At Hot Chips 2024, SK Hynix was focused on more than standard DRAM for AI accelerators. Instead, the company showed off its latest advancements in in-memory computing, this time for LLM inference with its AiMX-xPU and LPDDR-AiM. The idea is that instead of moving data from memory to compute to perform memory-related transformations, those can be done directly in the memory without having to traverse an interconnect. That makes it more power efficient and potentially faster.
We are doing these live at Hot Chips 2024 this week, so please excuse typos.
SK Hynix AI-Specific Computing Memory Solution AiMX-xPU at Hot Chips 2024
SK Hynix is professing its love LLMs since they are memory-bound.
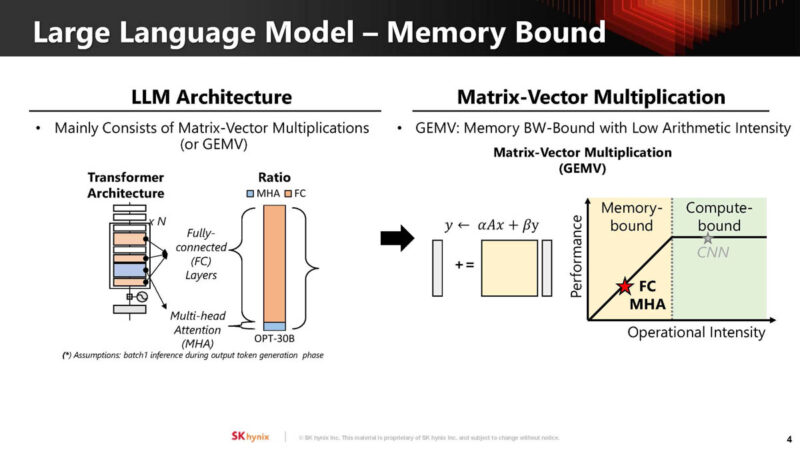
The company is showing off its GDDR6 Accelerator-in-Memory card using the Xilinx Virtex FPGAs and the special GDDR6 AiM package.
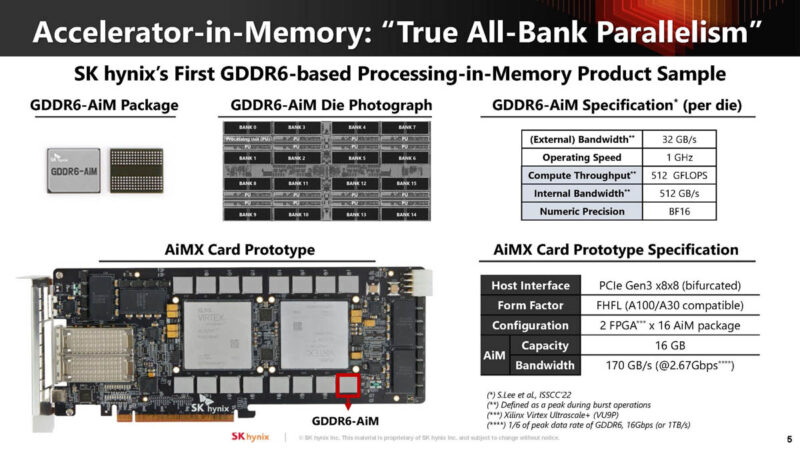
Here is a look at the card.
