MLPerf Inferencing v0.5 results are now online. Many companies are claiming victories today. We wanted to provide some context for our readers, then provide some of the results we saw hit the wire. Perhaps one of the geekiest parts about the new benchmark is that the MLPerf team can have hundreds of results submitted from many different vendors and yet, we are still in a pre v1.0 world. It reminds me of a software company I worked with that had a 5-year-old product that never seemed to hit V1.
MLPerf Inferencing v0.5: A Quick Overview
The MLPerf Inferencing v0.5 has and open and closed division. The easiest way to think about this is that the closed division is designed to allow cross-vendor comparisons by more tightly controlling how vendors run the benchmark such as using a specific model. Open division results are designed to be the wild-west where teams have more flexibility to show off what they can do. In terms of the popularity, with v0.5 we see 169 closed division results and 428 open division for a total of 597 results from 14 organizations.
There are five benchmarks for v0.5 two image classification, two object detection, and one machine translation. Here is the official chart:
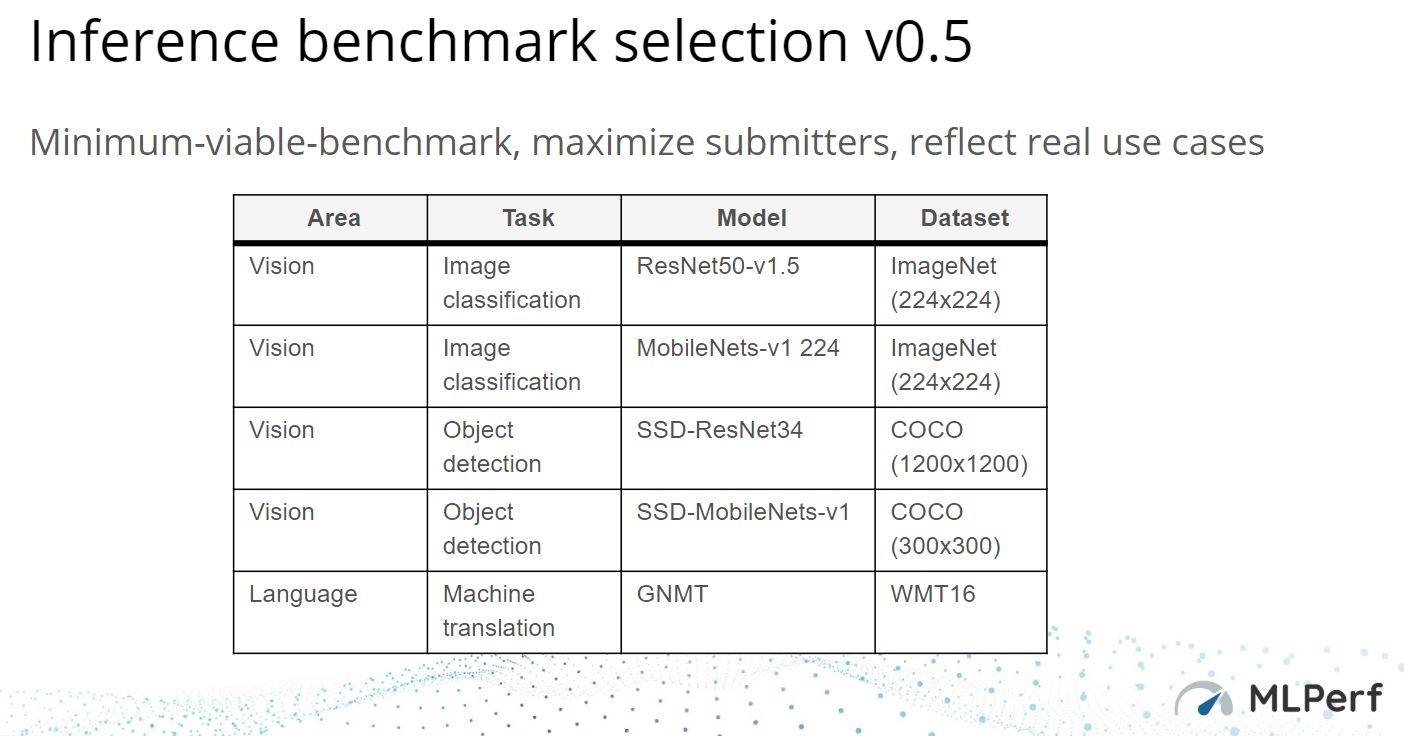
For a sense of scale, here is how often each was used. ResNet50-V1.5 was the most common model while GNMT was the least used.
