MLPerf Training v3.1 results were out, and it was probably one of the least exciting rounds we have seen in some time, except for two results. First, NVIDIA is using MLPerf to show off its latest Hopper-generation supercomputer. Second, in an uncommon occurrence, both Intel and NVIDIA trumpeted the Intel Gaudi 2 accelerator’s excellent price/ performance.
Intel Scores a Huge Gaudi2 Win in NVIDIA MLPerf Training v3.1
There were 209 results run across 83 different configurations between the Closed division and Open. Open only had two configurations submitted, each with a single but different result. MLPerf Training v3.1 has nine tests, so the average completion ratio of the benchmark suite is only around 27% for each submitted configuration. Only 14 of 209 results were anything other than NVIDIA GPUs. Six of those were Intel showing off Intel Xeon Sapphire Rapids performance in AI, setting a baseline for CPU performance in the industry.
The first big takeaway is that NVIDIA is showing off its new supercomputer. Dubbed NVIDIA Eos, this is a 10,752 H100 GPU system connected via 400Gbps Quantum-2 InfiniBand. Putting this into some perspective, if a company were to buy this on the open market, it would likely be a $400M+ USD system.
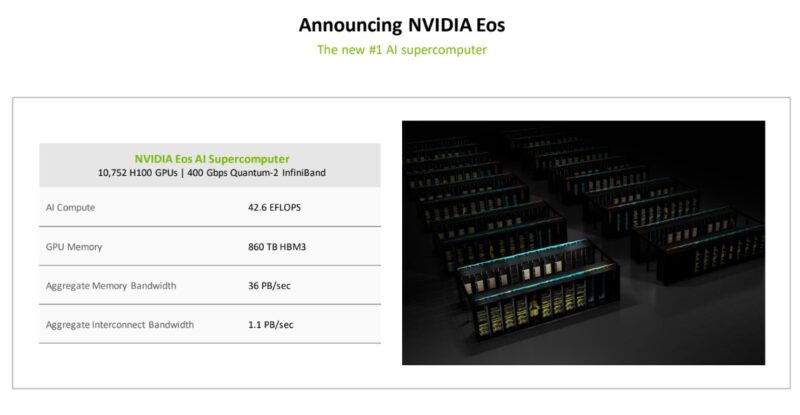
With its giant supercomputer, NVIDIA can win on the MLPerf Training suite across varying GPU numbers. It even matched the Microsoft Azure cloud running at 10,752 NVIDIA H100 GPUs.
In general, so few other companies are submitting results to MLPerf Training that NVIDIA had to make up a summary slide showing that very few others are using its MLPerf Training suite. We know from AMD earnings that it is shipping MI300 variants, which would be “commercially available” for MLPerf. We also know that companies like Cerebras sold a $1B(+) Wafer-Scale Cluster without MLPerf. MLPerf Inference is often fascinating for vendor-to-vendor comparisons. MLPerf Training has lost that, except for one instance.
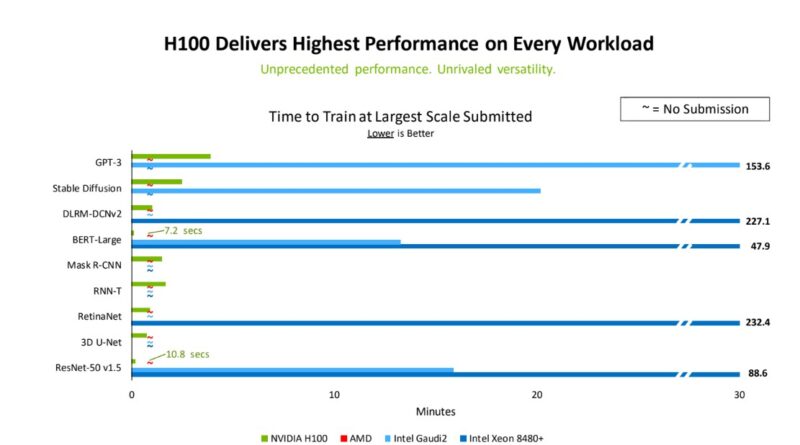
Looking at the Stable Diffusion test above, one might see NVIDIA as being around 8x faster than Intel Gaudi2. Then we get to the details. NVIDIA uses 16 times as many H100 GPUs to get that kind of speedup. Putting that into perspective, we would expect that it is well over 32x the cost to go 8x faster. Put another way, NVIDIA’s marketing slide here shows Intel is somewhere between relatively close and 4x better performance per dollar versus NVIDIA (~8x the performance for ~32x the cost.)