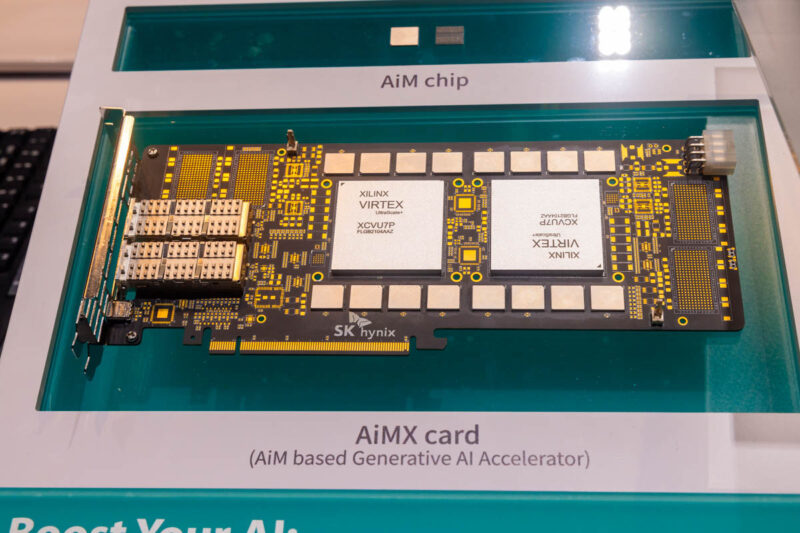
Here is a look at the GDDR6 chip. We saw these again at FMS 2024, but we already had photos of them.
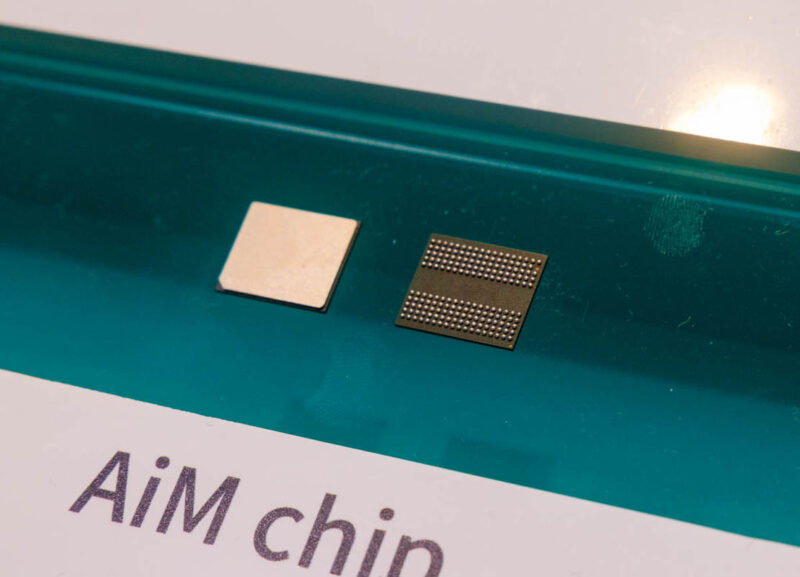
Plus, SK Hynix mentioned the OCP 2023 live demo where we took these photos.
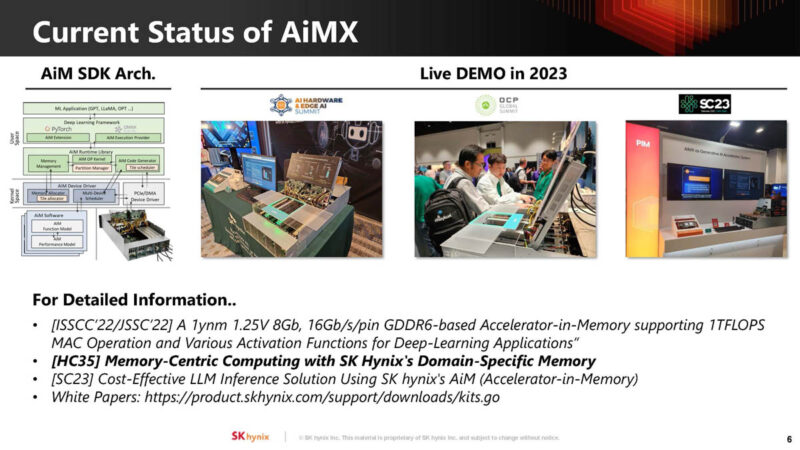
Here are the fully connected layer and multi-head attention memory and compute bound parts of LLMs.
