Kicking off our Hot Chips 32 coverage, we are going to take a detailed look at the NVIDIA DGX A100 SuperPod design. Earlier, Cliff took us through how NVIDIA built its Selene supercomputer, currently #7 in the world, during the pandemic in 0 to Supercomputer NVIDIA Selene Deployment. Now, we are going to look more at what goes into that type of machine. Note we are going to update this as the talk continues.
NVIDIA DGX A100 SuperPod Nodes
We previously covered the NVIDIA DGX A100 so you can see a bit more about the solution there. The key is that each system has 8x NVIDIA A100 GPUs (no longer a “Tesla” brand”.) NVIDIA detailed a bit more about what is behind the system.
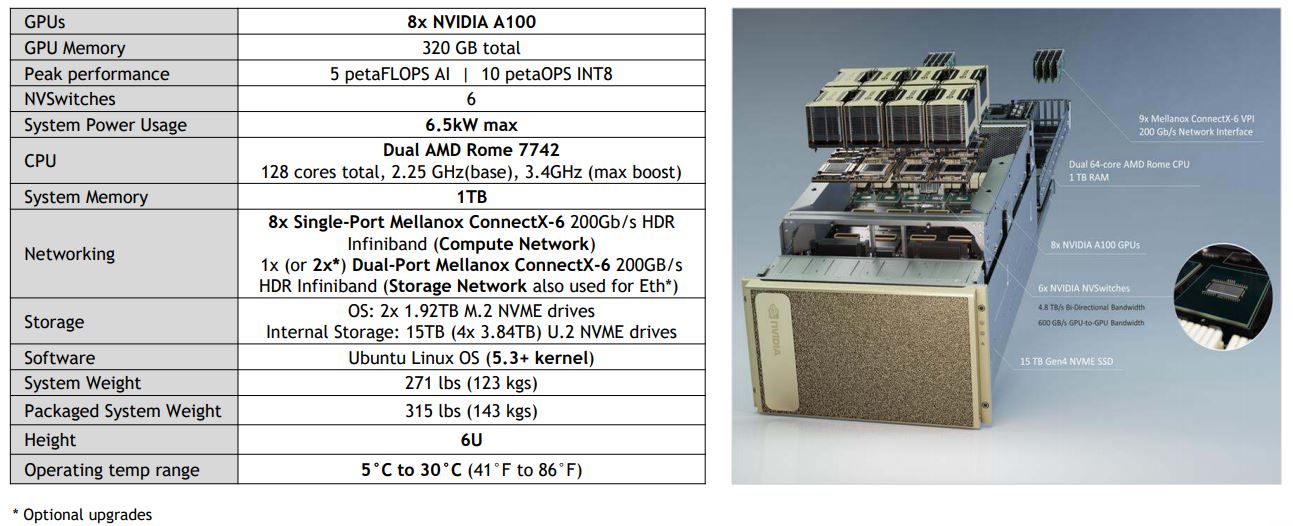
Some of the interesting facts, we see that NVIDIA is indeed using AMD EPYC 7742 SKUs, not a custom SKU. The system also has a few options. For example, one can have a second dual-port Mellanox ConnectX-6 card for the storage network and optionally use for Ethernet.
In terms of topology, the new DGX A100 now uses a 1:1 GPU to NIC ratio. In the previous generation, we generally saw 2:1 ratios. Along with that, the NICs have doubled in speed. Instead of a 100Gbps EDR adapter per two GPUs we now get 2x 200Gbps HDR IB adapters for two GPUs.
