MLPerf Training 2.0 is out. As is custom, MLPerf Training is mostly an exercise for NVIDIA and its server OEMs. MLPerf Training at this point has tests that are exclusively, or almost exclusively NVIDIA benchmarks at this point. Still, there were a few more submissions, so we are going to focus on those.
Google TPUv4 Selectively Joins NVIDIA MLPerf Training 2.0
Perhaps the coolest entry in the MLPerf Training 2.0 results was the Google TPUv4 results. Google ran large clusters of TPUv4 machines against NVIDIA’s large on-prem clusters, and was able to show some impressive performance:
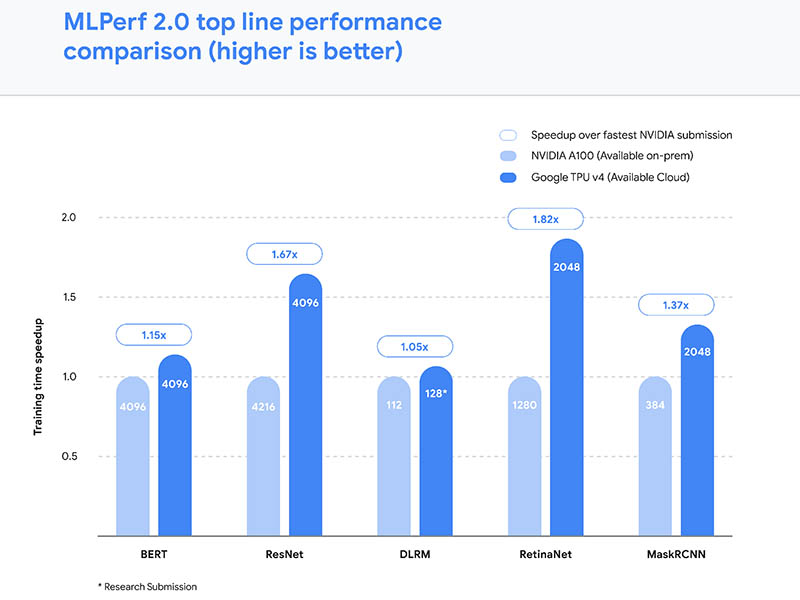
Google is eyeing Microsoft Azure as its competition here, so it is showing its cost to train at a whopping 4096 accelerator chips:
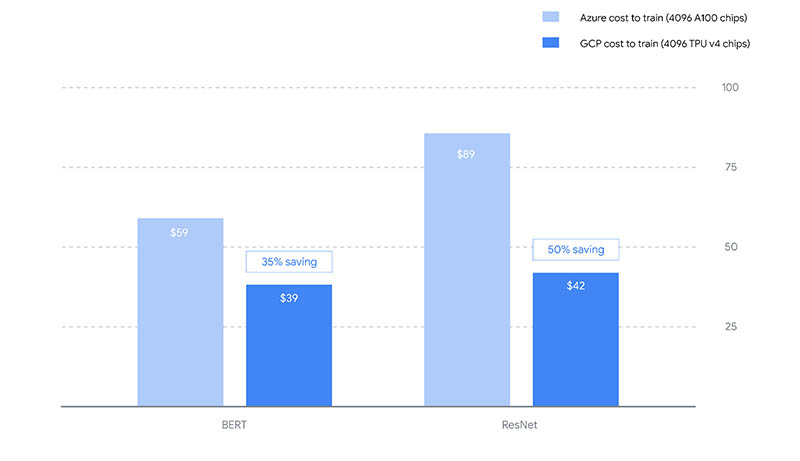
That along with the Intel Habana Gaudi2 was the most interesting data point.
Just for a sense of how NVIDIA-focused MLPerf is at this point:
- The ResNet ImageNet has the most diversity. In this one the Intel Habana Gaudi2 results seem to show that especially with 8x Gaudi2 chips and TensorFlow 2.8 it is a fast solution compared to the scores of NVIDIA MxNet results. Google TPUv4 was added here with two results of thousands of TPUv4’s.