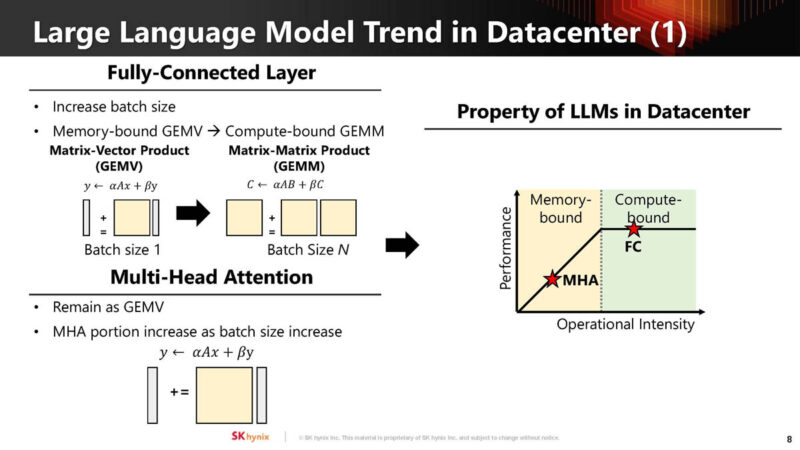
Here is how the pressure varies based on batch size.
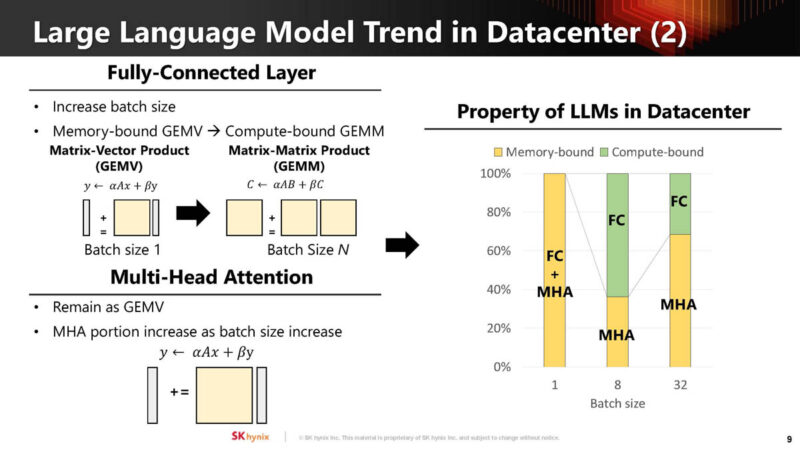
SK Hynix mapped multi-head attention into AiM.
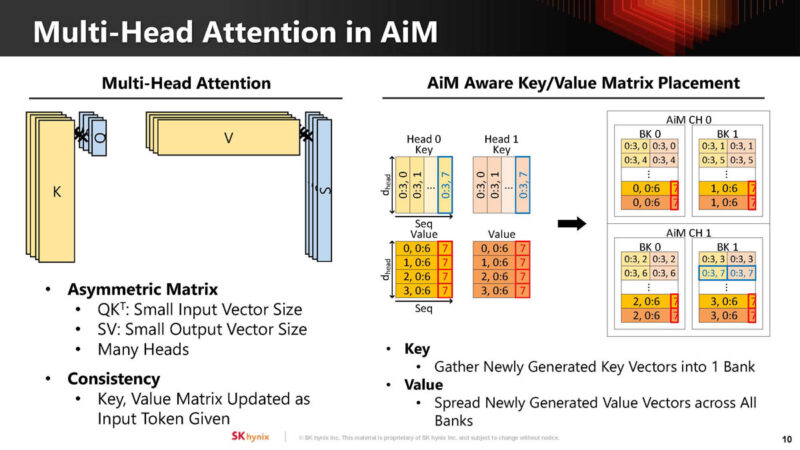
The company also doubled the memory capacity to 32GB using 32 AIM packages up from 16. 32GB is probably not enough for a product, but it is for a prototype. Still, the company was able to show the performance of the technology.