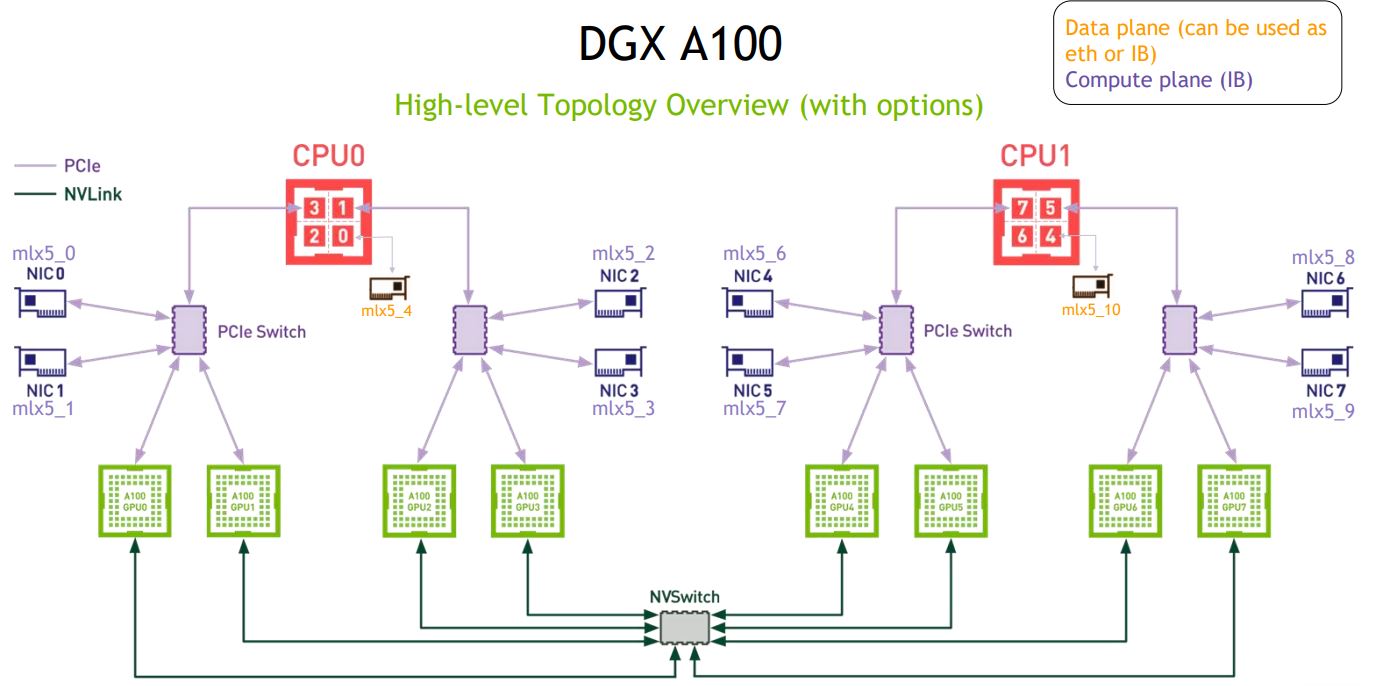
There are four PCIe switches and six NVSwitches onboard. The NVSwitch in the image should be “NVSwitch fabric.” In terms of networking, we get a data plane that can be Infiniband or Ethernet. Each CPU gets its own NIC so the system does not need to cross NUMA nodes. If you want to see the previous generation where we take a look at the NVIDIA V100 NVSwitch solution, check out our Inspur NF5488M5 Review.
NVIDIA DGX A100 SuperPod Design and Topology
Here is how NVIDIA DGX A100 is designed with modularity. As one can see there are racks for local networking, compute, the centralized fabric and management, and storage racks. NVIDIA is using this model to both allow for upgrades but also to make the solution modular so one can deploy many of these SuperPods quickly.
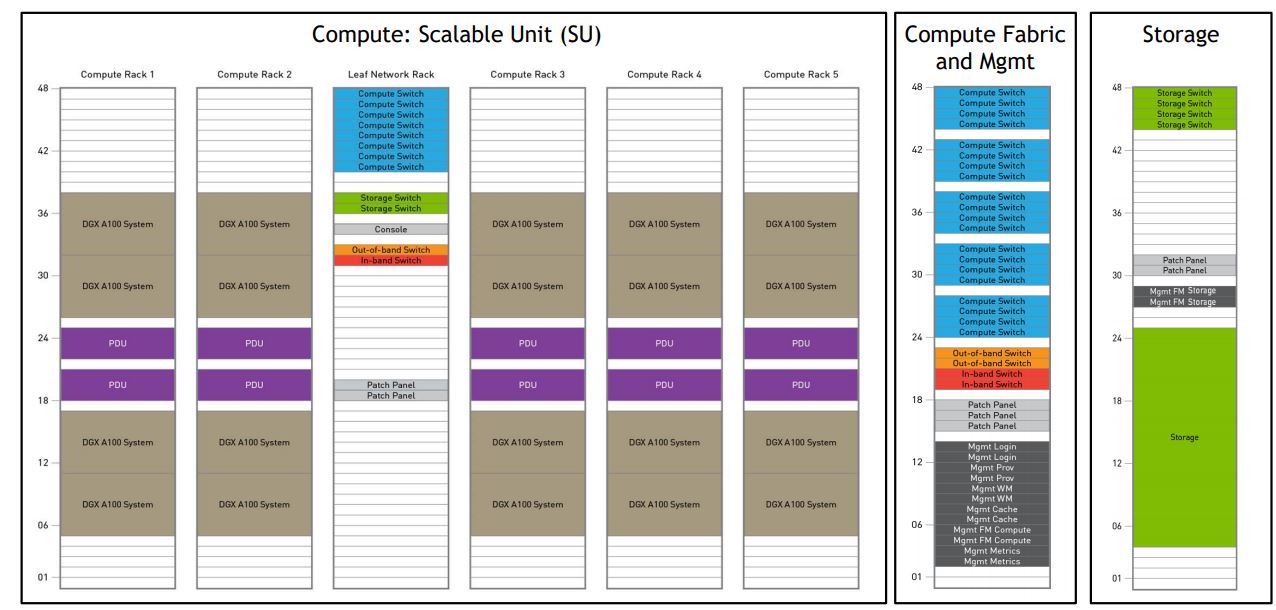
Each SuperPod cluster has 140x DGX A100 machines. 140x 8GPUs each = 1120 GPus in the cluster. We are going to discuss storage later, but the DDN AI400x with Lustre is the primary storage. NVIDIA is also focused on the networking side using a fat-tree topology.
