Today was a coordinated disclosure of new MLPerf v0.5 results. MLPerf v0.5 is the industry’s attempt at creating a “SPEC-like” industry performance benchmark to show the differences between different machine learning architectures. The entire space changes pace so rapidly that it has been hard to get a coordinated effort around performance comparisons. Now we have three teams submitting results. Coincidentally, these are companies with large software engineering teams: NVIDIA, Intel, and Google. Despite the upstarts, NVIDIA is still the leader in deep learning training hardware, and it set out to show why in its first MLPerf results.
MLPerf Overview
Here is a nice overview chart of MLPerf including what workloads it is using and the application of those workloads.
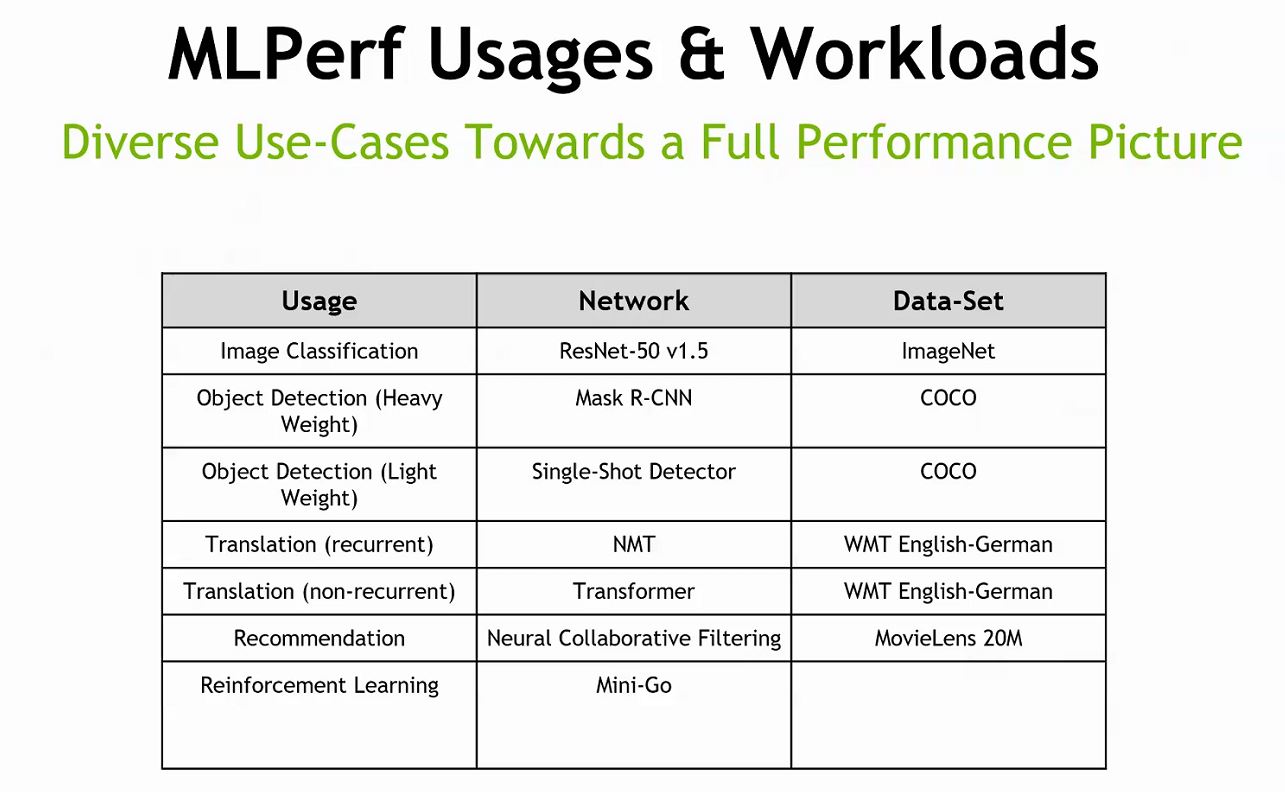
Machine learning frameworks change over time, so picking relevant benchmarks is a difficult task. STH will be adopting MLPerf in January 2019 and we already have it running on 8x GPU systems in our labs.
