At Supercomputing 2018, STH saw the QNAP TS-2888X which is a fascinating machine. It is an amalgamation of a workstation, deep learning server, and NAS in a single system. The QNAP unit combines a multi-GPU capable Intel Xeon W platform with a 28 bay NAS. The NAS supports up to four double-width GPUs, dual 10Gbase-T, a closed-loop liquid cooler, 8x 3.5″, 16x 2.5″ SATA, and 4x U.2 NVMe slots. There is a lot going on with this server, and we think it may be close to a category-defining product.
QNAP TS-2888X Overview
The front of the QNAP TS-2888X has USB ports as well as a LCD screen with basic status information. The main part of the front is a giant vent with a fan well behind it. This unit is on wheels to make it easy to move.
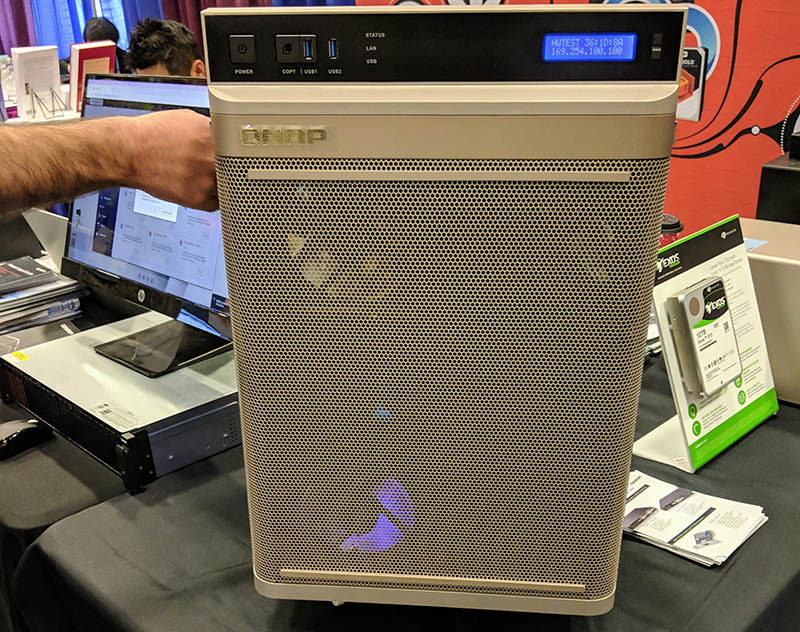
The rear of the unit has a 2kW PSU. Note, this is more than the average North American 15A/ 120V office or home circuit will support, so you will likely need a specific circuit to run the pedestal server. There are additional fans for cooling and spaces for four dual-width GPUs including extra space. Quad 1GbE and dual 10Gbase-T LAN ports provide networking. You will need to lose a GPU slot if you want high-speed networking for the QNAP TS-2888X.
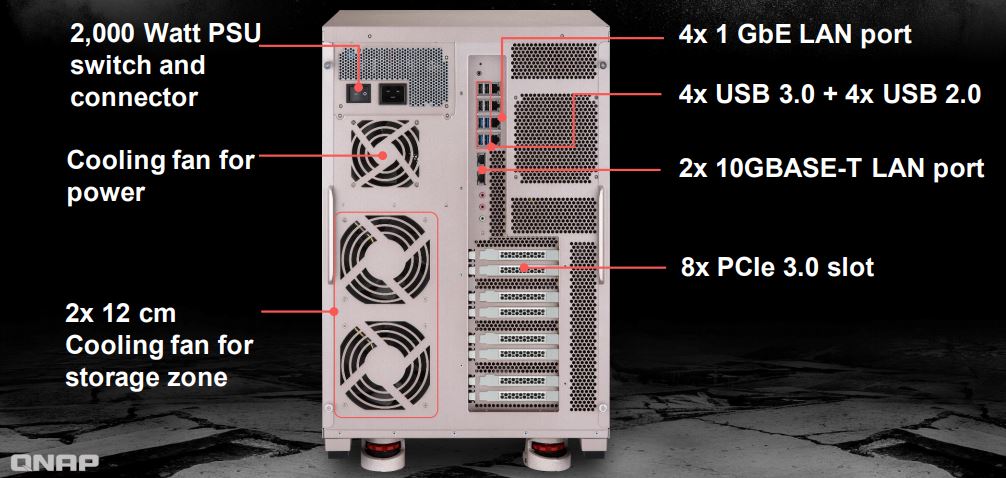
The rear of the unit is the NAS part. There are eight 3.5″ SATA bays for bulk storage. 16x 2.5″ SATA bays are for SSD storage, and there are 4x 2.5″ U.2 SSD ports as well. As we move to 2019, SATA for SSDs makes little sense. We wish QNAP had a revised design with more 3.5″ bays.

Inside you can see the four GPU double-width slots with extra gaps to use blower-style active vards.