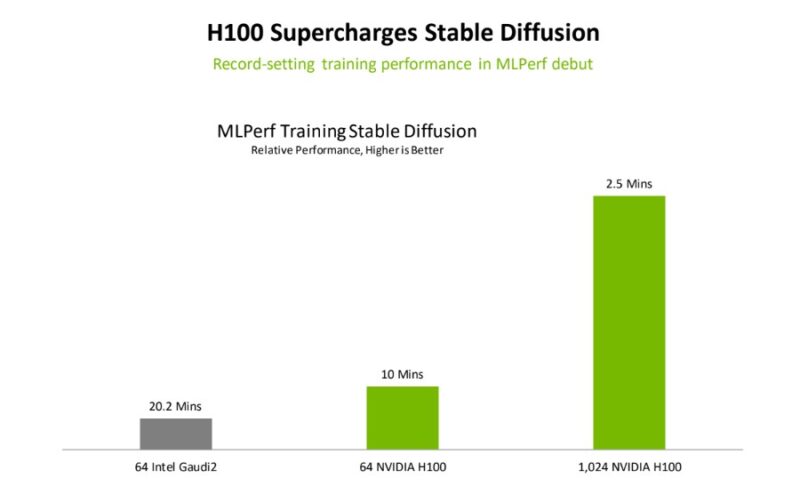
In a direct comparison with 64 accelerators, NVIDIA is around twice as fast. That is an excellent result for Intel since it uses accelerators that cost less than half its NVIDIA counterparts and has a much simpler system architecture.
NVIDIA is further along tuning its H100 than Intel is with Gaudi2, but the big delivery with this MLPerf was supporting FP8. That led to a ~2x speedup in performance.
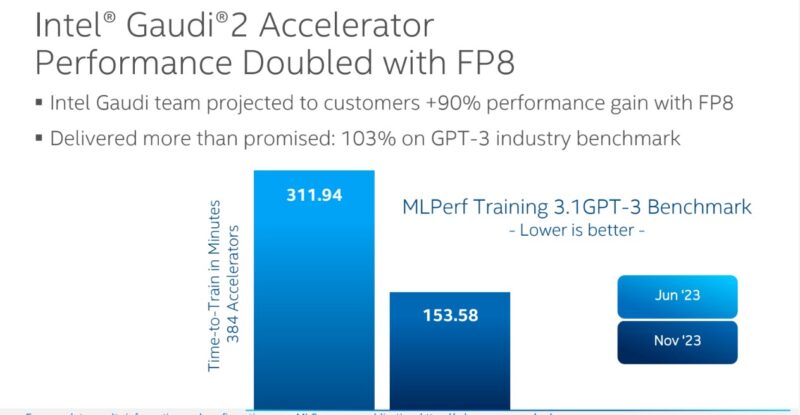
Intel now has an exciting play. The Gaudi2 is seemingly somewhere between A100 and H100 performance. Still, from what we understand, it costs less than half of NVIDIA’s H100 part on an accelerator-to-accelerator basis but can be much lower in total system costs.
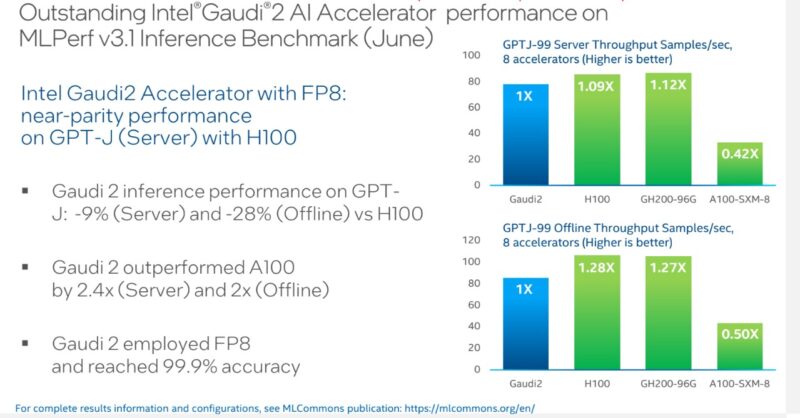
Last week, we looked at some Gaudi2 systems in our Inside the Intel Developer Cloud tour:
Intel Gaudi2 is getting enough traction that it is supply-constrained, but less so than the H100.