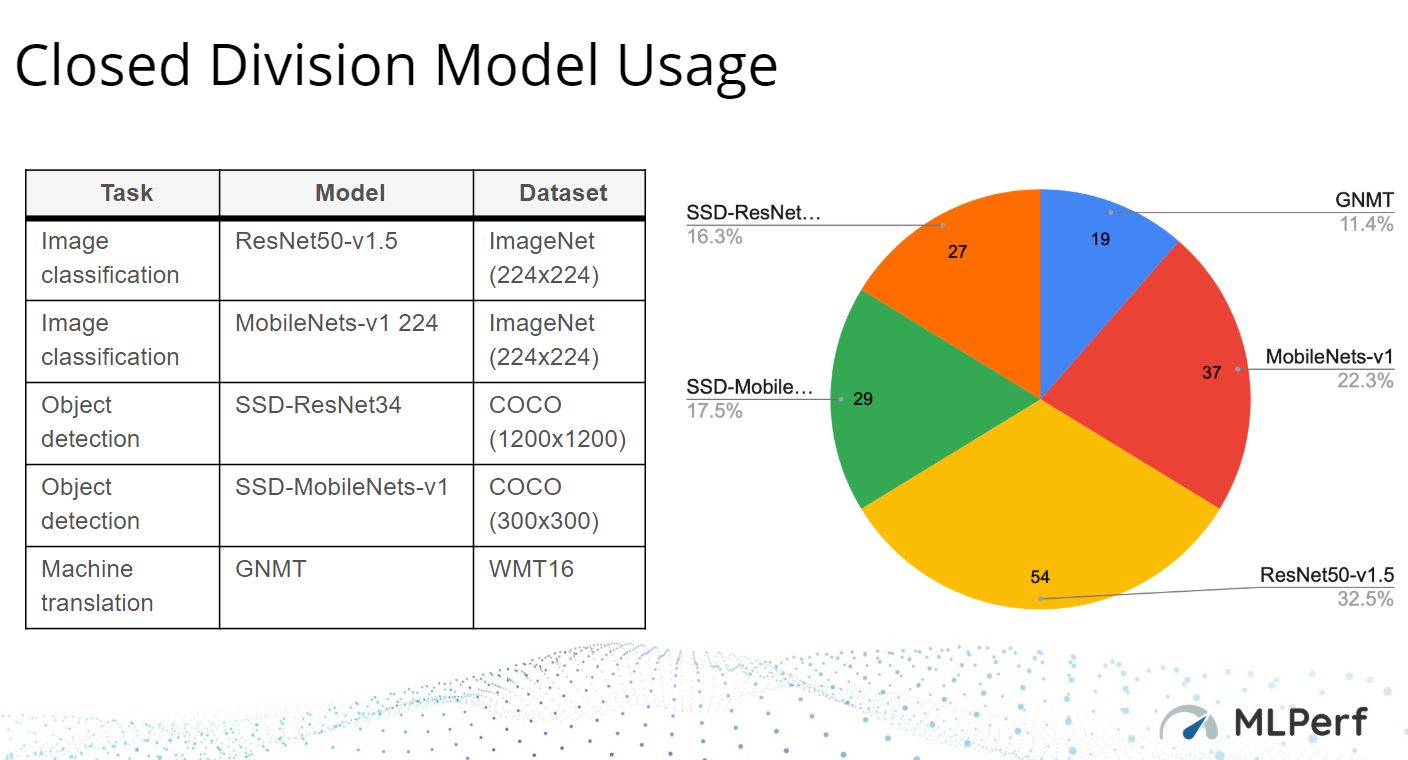
Since vendors are free to submit results for any of the models, we can see that some architectures favored some models. For example, GPUs and ASICs dominated the GNMT and SSD-ResNet34 results which are also part of the reason we see those as the two least frequently used models.
