NVIDIA Quadro RTX 6000 Deep Learning benchmarks
Before we begin, we wanted to note that the latest NVIDIA Docker Containers had just dropped a few days ago, and there were changes to how these benchmarks run. We expect more Docker Container changes to come in, so please use our numbers presented as a reference.
ResNet-50 Inferencing in TensorRT using Tensor Cores
ImageNet is an image classification database launched in 2007 designed for use in visual object recognition research. Organized by the WordNet hierarchy, hundreds of image examples represent each node (or category of specific nouns).
In our benchmarks for Inferencing, a ResNet50 Model trained in Caffe will be run using the command line as follows.
nvidia-docker run --shm-size=1g --ipc=host --ulimit memlock=-1 --ulimit stack=67108864 --rm -v ~/Downloads/models/:/models -w /opt/tensorrt/bin nvcr.io/nvidia/tensorrt:20.11-py3 trtexec --deploy=/models/ResNet-50-deploy.prototxt --model=/models/ResNet-50-model.caffemodel --output=prob --batch=16 --iterations=500 --fp16
Options are:
–deploy: Path to the Caffe deploy (.prototxt) file used for training the model
–model: Path to the model (.caffemodel)
–output: Output blob name
–batch: Batch size to use for inferencing
–iterations: The number of iterations to run
–int8: Use INT8 precision
–fp16: Use FP16 precision (for Volta or Turing GPUs), no specification will equal FP32
We can change the batch size to 16, 32, 64, 128 and precision to INT8, FP16, and FP32.
The results are Inference Latency (in sec).
If we take the batch size / Latency, that will equal the Throughput (images/sec) which we plot on our charts.
We also found that this benchmark does not use two GPUs; it only runs on a single GPU.
You can, however, run different instances on each GPU using commands like.
“`NV_GPUS=0 nvidia-docker run … &
NV_GPUS=1 nvidia-docker run … &“`
With these commands, a user can scale workloads across many GPUs.
Also one can use the —device=0,1,2,3,4,… a command to select which GPU to run on, more on this later.
We start with INT8 mode.
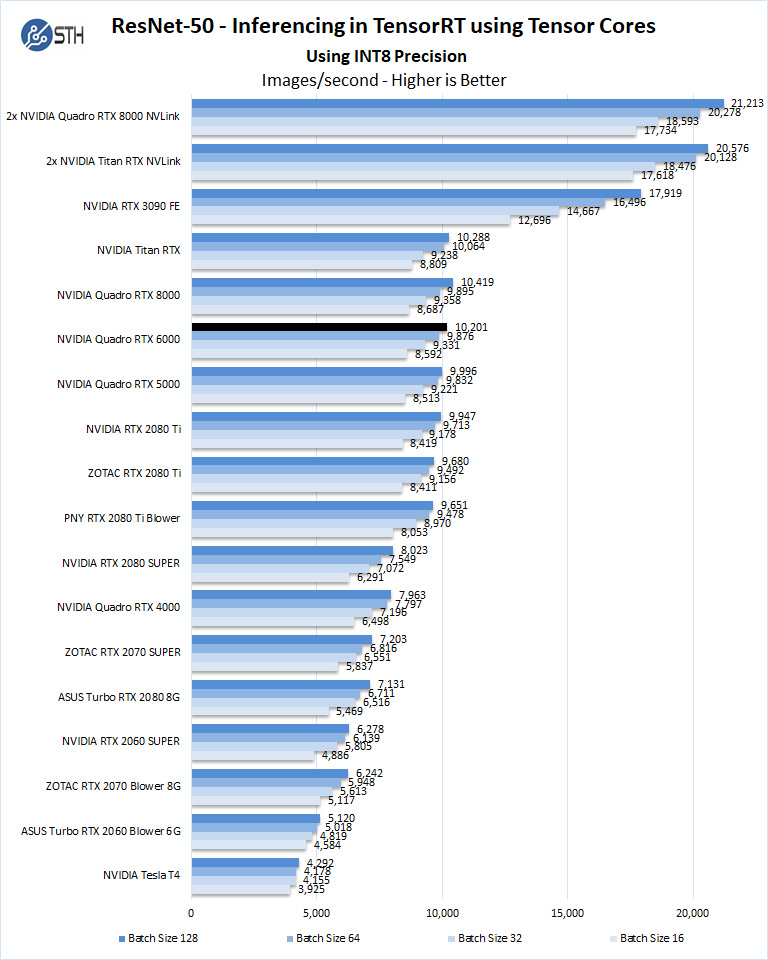
Using the precision of INT8 is by far the fastest inferencing method if at all possible, converting code to INT8 will yield faster runs. Installed memory has one of the most significant impacts on these benchmarks. Inferencing on NVIDIA RTX graphics cards does not tax the GPU’s to a great deal. However, additional memory allows for larger batch sizes.