One really interesting note here, the reinforcement learning Mini-Go network NVIDIA did not submit on. On the pre-briefing call, NVIDIA said that it is a network that favors CPUs. A dual Intel Xeon Platinum 8180 system offered a 6.6x speedup over the NVIDIA Tesla P100 reference so NVIDIA did not think it could beat Intel here. That is interesting just because Intel is pushing the deep learning capabilities of its next-generation Cooper Lake bfloat16 server chip due in a few quarters. From what we have heard around Cooper Lake, it should outpace the current Platinum 8180 here by a substantial margin.
NVIDIA Setting Records in MLPerf with the DGX-2H
What is really interesting is that NVIDIA is using its DGX-2H platform. This is a 16x NVIDIA Tesla V100 platform that is an update to the DGX-2. We recently highlighted the NVIDIA DGX-2H at Supercomputing 2018. The footnote on the NVIDIA slides indicates a different CPU, the Intel Xeon Platinum 8189 SKU. [Update: NVIDIA sent us updated slides with different test system specs that now list the Platinum 8174 SKU in the DGX-2H as we reported previously. Intel sent us a reply on the Platinum 8189 which was essentially a “8189?” so we think this was just a typo in the original slides.] We also know that the DGX-2H uses a 450W TDP limit on its Tesla V100 32GB modules. That gives the DGX-2H a whopping 12kW maximum power consumption figure. With that large single node, here are the results:
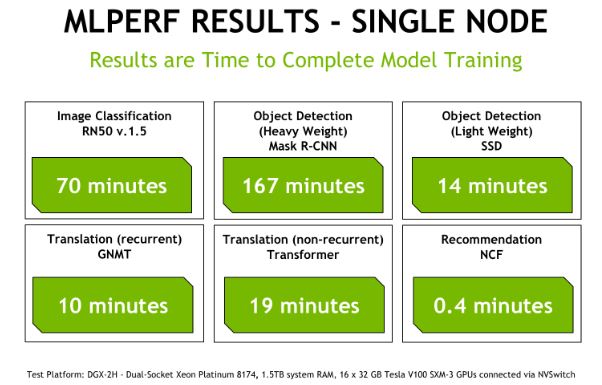
Here the results are a bit less clear. NVIDIA uses a DGX-2 cluster for the top three tests. For the bottom two tests, it is using a DGX-1V Saturn cluster from the company’s internal Top500 supercomputer. There is the same DGX-2H spec with the dual Intel Xeon Platinum 8189. [Update: see note above, this seems to have been an NVIDIA typo in the slides we were shown.] In the results, NVIDIA was binding processes to 24 cores, so it is possible that this part is a high-frequency 24-core part. We asked for clarification on this.
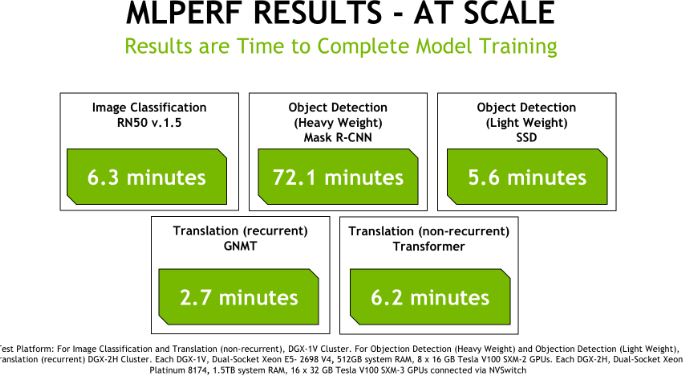
Overall, these are very fast figures. On the Image Classification test, Google had a 260 chip count TPUv3.512 + TPUv2.8 cluster that was marginally faster than 32x NVIDIA DGX-2H systems but slower than 80x DGX-1 systems. 32x DGX-2 (standard) systems are listed at around $400,000 each makes that cluster likely a $12.8M+ cluster.
Final Words
You will see more from various companies on MLPerf, and STH will be using this on our go-forward GPU system reviews. It has had a few quirks during development which is why we have not used it thus far. We thought it was interesting that Intel, NVIDIA, and Google were the only three companies to release benchmarks today in the Closed Division. Conspicuously absent was AMD as well as an enormous number of AI startups.