We are going to give a special mention to the NVIDIA L40S that made a single appearance in this set. We just went into why the L40S is becoming an alternative where one can use more L40S GPUs given their cost and availability advantage over the H100. Check out the NVIDIA L40S vs H100 PCIe piece here. The MLPerf Results seemed to track that story.
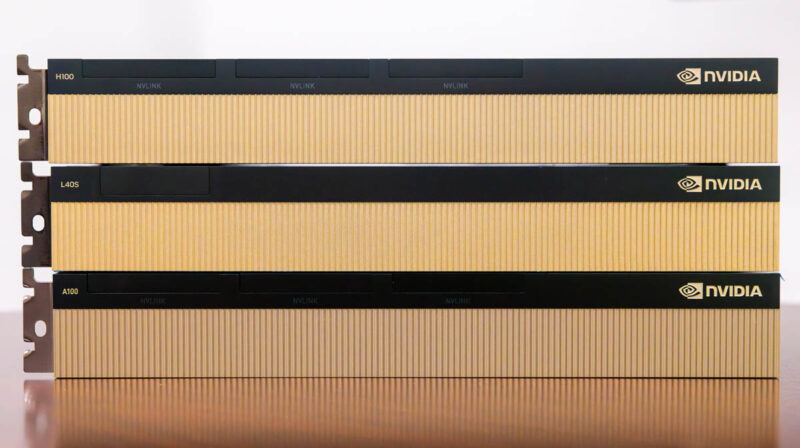
An honorable mention also goes to Google for its TPUv5e submission. There is not much to compare it to directly, given it is cloud-only, but at least they submitted something.
Final Words
It feels like most of the industry other than NVIDIA has abandoned MLPerf Training, save for a few Intel results and a lone Google result. The NVIDIA H100 SXM5 is the big GPU today, and few use MLPerf to challenge that. There are only a handful of companies that can scale their hardware and software past 1000 accelerators, so at the top end, there is not a lot of competition. If you want 10,000 accelerators six months from now, most likely, you are buying a NVIDIA H100 SXM5 system.
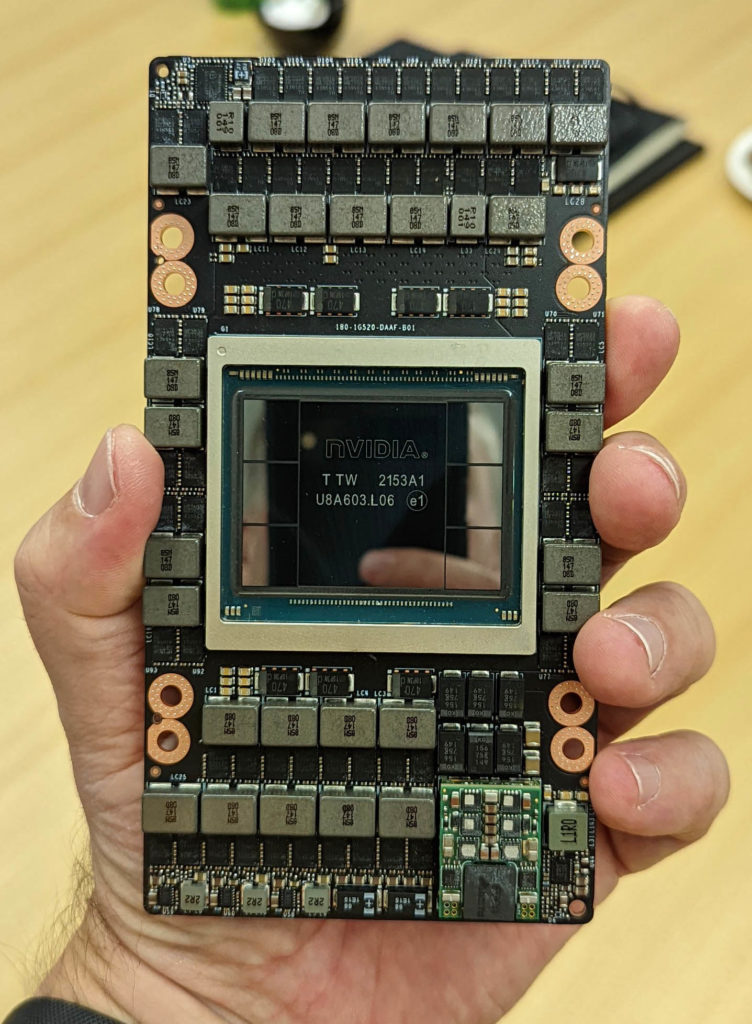
With the NVIDIA Eos supercomputer, NVIDIA has something in-house that would have a retail value of over $400M. Perhaps no other company in the industry can afford to match that at this point. Of course, that is retail value, not what NVIDIA is paying for its own parts, but it is a big machine.