Let us look at FP16 and FP32 results.
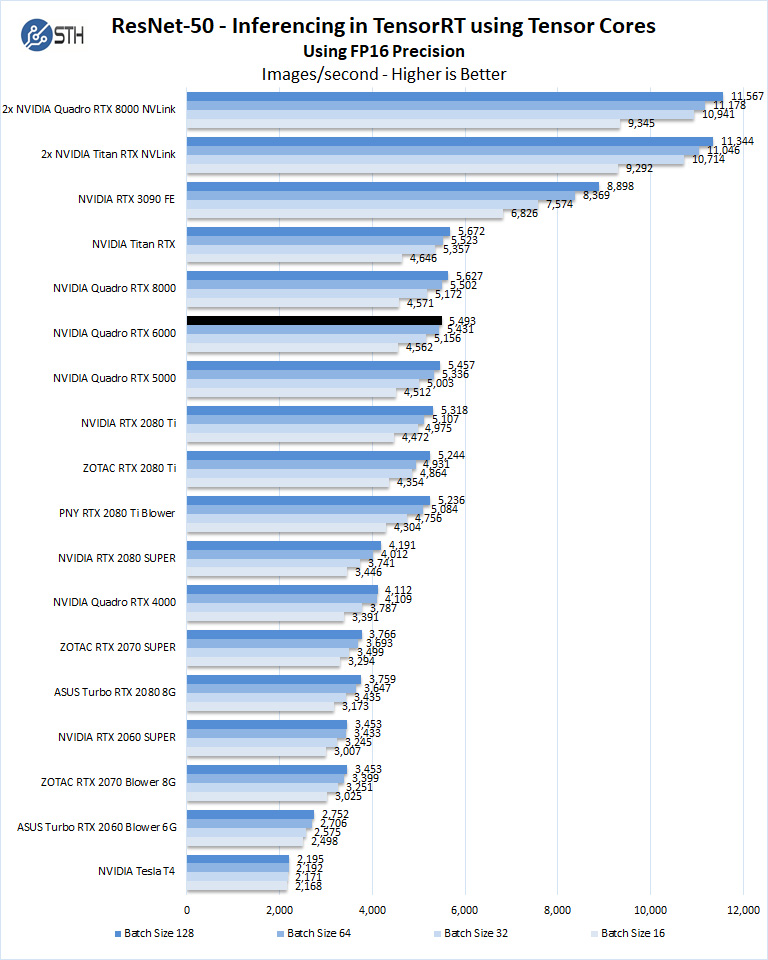
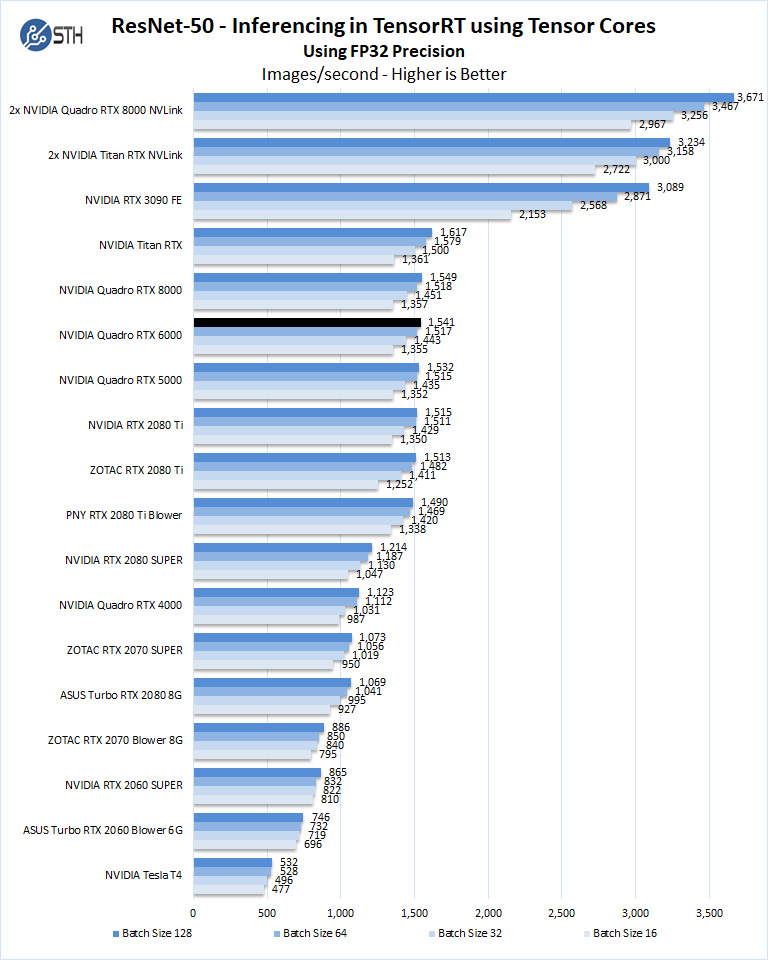
We have also added this new benchmark, ResNet-50 Training, using Tensor Cores.
ResNet-50 Training, using Tensor Cores
We also wanted to train the venerable ResNet-50 using Tensorflow. During training, the neural network is learning features of images, (e.g., objects, animals, etc.) and determining what features are important. Periodically (every 1000 iterations), the neural network will test itself against the test set to determine training loss, which affects the accuracy of training the network. Accuracy can be increased through repetition (or running a higher number of epochs.)
The command line we will use is.
nvidia-docker run --shm-size=1g --ipc=host --ulimit memlock=-1 --ulimit stack=67108864 -v ~/Downloads/imagenet12tf:/imagenet --rm -w /workspace/nvidia-examples/cnn/ nvcr.io/nvidia/tensorflow:20.11-tf2-py3 python resnet.py --data_dir=/imagenet --batch_size=128 --iter_unit=batch --num_iter=500 --display_every=20 --precision=fp16
Parameters for resnet.py:
–layers: The number of neural network layers to use, i.e. 50.
–batch_size or -b: The number of ImageNet sample images to use for training the network per iteration. Increasing the batch size will typically increase training performance.
–iter_unit or -u: Specify whether to run batches or epochs.
–num_iter or -i: The number of batches or iterations to run, i.e. 500.
–display_every: How frequently training performance will be displayed, i.e. every 20 batches.
–precision: Specify FP32 or FP16 precision, which also enables TensorCore math for Volta, Turing and AmpereGPUs.
While this script TensorFlow cannot specify individual GPUs to use, they can be specified by
setting export CUDA_VISIBLE_DEVICES= separated by commas (i.e. 0,1,2,3) within the Docker container workspace.
We will run batch sizes of 16, 32, 64, 128, and change from FP16 to FP32.
Some GPU’s like RTX 2060, RTX 2070, RTX 2080, and RTX 2080 Ti will not show some batch runs because of limited memory.