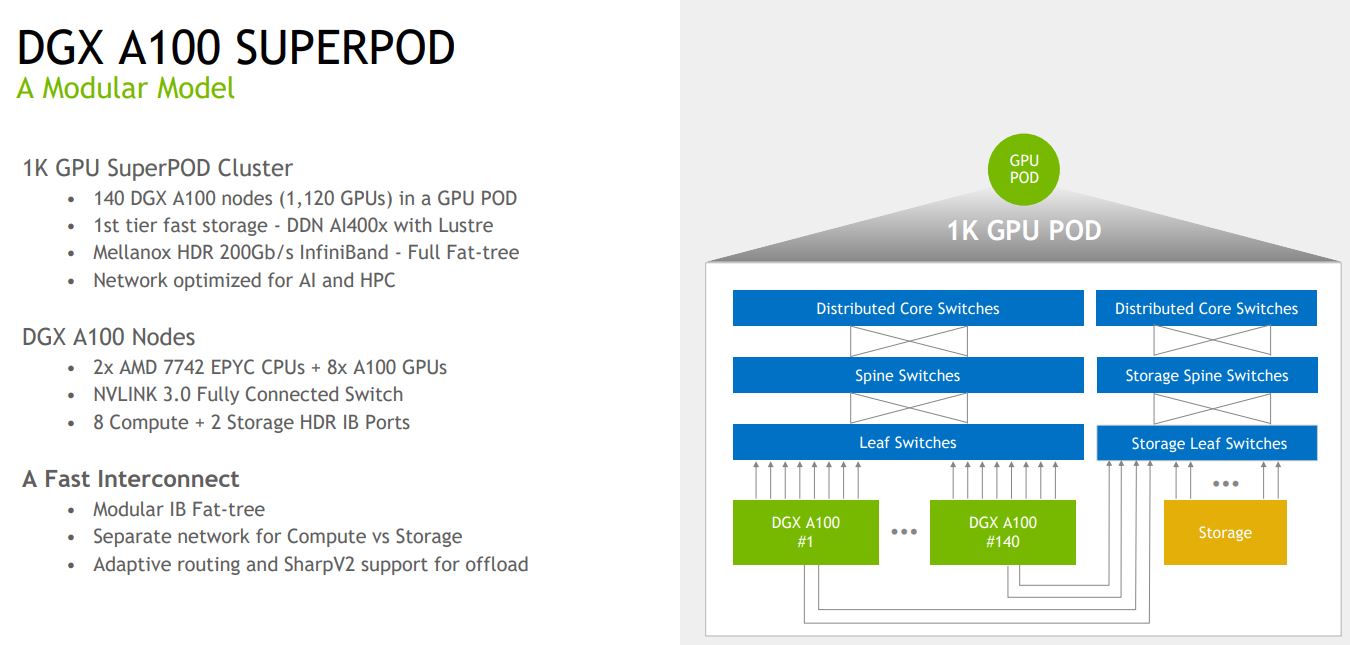
NVIDIA also designed the SuperPod to scale to multiple SuperPods. Here, NVIDIA is using Fat-tree or DragonFly+. Some of the 3D torus topologies are less efficient when it comes to cabling.
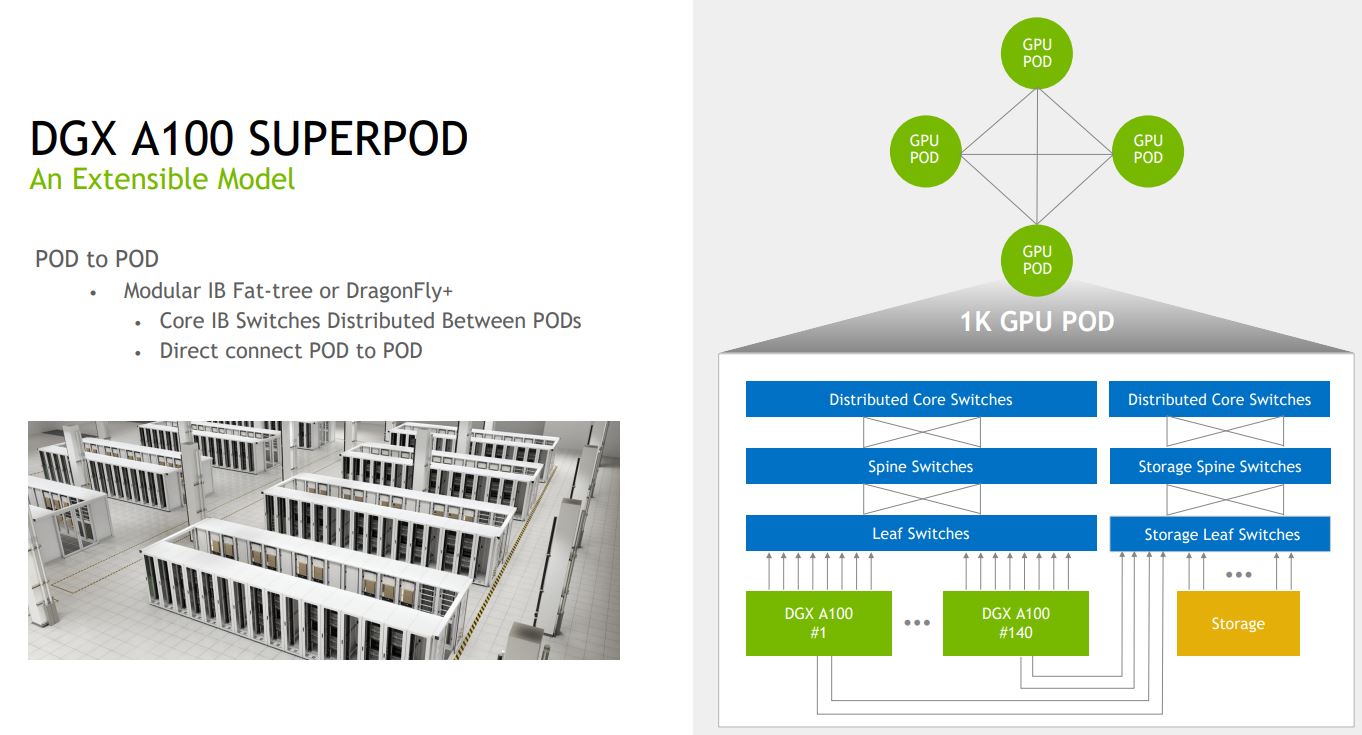
Each DGX A100 gets 8x HDR 200Gbps Infiniband links for compute and two for storage.