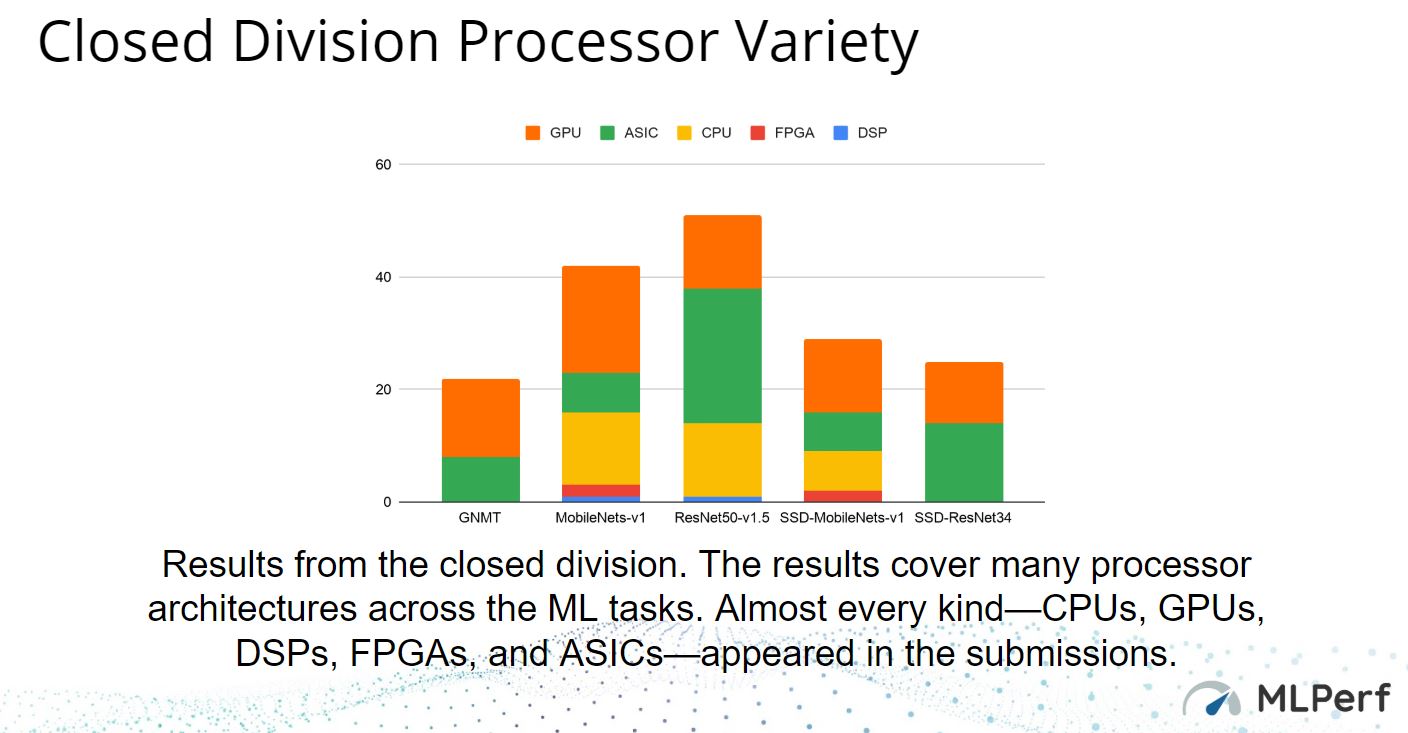
There are also four different metrics that solutions can focus on. Here the important aspect is each scenario and metric pairing is designed to align to a way we can see near-term inferencing being used.
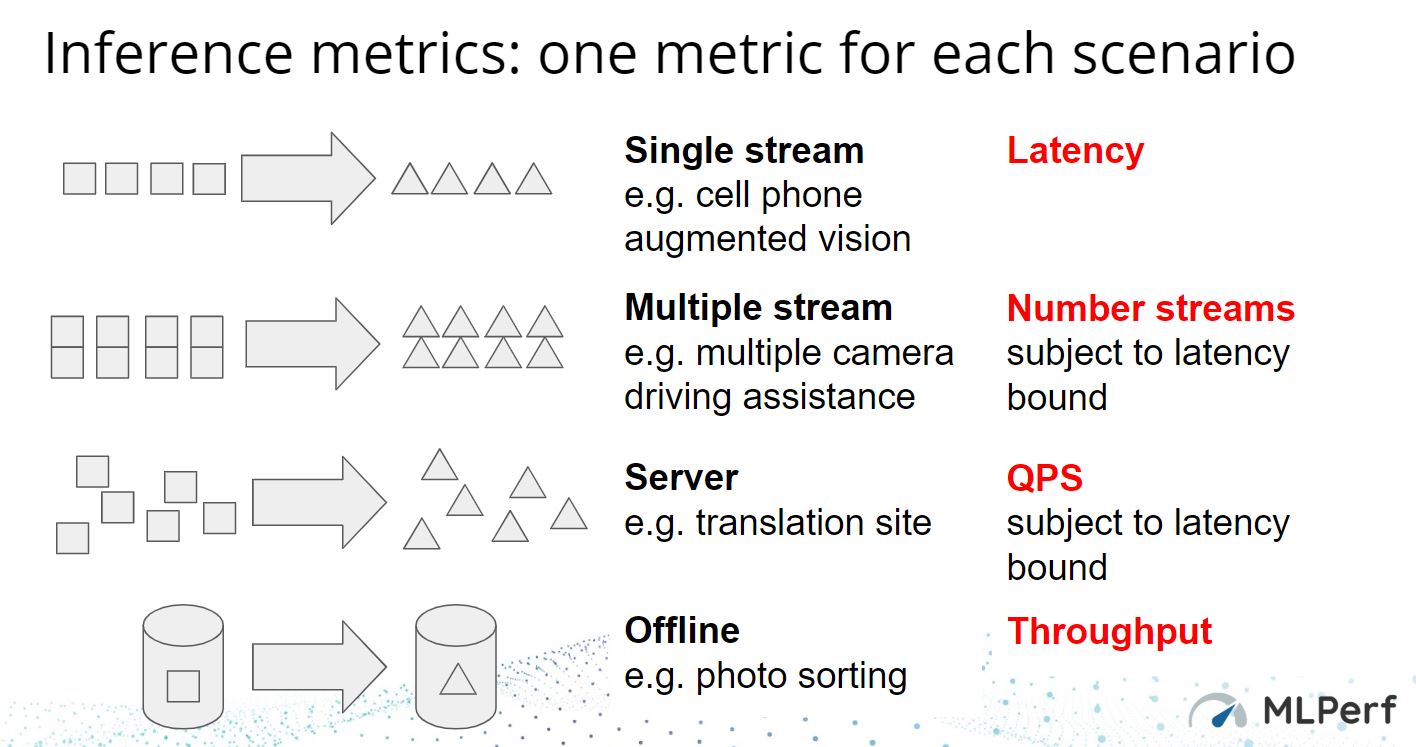
What you will see, given the breadth of where inferencing is being used, is that some vendors have optimized on specific benchmarks and metrics.