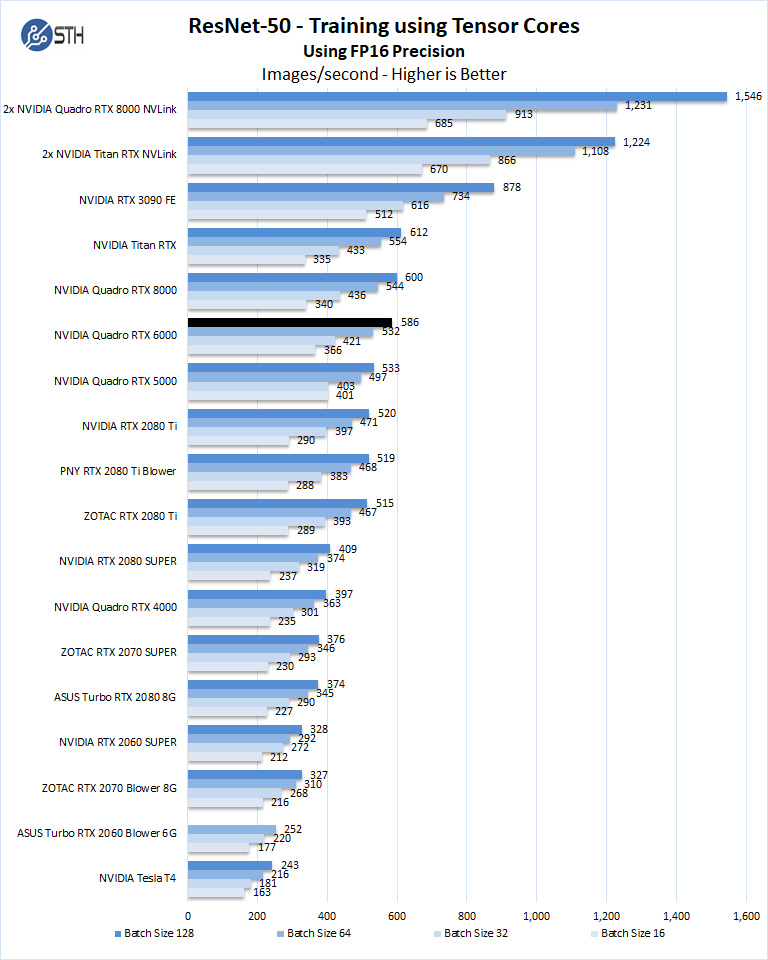
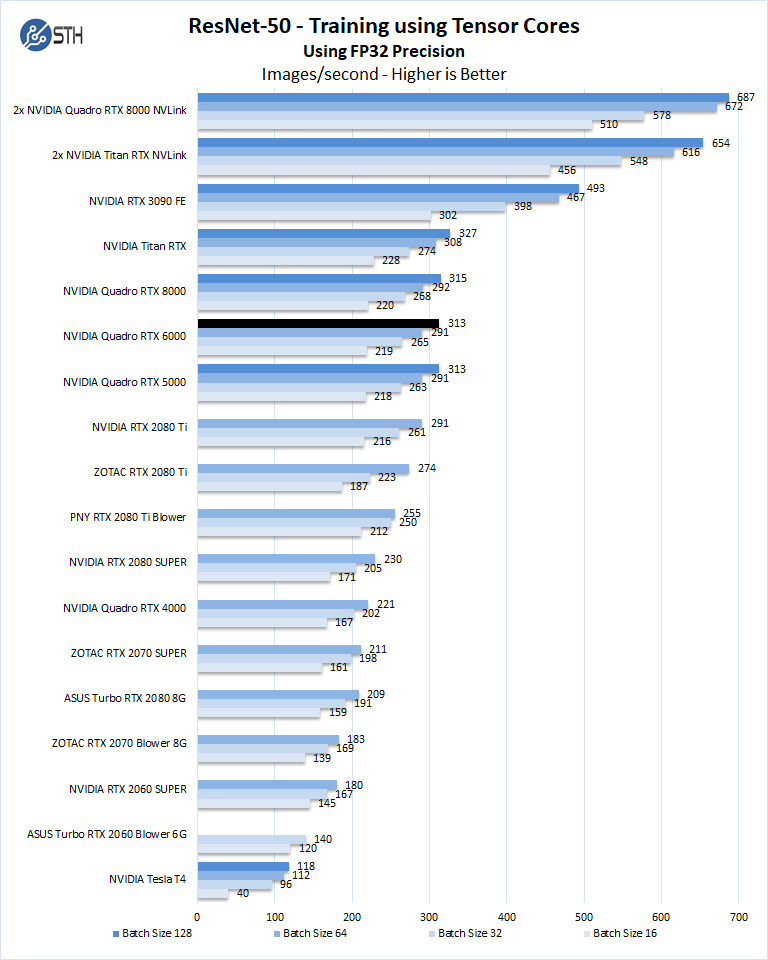
The Quadro RTX 6000 compares well to similar GPU’s like the Quadro RTX 8000 and Titan RTX.