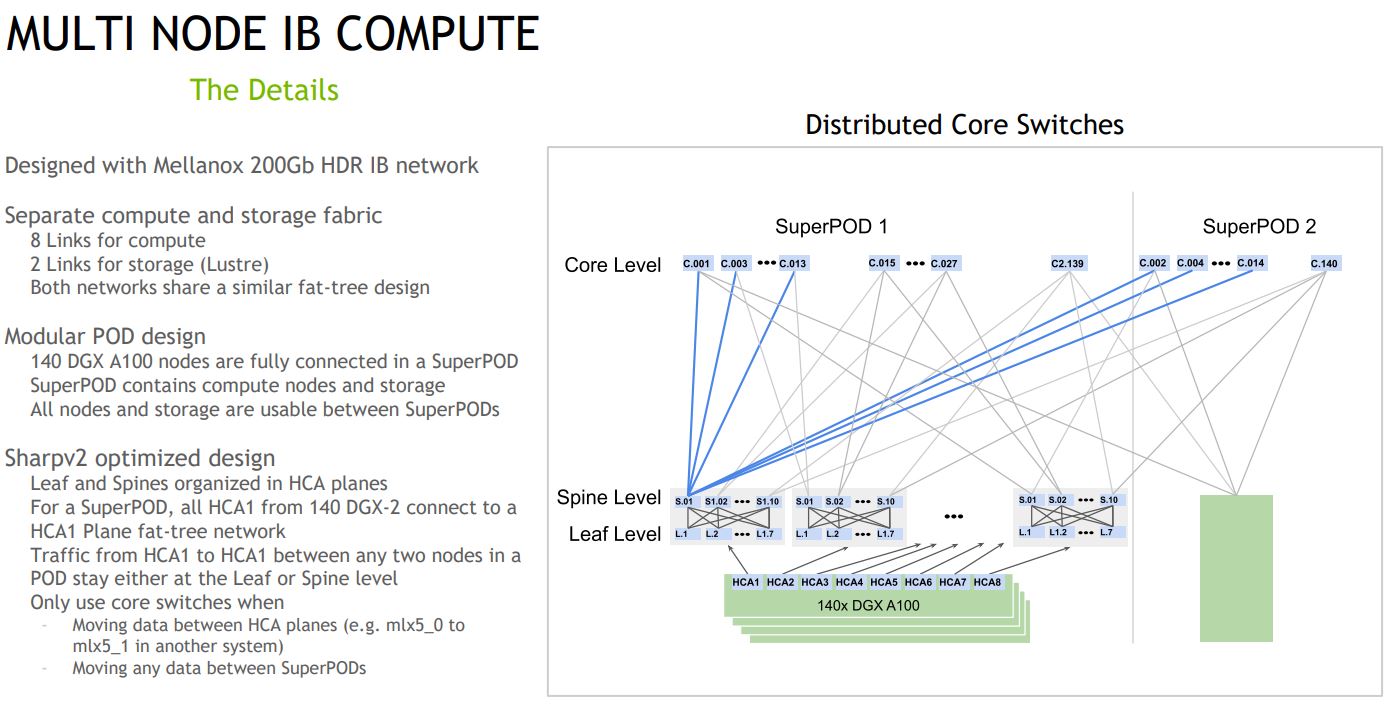
Here we can see what the networking looks like. On each side of the system we have the compute HDR IB cards.