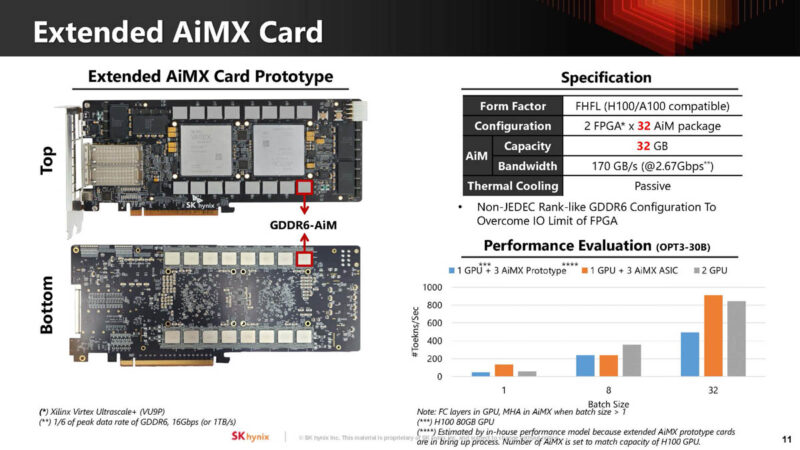
The next-gen demos are going to show things like Llama-3 and the company is also looking at scaling from 32GB to 256GB per card.
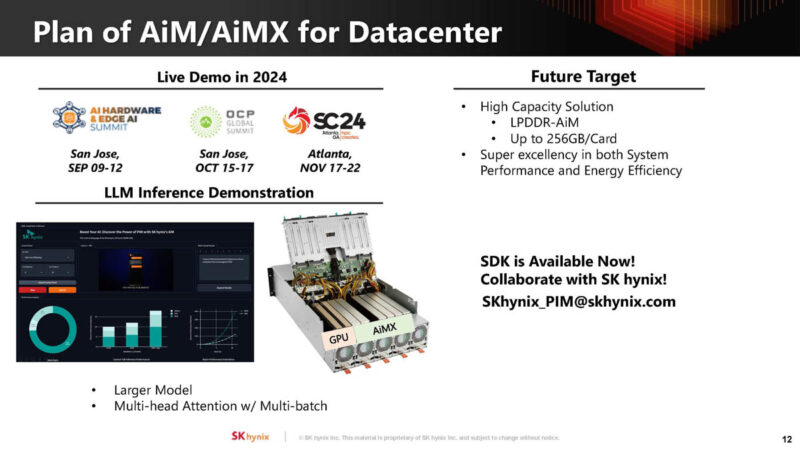
Beyond just looking at data center AI, the company is looking at on-device AI. We are already seeing companies like Apple, Intel, AMD, and Qualcomm push NPUs for AI.
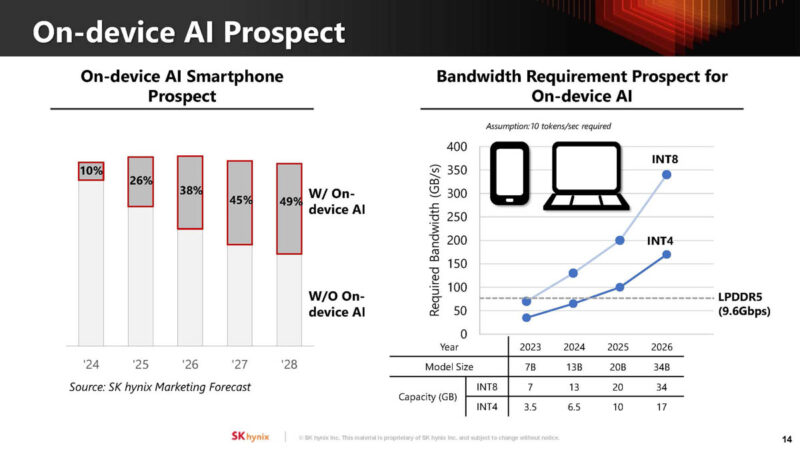
On-device AI typically lowers batch size, making those workloads memory-bound. Moving the compute off-SoC means it can be more energy efficient and not take compute die space on the SoC.