In the end, the closed division has a fairly wide performance range on some tests while others remain very close. Here is what the range for the 149 closed division results is:
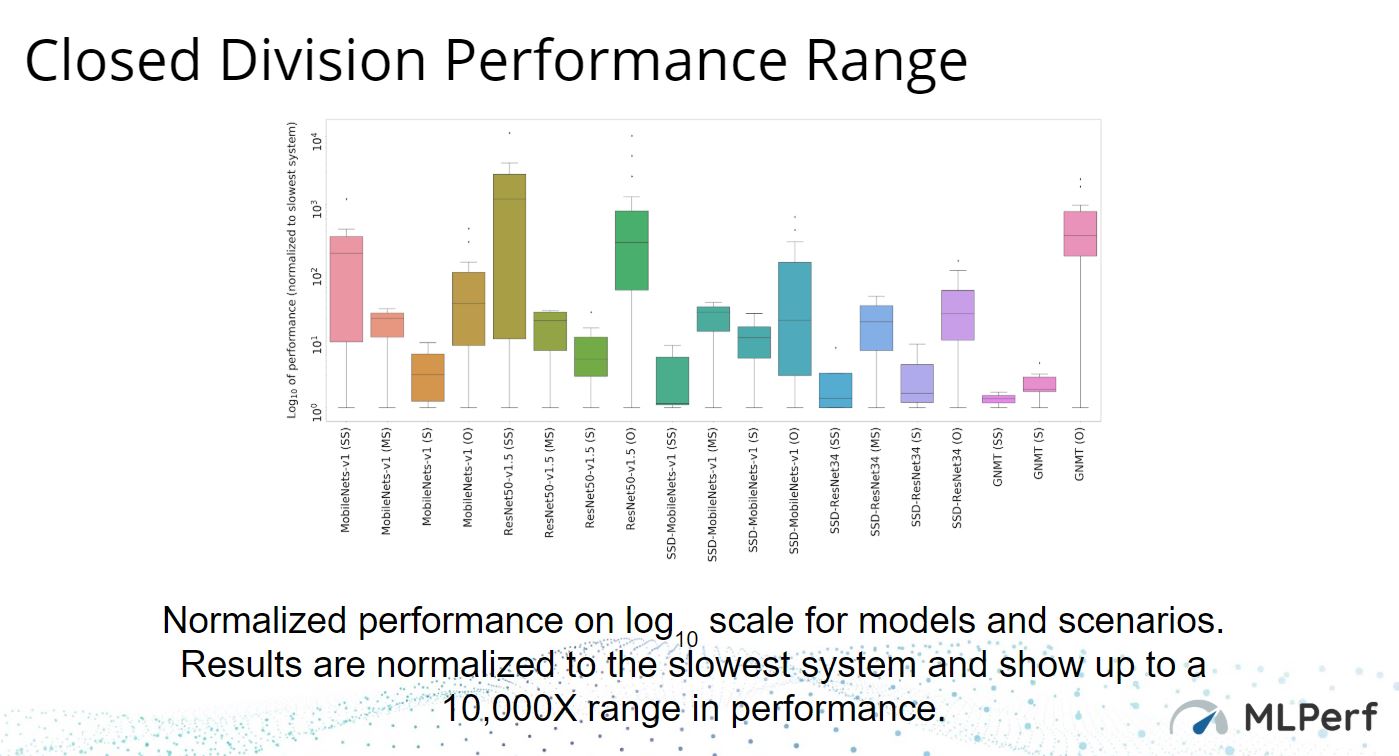
This is still an optional submittal closed division so we are seeing a lot of “cherry-picking” submitted results.