A fun result of NVIDIA’s race to scale is that it showed Intel’s Gaudi 2 can deliver stable diffusion performance at four times the performance per dollar. NVIDIA was trying to show it had a much longer bar on the chart, but in the race for a longer bar, it lost scaling efficiency and gave Intel a big win.
> Looking at the Stable Diffusion test above, one might see NVIDIA as being around 8x faster than Intel Gaudi2. Then we get to the details. NVIDIA uses 16 times as many H100 GPUs to get that kind of speedup. Putting that into perspective, we would expect that it is well over 32x the cost to go 8x faster. Put another way, NVIDIA’s marketing slide here shows Intel is somewhere between relatively close and 4x better performance per dollar versus NVIDIA (~8x the performance for ~32x the cost.)
Uh? We mainly see that training Stable Diffusion is not linearly scalable: there’s a 4x speedup (from 10 min to 2.5 min) for a 16x scaling in number of GPUs (from 64 for 1,024 H100s).
But in terms of comparison with Gaudi2, that slide shows:
* 64 Gaudi2 : 20.2 min
* 64 H100: 10 min
So even considering H100s are twice the price of Gaudi2, it puts the performance/price of each accelerator in the same range: H100s are training Stable Diffusion twice as fast as Gaudi2, for twice the price. Same perf/price ratio here.
So, the 4x in this headline is about software scalability, not about accelerator performance/dollar ratios. This is quite a misleading takeaway from that slide, to say the least.
Odd thing though is I feel guadi2 would be rather niche for colocation or on premise.
While I’m rather familiar with ML, I am not exactly an expert at how cloud services maximize their profit but I feel renting out H100s at the price point they do would still be ideal for the consumer and business.
– For business it’s less space/infrastructure if compared a 1to1.
– For the consumer it’s the same or less total cost due to per hour resource consumption.
It’s possible guadi may be more ideal for prod deployment of non massive models but who knows.
In some cases twice time for half price give 0 profit to the conpany. Or might disable a research initiative.
Example: reinforcement learning for market impact of high frequency futurs trading.
If you delay by 400 milliseconds instead of 200 yor loss due to miss forcasting market impact may go from 20% to 50%
Looking at how Gaudi2 performs in BERT (MLPerf Training v3.1) and GPTJ (MLPerf Inference v3.1), it seems like for the same datatype (e.g. FP8 or BF16), Gaudi2 is within 20% of H100.
But for GPT3 model, it is 2x slower than H100. Which is puzzling because they are all similar at the fundamental level — i.e. all built using transformers.
I suspect it’s because to run GPT3 you need to have some form of model level parallelism (e.g. tensor or pipeline), which demands much higher inter-GPU bandwidth. The 8-Gaudi2s in a node are all connected in an all-all fashion, which is not super high bandwidth. Which is why Nvidia connects their 8-H100s in a DGX node using NVSwitches.
If Gaudi2 are connected (inside a node) using a standard high speed Ethernet switch instead of all-2-all, I think Gaudi2’s performance for GPT3 training will dramatically improve.
Patrick, can you please check with your Intel/Habana contacts if this makes sense and/or whether they’ve considered it?
Who cares performance per dollar !!!
Maybe in Africa