Training using OpenSeq2Seq (GNMT)
While Resnet-50 is a Convolutional Neural Network (CNN) that is typically used for image classification, Recurrent Neural Networks (RNN) such as Google Neural Machine Translation (GNMT) are used for applications such as real-time language translations.
The command line we use for OpenSeq2Seq (GNMT) is as follows.
nvidia-docker run -it --shm-size=1g --ipc=host --ulimit memlock=-1 --ulimit stack=67108864 -v ~/Downloads/OpenSeq2Seq/wmt16_de_en:/opt/tensorflow/nvidia-examples/OpenSeq2Seq/wmt16_de_en -w /workspace/nvidia-examples/OpenSeq2Seq/ nvcr.io/nvidia/tensorflow:20.11-tf2-py3
We then open the en_de_gnmt-like-4GPUs.py and edit our variables.
vi example_configs/text2text/en-de/en-de-gnmt-like-4GPUs.py
First, edit data_root to point to the below path:
data_root = “/opt/tensorflow/nvidia-examples/OpenSeq2Seq/wmt16_de_en/”
Additionally, edit the num_gpus, max_steps, and batch_size_per_gpu parameters under
base_prams to set the number of GPUs, run a lower number of steps (i.e. 500) for
benchmarking, and also to set the batch size:
base_params = {
...
"num_gpus": 1,
"max_steps": 500,
"batch_size_per_gpu": 128,
...
},
Also, edit lines 44 and below as shown to enable FP16 precision:
#”dtype”: tf.float32, # to enable mixed precision, comment this
line and uncomment two below lines
“dtype”: “mixed”,
“loss_scaling”: “Backoff”,
We then run the benchmarks as follows.
python run.py –config_file example_configs/text2text/en-de/en-de-gnmt-like-4GPUs.py –mode train
The results will be Avg. Objects per second trained which we plot.
We should note that other GPU’s we used to, like the RTX 2060, RTX 2070, RTX 2080, and RTX2080 Ti could not complete this benchmark due to the lack of installed memory. To enable this benchmark to finish on these GPU’s one might need to lower the batch size to smaller values like 32, 16, 8. We tried this but had no luck. Using a batch size four could be run but it was decided that this was not a very usable size. We have the RTX 2060 to show that case here.