- The KiTS19 medical image segmentation results were all NVIDIA-only in the closed division
- RetinaNet lightweight object detection was NVIDIA-only in the closed division
- COCO heavy-weight object detection was NVIDIA-only in the closed division
- LibriSpeech RNN-T was NVIDIA-only in the closed division
- The BERT Wikipedia NLP by far had the most diversity.
- The Intel Habana Gaudi2 again looks like a serious competitor for the NVIDIA A100 (although they are effectively different generations of cards given where Gaudi2 is launching in the A100 lifecycle.)
- The Graphcore machines make another underwhelming showing on the list.
- Microsoft Azure with its NVIDIA A100 machines and Google GCP with its TPUv4 went to benchmark clusters which were interesting. Perhaps the real contest though was between Google Cloud TPUv4 and NVIDIA’s own large-scale cluster A100 benchmarking here since both of those solutions scaled to over 4000 accelerators in this test
- The Recommendation Engine DLRM test had only NVIDIA-based submissions under 128 accelerators. Google had a single 128 TPUv4 submission that could not really be compared with anything in a meaningful manner. That single result that was on a different scale was the only thing not making this a NVIDIA-only test.
- The Reinforcement MiniGo benchmark was NVIDIA-only.
There is a real lack of diversity with MLPerf Training. Luckily the inference efforts seem to have better representation.
Final Words
With so little competition in MLPerf Training, we are now just calling this the NVIDIA MLPerf Training benchmark. NVIDIA did not just have the majority of MLPerf Training 2.0 results, but if it was not for the single TPUv4 DLRM test (noted above on a different cluster scale) a full 75% of the workloads in the closed division would have had only NVIDIA-based accelerator submissions. We are using “NVIDIA-based” to cover either NVIDIA submitting or a NVIDIA partner submission where NVIDIA provides support. Even if we call that single TPUv4 result diversity in systems being tested, still 5/8ths of the benchmarks only had NVIDIA accelerator results.
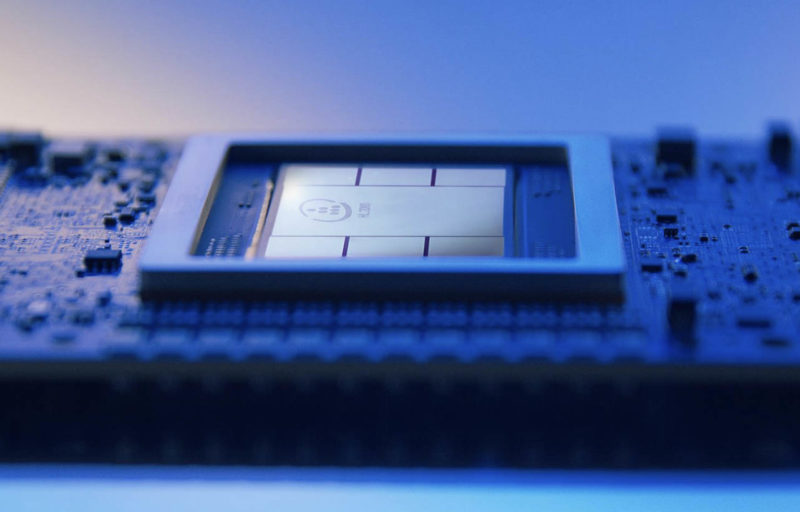
Since there is no real competition, the training exercise should just be called the “NVIDIA MLPerf Training” test. Luckily Google stepped in with something interesting along with the Intel Habana folks to make this interesting. Realistically, NVIDIA has owned AI training, for years, so that is part of it. Also, Graphcore’s poor results in MLPerf Training are probably keeping some other solutions out of the exercise. The Gaudi2 certainly looks interesting as well.
We will note that there were five submissions in the open division where systems were each tested on one of the eight benchmarks, but it is hard to use them as comparison points since there is so little there.
Well the lack of results is obviously because they loose against A100. If they reached good performance, be assured that they will all publish their achievement. Marketing 101
The sad thing is that competition still can’t totally beat a 2 years old silicon when H100 is already sampling and will probably send everybody to oblivion on next submission. Wake me up when AMD, intel and Google will be serious about AI/ML…