU and NVIDIA networking hardware topologies to achieve optimal throughput and low latency.
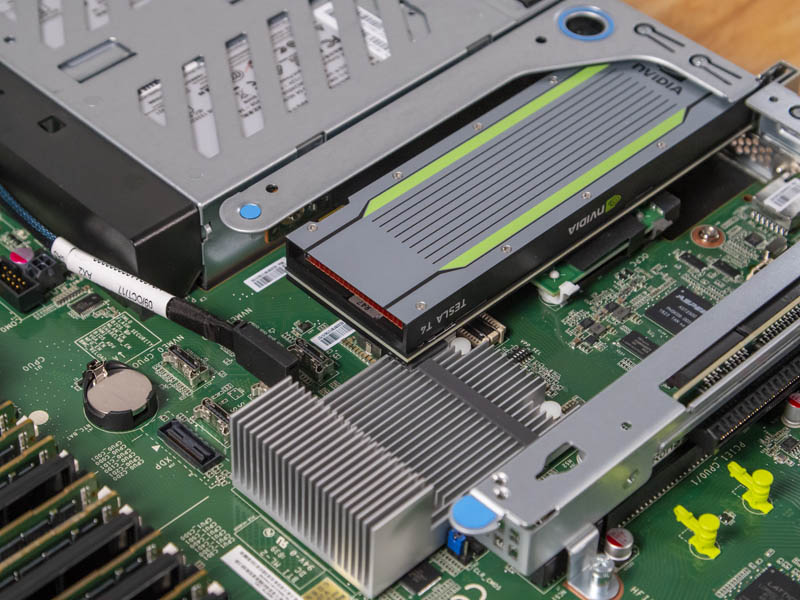
Patrick: Looking ahead to 2021, what do you expect will be the biggest trend we see next year?
Vangel Pojaxhi: With the development of 5G and IoT, a diverse range of applications will emerge in the fields like automated driving and industrial robots. Thus, AI-driven edge computing is likely to grow spectacularly
Paresh Kharya: AI and data-driven applications will continue to transform every industry. Enterprises are rapidly moving from piloting AI applications to a future where every enterprise application will be AI-infused and constantly improved with deeper data-driven insights. We expect this trend to continue next year.
Monolithic applications are giving way to microservices-based modern applications. Modern applications use components and services that are specialized, loosely coupled, and deployable, and scalable anywhere—using on-premises, cloud, and edge computing resources as needed.
Finally, enterprises will make progress implementing zero-trust security models, augmenting security at the perimeter with securing every host.
Patrick: If you could change one practice we have in the industry for AI training or inferencing, what would it be?
Vangel Pojaxhi: We hope that an open and general hardware and software platform specifications can be established for AI-driven computing so that the cooperation in the AI technical innovation can be more agile and effective.
Paresh Kharya: The average upgrade cycle for data center infrastructure has nearly doubled from 3 years to over 5 years in the last decade. The infrastructure deployment decisions made today will need to support not just current workloads but the emerging requirements years into the future.
So instead of buying more of the same, successful organizations do detailed workload analysis of current and emerging workloads. And choose a platform that offers performance, programmability, and productivity to accelerate a widening range of AI models and use cases, from computer vision to fraud detection, to conversational AI to recommenders and beyond.
Final Words
First off, thank you to Vangel and Paresh for answering my questions. The interview guide I drafted with questions to ask both was probably a bit on the long side so I appreciate the extra effort. Also, thanks to the teams at Inspur and NVIDIA that helped put this together.
2021 is shaping up to be an exciting year. A number of silicon products are going to come to market in 2021 that will enhance compute, accelerated compute, networking, and storage. 2020 was a relatively slower year for the industry as a whole in terms of hardware innovation which makes the software side more exciting. Looking ahead, 2021 is going to be an enormous year for this space.