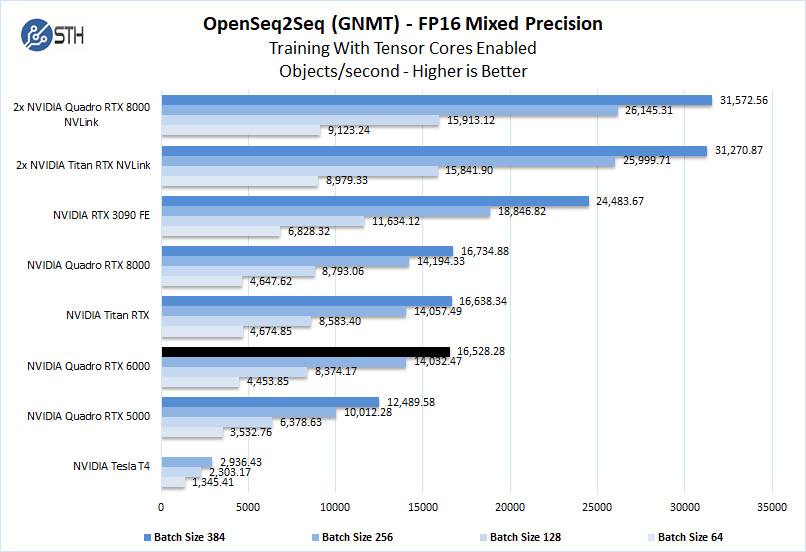
The Quadro RTX 6000 has 24GB of memory, equal to that of the Titan RTX. The Quadro RTX 8000 includes 48GB of memory, which can train much deeper workloads.
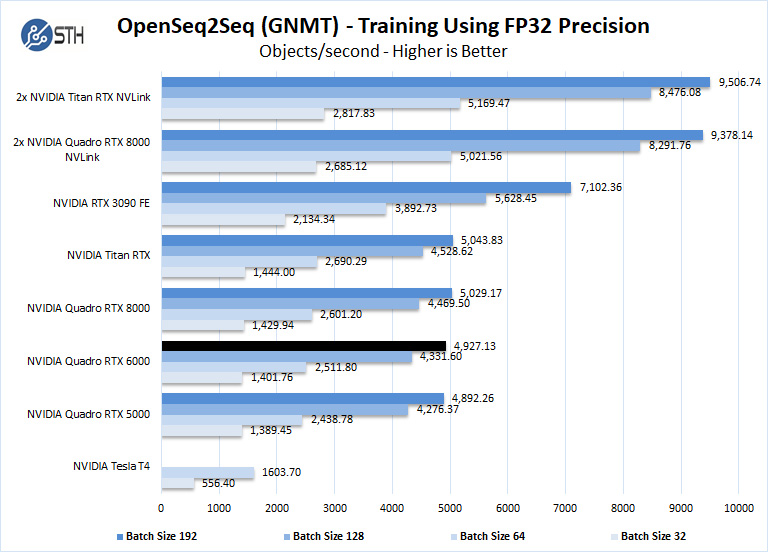
Next, we are going to look at the power consumption before getting to our final thoughts.
How many dB 1m from chassis when under load and idle?
1. Stop using “WEPYC”, it’s misleading and sounds atrocious
2. The DVD bay does not rotate to make it tower like? Dell/Supermicro have been doing that for ages, shame Lenovo could not have done the same.
3. Stop using “WEPYC”
Another good article, and nothing wring with the WEPYC nickname.
But Wepyc is just such an awesome name for what this is. AMD should pay Patrick to adopt it, in fact.
The pre-discount pricing of U$6K for 16c 32MB was eyewatering, discount pricing is still a bit stiff: https://www.lenovo.com/us/en/think-workstations/thinkstation-p-series-towers/ThinkStation-P620/p/33TS3TPP620 – the “W” is for Whoa!
It just occurred to me that the 12/16C models will suffer from the memory bandwidth problem, no? If they are indeed implemented as two CCD as the Wikipedia page suggests, then the aggregate CCD to/from IO die connectivity would be half of the 8 channel memory bandwidth. That sucks. I would have expected 12/16C models to be implemented with 4 CCD even if that means only singe CCX is used on a CCD. Bummer!
I have a P620 with the 3945WX and a W5700 on the way. I only have enough RDIMMs to do a four channel test, but I’m willing to run any benchmarks anyone here would like.