[Update: Since we received updated slides after the Platinum 8189 was published, we are going to save one of the original slides below. The slides in the article have been replaced with their updated versions.]
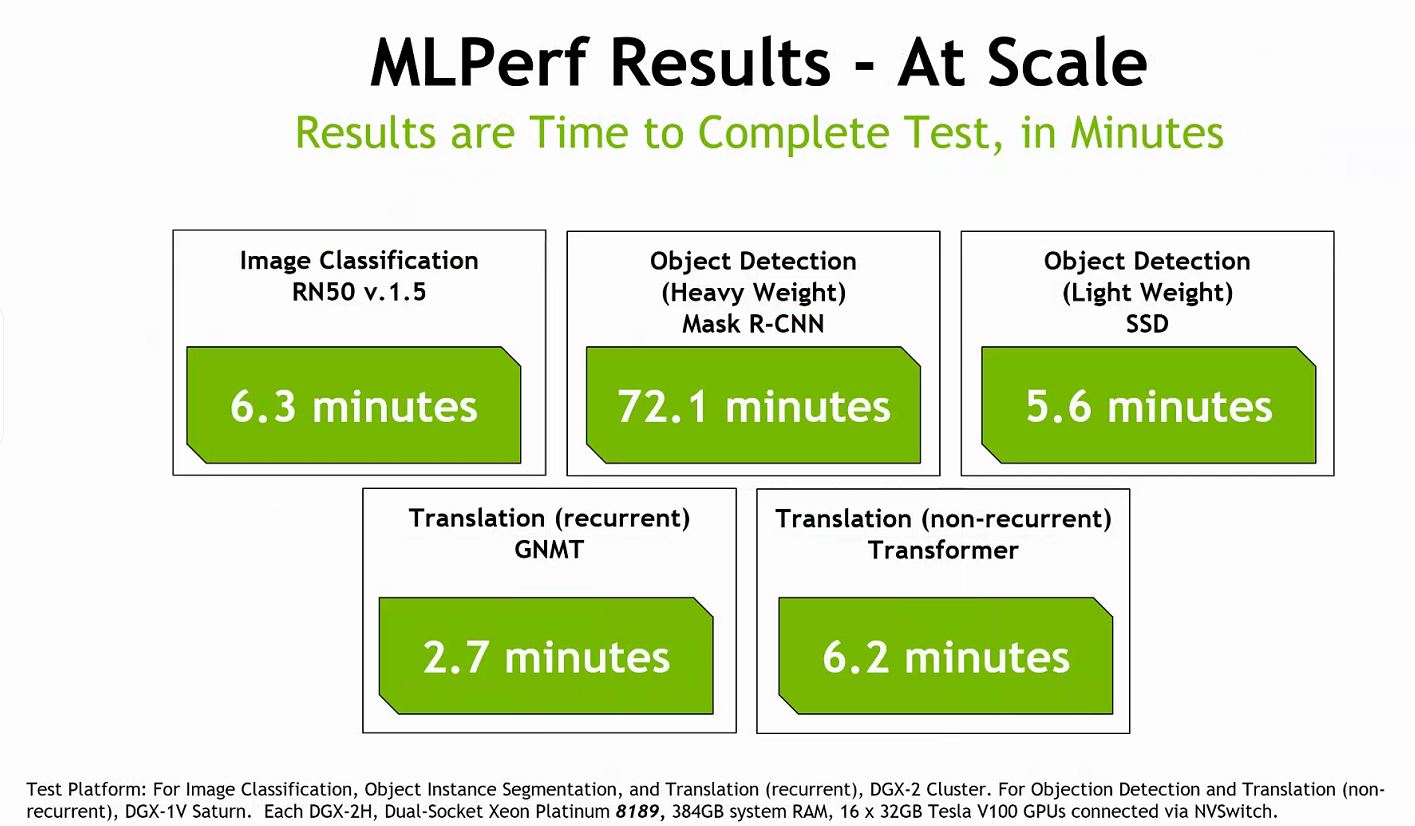