One can also see the closed loop liquid cooling system for the Intel Xeon W processor and eight DDR4 DIMM slots.
QNAP TS-2888X Block Diagram
We wanted to take a quick moment to discuss the block diagram. This is a discussion less technical sites will overlook in discussing the TS-2888X, but it is absolutely essential to any deep learning system.

With 24x PCIe 3.0 from the CPU and 8x PCIe lanes from the PCH, there are a total of 32x PCIe lanes available. Typically, you would not put GPUs attached to the PCH. QNAP puts a SATA controller and the four NVMe U.2 drive bays off of the CPU lanes. The Intel Xeon W is a good platform, but it is more appropriate for 2-3 GPUs if one wants maximum GPU performance for training. Adding a PCIe switch can help, however, we generally see two 48 port PCIe switches in single CPU designs, where each PCIe switch leads to two GPUs. An example of this can be seen in our Tyan Thunder HX GA88-B5631 Server Review. For a hybrid GPU and storage server, this is certainly one architecture to use, but we can also see an AMD EPYC or dual Intel Xeon Scalable system is what some of our readers prefer.
From Patrick our Editor-in-Chief on the QNAP TS-2888X:
The QNAP TS-2888X is a greatly refined solution compared to some of the prototypes we saw at Computex 2018. It is great to see that the company iterated on the product since we first saw it. For some, this is going to be the system they have been waiting for.
At the same time, this is a perfect example of a system that, given its limitations, should have been designed as an AMD EPYC 1P system. As we covered in AMD EPYC’s Extraordinarily Aggressive Single Socket Mainstream Pricing, QNAP could have provided the PCIe lanes for more NVMe storage in this configuration. The company could have also provided greatly expanded memory per socket and a 32-core AMD EPYC 7551P processor for less than its 18 core Intel Xeon W. The system is well concieved but could benefit from using a more appropriate platform.
QNAP TS-2888X Software
QNAP is making an interesting play for the edge AI space. QNAP has its own operating system and has been adding AI applications to its ecosystem using containers. Here is a shot of the system running its AI containers at SC18.
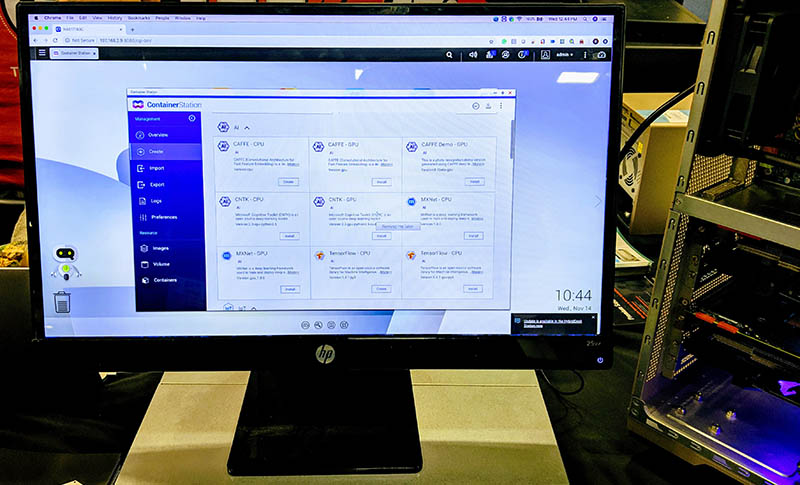
This provides a relatively easy GUI for those who are technical enough to want a large storage array and do not want to take on the technical complexity of using other containers.
Final Words
The Intel Xeon W is more powerful than the average QNAP appliance CPU. It is great to see QNAP embrace the larger CPU platforms as it fills out its range. If you are looking for pure GPU performance, you will want a different PCIe topology. What QNAP offers in the TS-2888X is a unique blend of a storage server with a GPU server. The one item that will give you pause is the price.
Even with the November “pre-order” discount, the higher-end configurations are priced at a steep premium. An Intel Xeon W-2195 lists at $2553 and 8x 64GB RAM lists for around $5300 which puts the $23100 (pre-order) and $27199 top-bin system (TS-2888X-W2195-512G) at a $15,000-$19000 premium for everything other than RAM and CPU. That is about the largest premium you will see for barebones on the market, making it more costly than a DeepLearning11 style 10x GPU system with more networking, redundant power supplies albeit less storage. It is also more costly than the Gigabyte G481-S80 barebones we reviewed. We think QNAP needs to align its pricing with market expectations or else we would simply recommend our readers purchase the base TS-2888X-W2123-32G at $4900 and upgrade themselves, saving ~$10000 in the process. Even at that price, the premium is significant.
This is an extremely interesting unit. At the same time, QNAP is asking for an enormous premium for a server with limited connectivity. A revised QNAP TS-2888X with revised pricing in the 20% gross margin range and sporting AMD EPYC would be a game-changing solution in this space.
https://www.ieiworld.com/en/product/model.php?II=639
https://www.ieiworld.com/en/product/model.php?II=639
iei
GRAND-C422-20D
The GRAND-C422-20D is an AI training system with Intel® Xeon® W family processor and powerful PCIe expansion ability