If anyone has four more 16GB RDIMMs to toss my way for benchmark purposes I could check the bandwidth question out as well.
hi Rob,
it’s 15 years since I last wrote a PO myself and to the old public Dell, however we enjoyed better than any online quotes and specials and far better than that on GPUs.
we were able to buy as a small business customer after engineering to be a non consolidated subsidiary of our parent.
many corporate organisations incorporate functions and divisions separately but breaking them out if they’re going to be consolidated anyway is not always easy to negotiate. We did and certainly could, because of a excellent structured finance department who kept us a startup in every positive and naturally inherently eminent way we were.
I mention this because if you need a high degree of acceleration and options, unless the parts aren’t available via the small business channel (PERCs were not on workstations at least at that time*) the possibility of developing a positive relationship with your account manager who may benefit from developing their familiarity with your niche market needs, feeds from this naturally and did with us on numerous occasions.
*I really should add my caveat emtor to bending sales channels that I learned when the Adaptek card lost its array tables in a way that no LSI would have done. I was asking to serve my head on a plate to the data gods when I said “go ahead” despite a consensus suspicion that that “PERC” (labelled, silk screened and BIOS declared) was inevitably going to lose the array tables, because not only didn’t anyone we could find believe that the PERC brand would be risked and the manual pages were identical to earlier parts and yet despite being never in doubt about our relationship with Dell our Dell counterparts themselves were as dumbfounded as the extent of cliché and caricatures can exemplify. I think we’ve still this very machine in storage – we mothballed it immediately afterwards in case anyone else needed to corroborate a identical failure and needed a case study to invoke insurance terms or even if Dell needed to go back to the OEM. I have never ever heard of a Adaptek in a Dell otherwise and this was in my view the time when Dell was beginning to scream out for the help that privatisation eventually delivered resoundingly.
if you are independent or able to get independent tax codes for your subsidiary unit and are looking at large – for – normal – small – business purchasing, and you have the time and manpower and the further time after purchasing, to level up your vendor team to understand your needs, and this is fine with vendor corporate, I have to say that we enjoyed superb experiences and economic terms as close to the bone as we could dare to ask. I highly recommend this strategy for a initial training project, for example.
I still omitted my points :
merely being more familiar with the technology would have almost certainly prevented that data loss – the card was fully supported but I am Perfectly Peachy Embarrassed just writing about this 15 years later because obviously I called it stupidly. Nonetheless, if our support technician and his line management worked datacenter accounts and not small business, I think numerous safeguard actions would have at least intervened to stop my stupidity. In my defense the data loss was meta data loss only, but expensive because it was the only copy of my nearly finished hand indexing of my 20 years of research files and papers and correspondence. I hadn’t backed up the most recent key to the tape archive job. Actually this whole mess was caused by my impatience with finishing work that wasn’t necessary at all and I was lucky to lose no records.
yep I am still beating myself up over that
the positive reason for this writing however I hope makes those previous pixels more acceptable, because I could have lead with more recent successes with obtaining dramatic discounts on integrated vendor hardware and maybe I should have done so.
in 2005 our Quadros MSRP $5,000 turned into line items at numbers below $2,000
we’ve been out of GPU requirements for 3 generations
however what I just said has been a reliable ballpark for the whole of this century for my company through multiple different types of incorporation and geographical location.
bluntly if you can get better discounts on GPU for today’s silicon, and I included as much caveats as I could since I can’t say anything except for the obvious which is you’d be doing extremely well to obtain comparable discounts as we have in the past, however despite my uncertainty in many respects, I am entirely sure that significant margin exists to reduce your quotes for workstations like this WEPYC today.
if you can invest in your vendor channel relationship sufficiently to the level where you can believe that your reps and team are going to build their business and break targets thanks to your involvement with them, I am entirely sure that you can secure the most meaningful economic benefits for your business that are possible for your vendor to afford you.
before you think about pricing however, do you have the capacity to reverse engineer your vendor’s team’s economics sufficiently accurately so that you will not be hitting any of their pain points during your negotiations?
are you sure that you can use independent economic intelligence to your advantage in ways that are absolutely incapable of creating in your vendors team any suspicion of leaking to you in any way whatsoever?