We pulled out the handsets and embedded boards, and here is a set of results that most closely mirrors what STH covers.
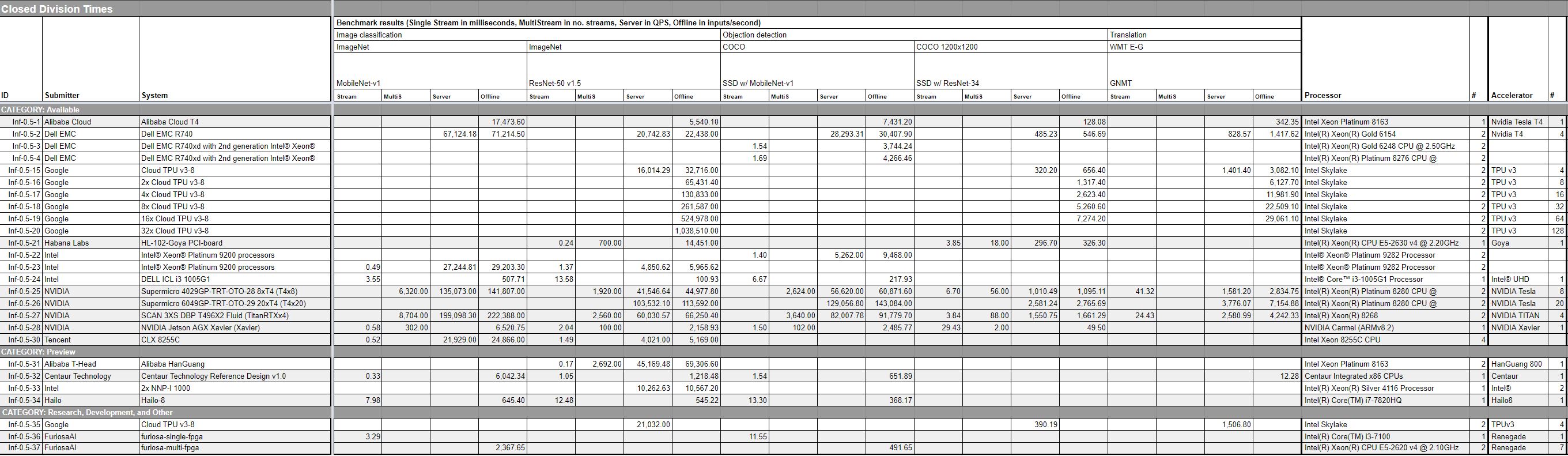
You can click on that image and get to a larger source image.
Intel MLPerf Inferencing v0.5 Results
Intel focused on using the Intel Nervana NNP-I, the company’s inferencing accelerator. Beyond that, Intel showed off what its DL Boost or Vector Neural Network Instructions (VNNI) can do both on the server side, as well as on the client side. Specifically, the company showed off results from its 2nd Gen Intel Xeon Scalable series and its Core i3-1005G1.

Intel submitted results in the closed division but focused on two sets of results: Closed ResNet-v1.5 Offline and Server and Closed SSD-Mobilenet-v1 Offline and Server. The company did not highlight other benchmarks.
As a quick note here, DL Boost does not need to be the fastest, and it is great that Intel is including this in their CPUs. Realistically, so much workload happens on Intel CPUs, having VNNI available to developers means that the bar gets raised for “zero additional cost” AI inferencing. By 2020, this will essentially be the baseline for inferencing that all accelerators need to prove they are better than. Again, a great job by Intel to journey down this path.
NVIDIA MLPerf Inferencing v0.5 Results
NVIDIA showed off its results and specifically bifurcated the results into the data center and edge / mobile sets.