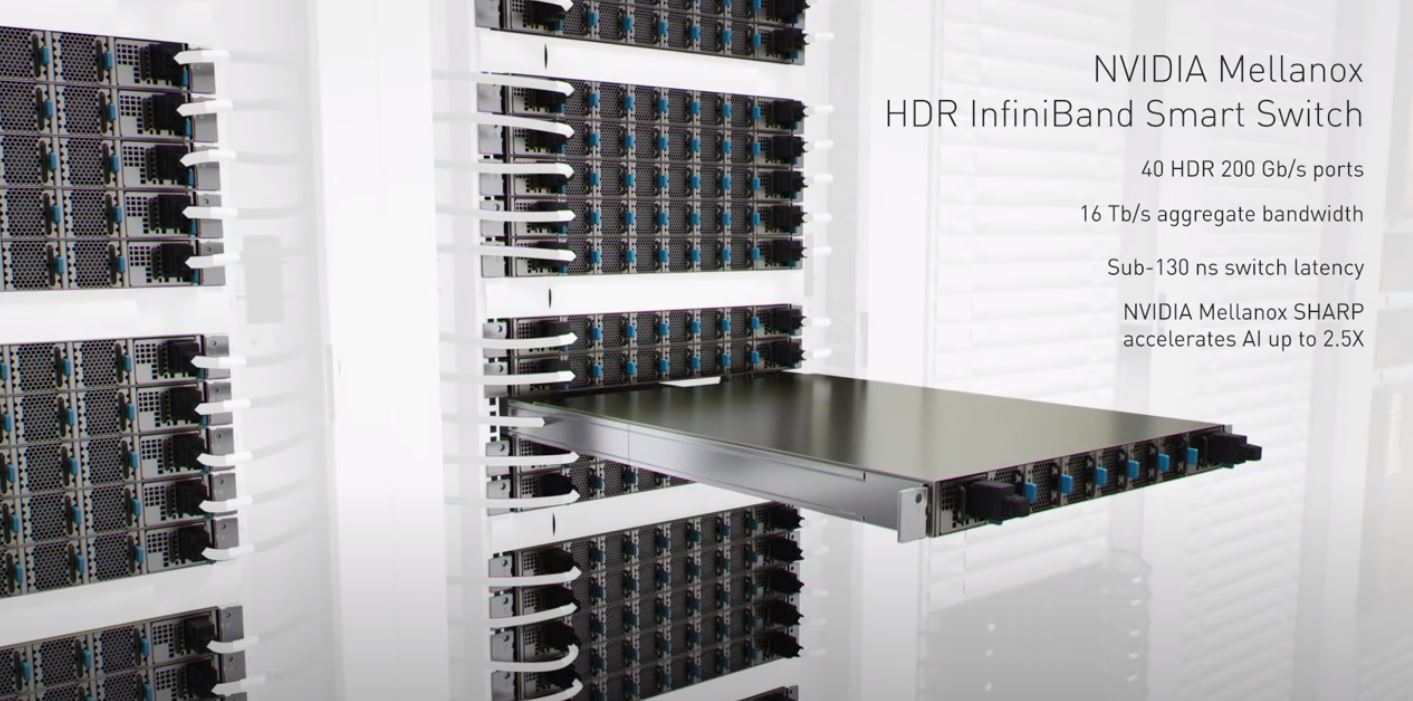
There are two storage cards with another port being used for in-band management on one of the storage NICs. There is an additional IPMI OOB management NIC.
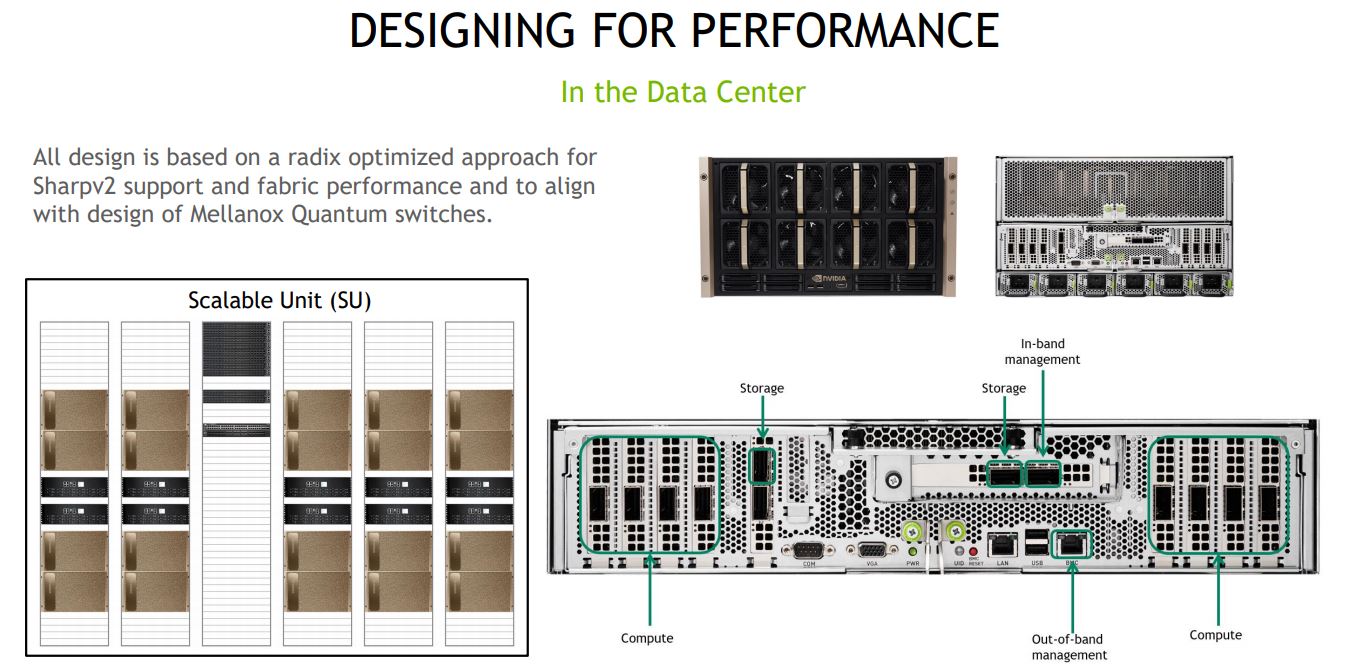
As part of what NVIDIA is doing with SHARP is that the Mellanox switches are actually doing some of the allreduce overhead computation which offloads this work from the expensive NVIDIA A100’s.