(buying industry market intelligence is a good option here and may potentially be helpful for your vendor team to learn from, although I don’t need to advise against didactic demeaning it’s really worth working out how this comes across beforehand and I’ve cold called at board and CxO for 35 years and I don’t find this at all easy)
I’ve blackened too many pixels here but I’ll leave this with just saying that the price of a specialist PR to write and photograph / talking head shoot the sale as a case study could repay you many times over. my company used to do all that as well if I asked I’ll respond best I can / appropriate / offline
” WEPYC ”
should have been coined in conversation with some interesting participants in the workstation EPYC ecosystem, to give it the introduction necessary.
This is a seemingly trifling but very definitely serious loss to the pandemic. Any other year and the PLATRONYM WEPYC would be serving efficient duty in the war on the market selling advanced solutions depending on the EPYC ecosystem. Chatting with the vendors most impacted – anyone needing PCIEV4 will do, but someone who has a good enough horizontal angle to want to downplay the platform and present their solutions as turnkey would be behind using WEPYC in enough ways it would have take-up plenty as of Christmas in private already.
I reacted negatively at first but quickly realised that my reaction was caused by the lack of vital context and transforming meaning.
the reaction I’m reading signals to me only that we’re hurting in unseen ways without closer industry community contacts happening
oh, I consistently was securing the First In Country Supply for the parts that we wanted.
this and our developed strategy has delivered us components frequently before very major accounts got theirs
supply has clearly stratified beyond recognition of my personally responsible days, we have delayed buying metal ourselves for over 2 years and will probably take delivery 3 years after our original planning dates, because we wanted to learn intimately what’s going on around the industry now. We’ve used cloud machines to be as early as possible as general public to learn as much as possible without any favors. Because of the stratification and the pressure of demand for next generation hardware, we’ve tried to pass on the present cycle to use this cycle to develop the knowledge and relationships – extending outward from who we work with already wherever possible – the risks we considered necessary to avoid to the point that we’re effectively contracted to key customers instead of selling to the market for now.
apart from boasting about the results I’ve helped get negotiating with vendors, I’m wanting to say that if you are likewise talking with new people and new channels, from where I see lots of people are almost sitting this one out to commit better in H222. no need to even necessarily start hinting about bigger business to come next time because I think about everybody is doing similar
hi to William and Patrick and STH team!
any chance of getting two MSI 3090s into this wepyc to test with the rest of the slots as loaded as possible?
I’ve written altogether too much but my business encompasses video production and 8K RED raw files scrub nicely only with RTX silicon which Apple doesn’t sell leaving clackintoshes (cloud + hack +) run on esxi the most sensible interim way
many people would love to serve a RTX to a colleague while checking out the state of play on Linux and Windows, Linux extensively used for paint and color work witht the high end applications coming within reach of the many due to hardware improvements so vastly lower license pricing recently (tens of kilobucks per seat to low single digit thousands now for Katana and Mark and similar to zero for NLEs…) and with bundles of legacy cards to bring over, this is a highly relevant configuration to think about. Consider everybody who could afford got a $20k RED rocket FPGA raw decode accelerator card only 3 years ago… the Brits are big in this game too and getting much less support for everything. Meanwhile the indy industry is in trouble big time and can’t afford to pay twice for workstations. this is a market in desperate need of your attention and insights.
Anyone knows the “normal” weight of this workstation? Lenovo’s documentation only lists maximal weight, which might include multiple GPUs and HDDs. I’d like to get one, however I need to put it in a holder hanging from a desk, which has strict weight limits. The maximal weight listed on Lenovo’s site is way above that maximum.
Got an answer on Lenovo forums: 29lbs / 13.2kg for a basic version. Going to get one soon.
Great machine but our newly purchased ThinkStation P620 suffers from a sudden power loss type shutdown, usually when not under any load. This happens around every 2 weeks.