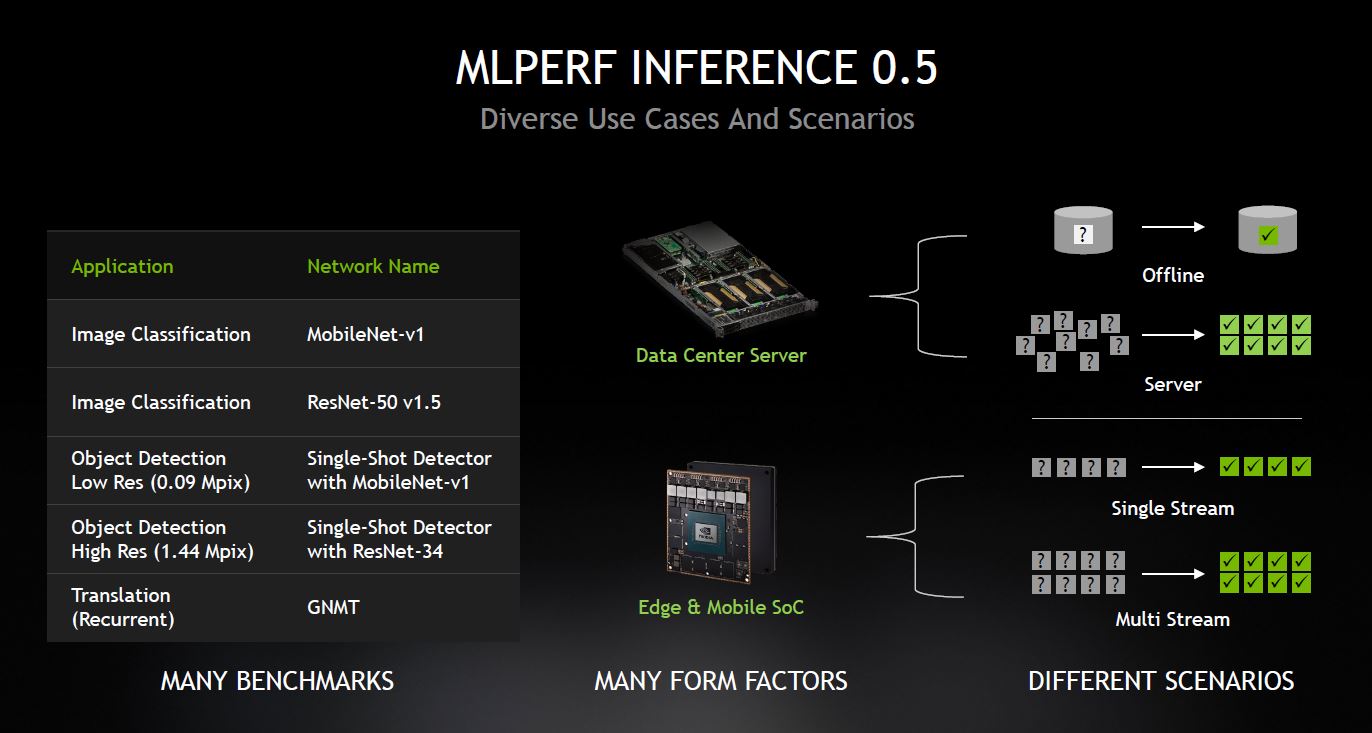
A quick note here is that the Offline and Server scenarios that Intel submitted on and is highlighting are being called data center server scenarios here by NVIDIA.
For the edge and moble results, one can see that NVIDIA is doing well. Perhaps the biggest competitor at this point is the Habana Goya that we covered in Favored at Facebook Habana Labs Eyes AI Training and Inferencing.