NVIDIA DGX A100 SuperPod Storage
Each SuperPod has a Lustre-based parallel filesystem at 2.5PB. These are based on DDN AI400X nodes and with ten of them, one gets 490GB/s read and 250GB/s write speeds at 16.6kW.
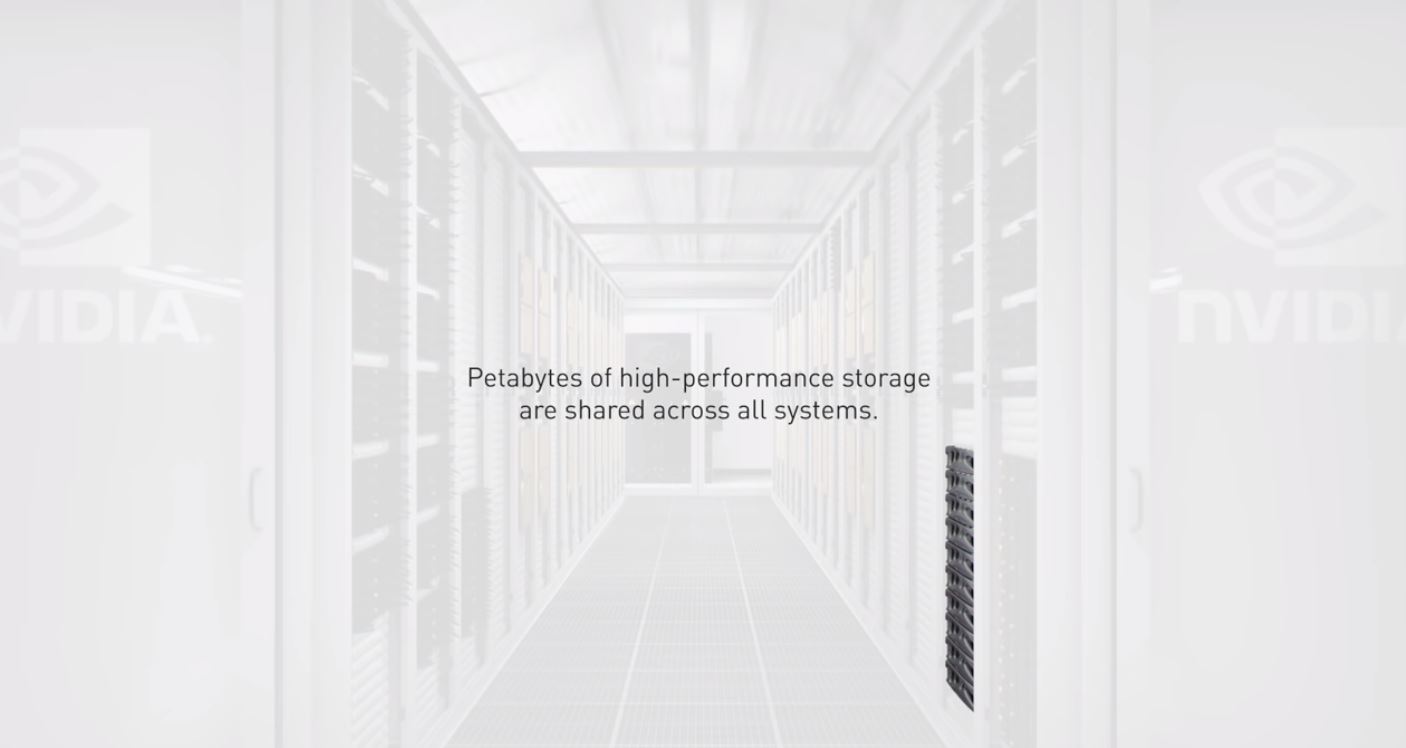
One also needs 80x network ports to power this storage. One can clearly see why NVIDIA was interested in Mellanox.