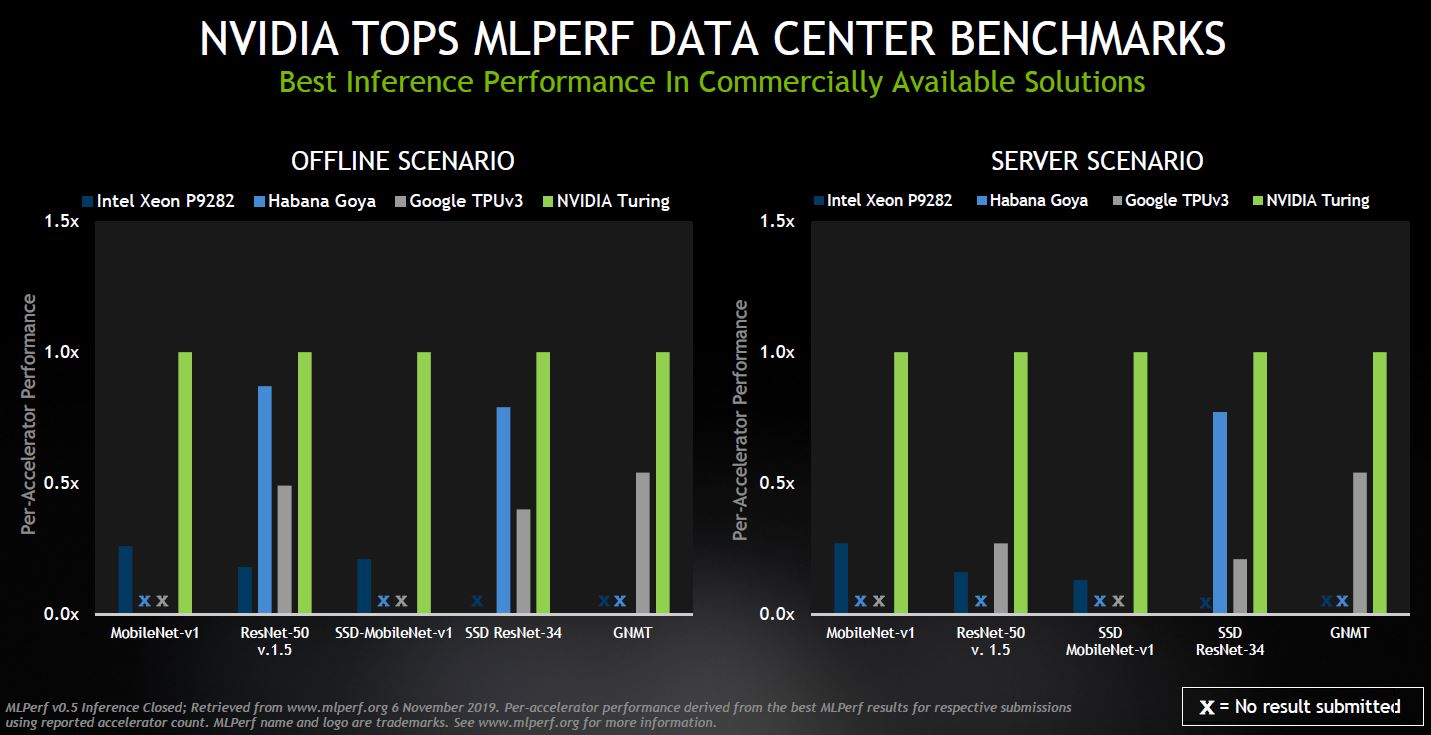
On the edge, NVIDIA is happy to show the performance of its chips versus Qualcomm and Intel.
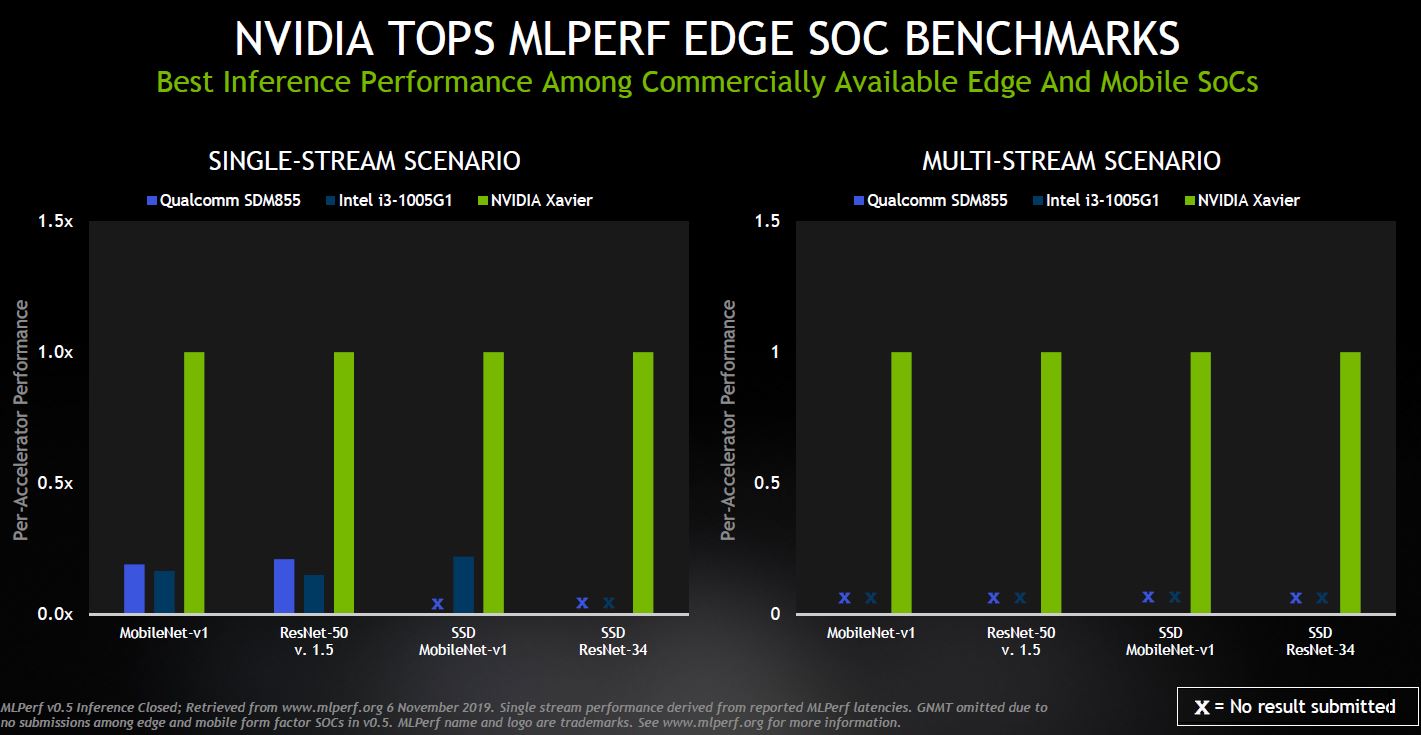
Whenever MLPerf results come out, we expect NVIDIA to do well in the closed categories and they have a story here.
One really notable finding was what happened when we found a 4x Titan RTX result versus an 8x Tesla T4 result. Here are the Offline and Server submissions with the 8x Tesla T4 result being 100%.
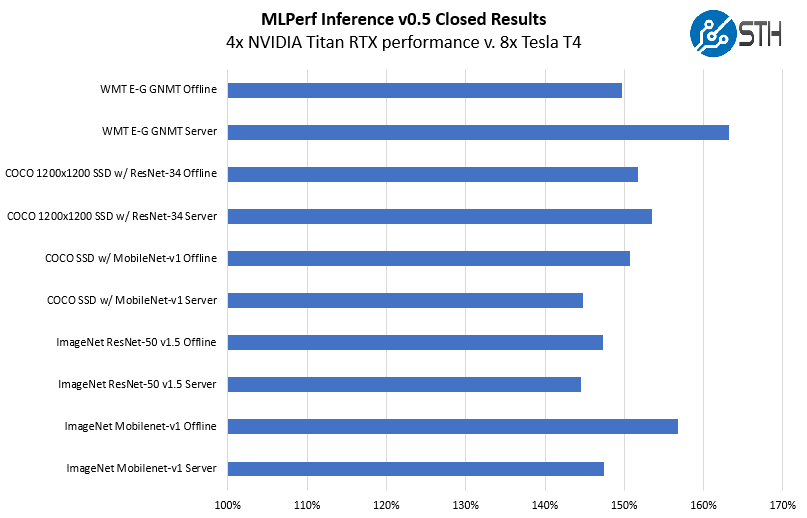
With these results, NVIDIA seems to be indicating that 4x Titan RTX GPUs are equivalent to around 12x Tesla T4’s. If you read our NVIDIA Tesla T4 AI Inferencing GPU Benchmarks and Review and our Analysis of Our NVIDIA Tesla T4 Review, that is similar to what we observed.
Final Words
Overall, this is a great first submission. Still, with five tests and four different scenarios, one gets 20 different categories for the 149 submissions to fall into. These can be further bifurcated into at least four markets such as data center or mobile which makes this almost an “everybody wins” scenario with at least 80 categories and 149 submissions. Hopefully, in the future, this gets augmented with power consumption categories as well and more vendors submit to the project.
Something that caught my eye was down in the preview section… Centaur is in the list!
For those who don’t know, Centaur Technology is part of VIA – the 3rd x86 & AMD64 license holder, who has a joint venture named Zhaoxin.
Their current batch of “available” (if you happen to be in china at least) CPUs (KX6000 from Zhaoxin) is still largely based on their older architectures, just ported over to TSMC 16nm, but allegedly the KX7000 series uses a much more modern architecture and be on TSMC 7nm. Zhaoxin was saying it would be a Zen2/Icelake competitor in terms of performance and IPC, but as far as leaked performance data, I only saw some forum posts referring to removed leaks that allegedly put its IPC as being somewhere around ivybridge-haswell. Of course theres plenty of time for that very early silicon to be improved, so we’ll see what it turns out to be like once it lands.