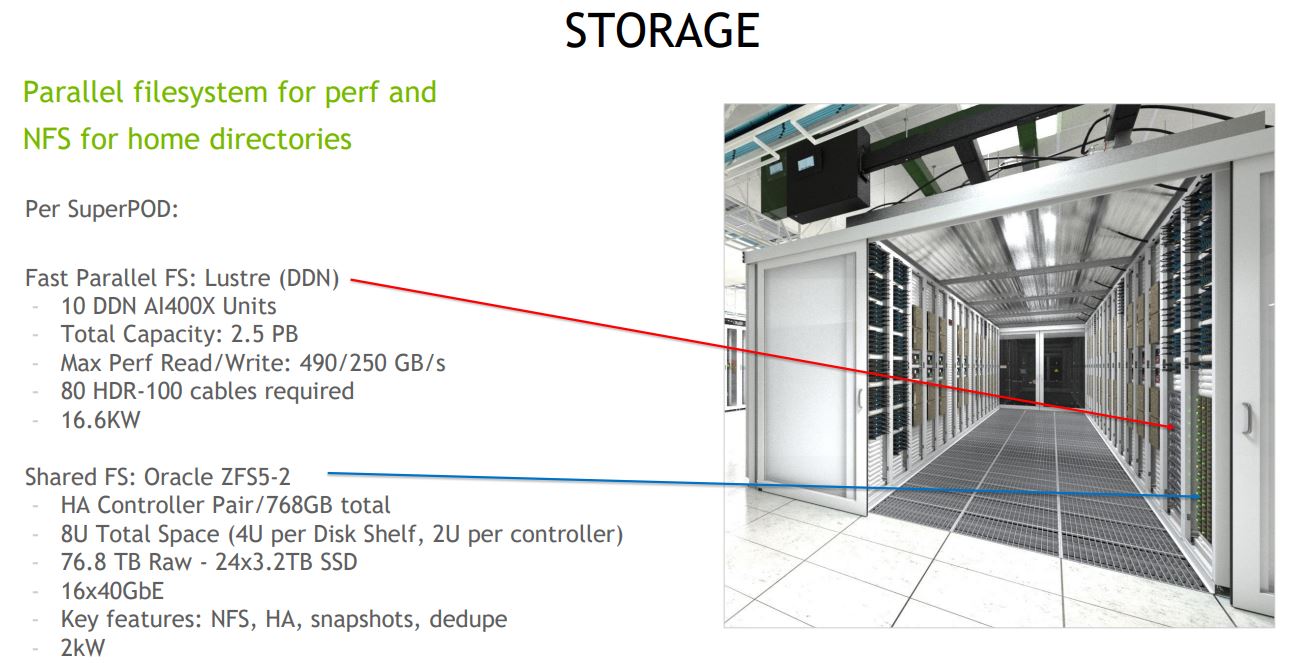
The remainder of the storage hierarchy, one has memory, NVMe Cache, this Lustre cache, and then object storage. We were told that this 100PB+ and 100GB/s object store is based on Ceph which we covered in our earlier piece.
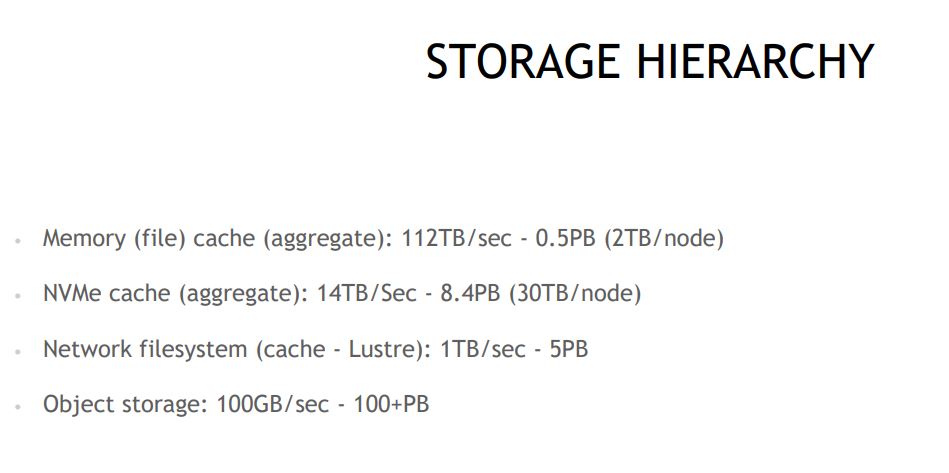
Where NVIDIA is clearly going here is moving from making GPUs to making complete solutions. The big question is how long the DDN machines last in future solutions before NVIDIA decides it can build or acquire the best clustered storage for AI and HPC workloads as well. NVIDIA has been working on the interconnect and software, so this is getting closer to becoming a reality.
Building a Multi-SuperPod Solution
We covered this more in 0 to Supercomputer NVIDIA Selene Deployment but NVIDIA optimized the in-data center deployment to require a minimum number of employees on-site. It even used a “Trip” robot to have its team remotely check LED status lights. That is a bit strange in some ways. One would expect those LED status lights to be remotely read on a system of this scale/ complexity.
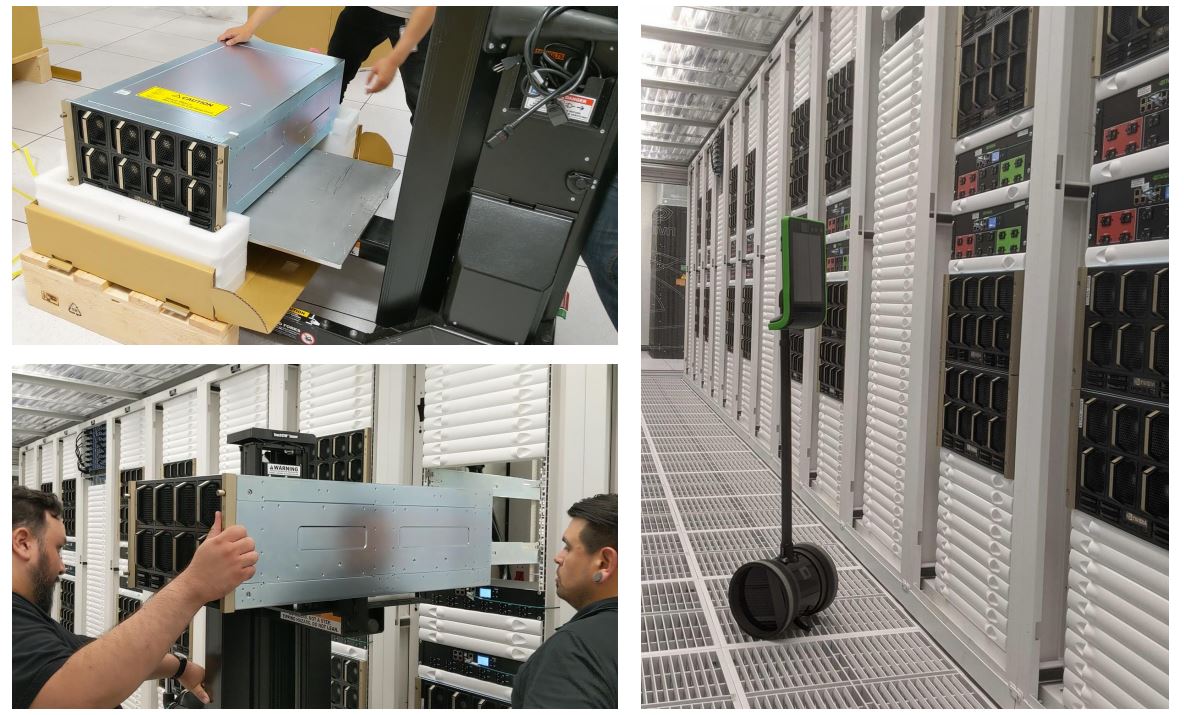
NVIDIA shared some of its cabling. By making a standard SuperPod deployment, it was able to standardize much of the cabling which means that cables could be bundled off-site and quickly deployed.
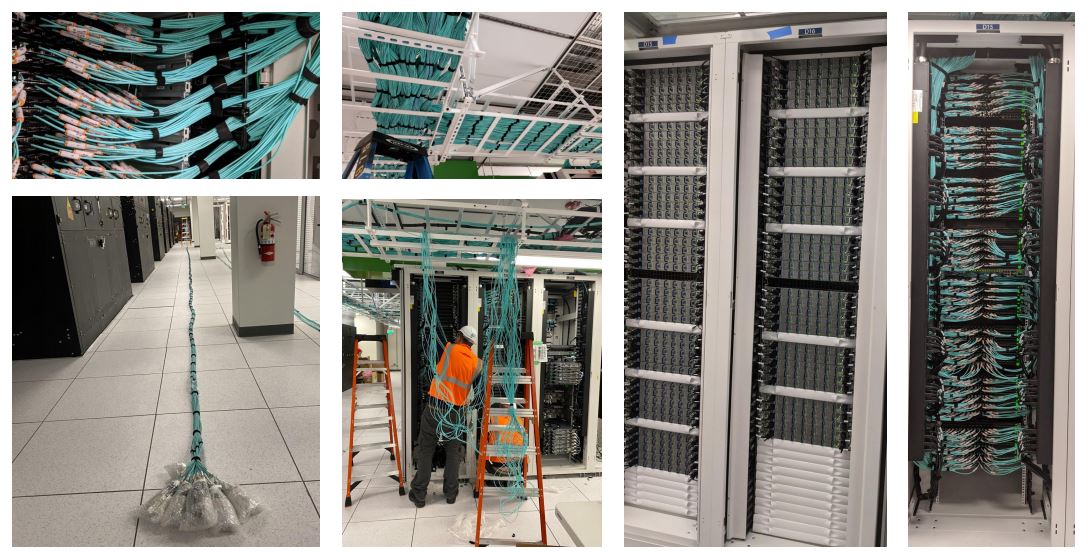
Cabling may not seem too exciting, but it takes an enormous amount of time in many supercomputer scale deployments. With this standardization, it makes on-site deployment significantly faster. NVIDIA also told STH that they leave a little bit of extra slack in each line to allow for future upgrades. This slack is needed in the event that ports move on future generations. Re-cabling a system this complex can be extremely costly so this is a way to reduce costs. We should also mention that Mellanox (now part of NVIDIA) can also supply the cables.
Final Words
Overall, one can see the clear direction that NVIDIA is taking here. The big question is how long until we get some of these features broken out further. With the NVIDIA EGX A100 NVIDIA can put the NVIDIA A100’s directly on the fabric. NVIDIA is pushing its software into the storage stack and other products such as Mellanox Bluefield-2 IPU SmartNIC can help change the storage paradigm. It seems like the next move here for NVIDIA is to tackle either the CPU side (with Arm?) and/or to start providing storage solutions that are accelerated by the company’s Infiniband and Ethernet fabric.
I got a kick out of learning the constraint on how fast they could assemble the system was the bandwidth of the loading dock to get systems from the truck to the building. :-)
What i would love to see would be a complete example on how these are used.
In my job, most of the stuff is web based, so you run with the most economical hard & software, So stuff like a HP DL360/380 with debian or RHEL on it, (lets call it the VW Golf of servers ;-))
What i would love to see:
– The Software Stack running on it.
– The Actual usecase (simulation, computation, whatever) with sample code
– Pictures of the Whole Setup
– An overview what the people working on this do on a day-to-day basis (and this for everyone involved, datacenter workers, sysadmins, devs, scientists etc)
– Especially NOT the “Whitepaper Interviews” where everyone just tells you how much they love the solution and how much faster it is than the old one (which, TBH should be the case anyway if you replace a 10 year old supercomputer)
Any additional details available about 100+PB object storage setup?