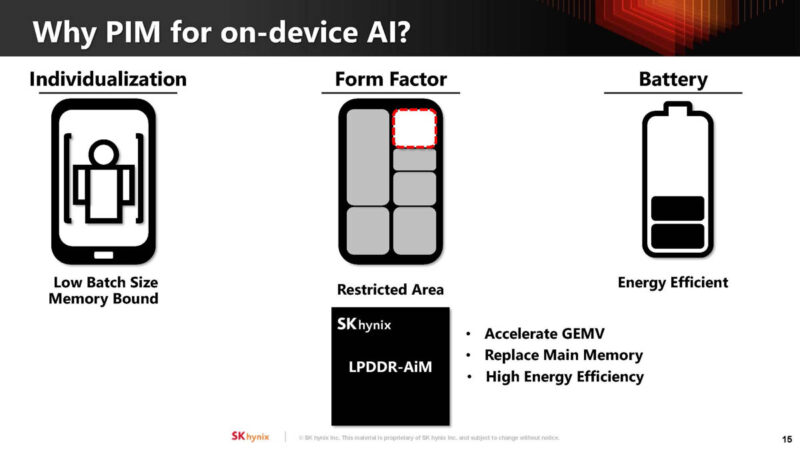
The goal is to optimize AiM in the future in a product for LPDDR5-AiM. The goal is not to change existing LPDDR commands and not to have a negative performance impact. Specs on this table are estimated.
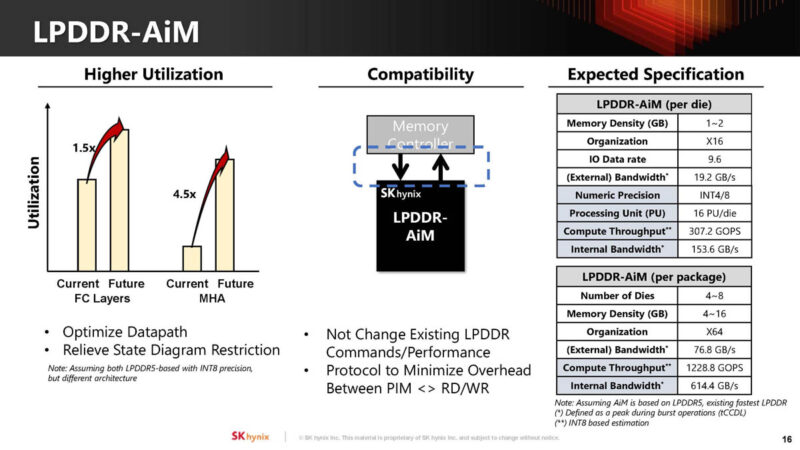
With LPDDR5, the can be integrated onto the SoC for mobile devices.
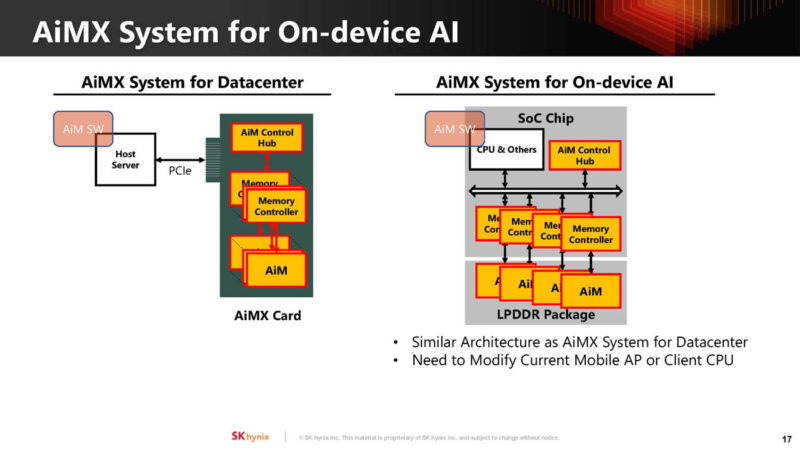
There may be a need to make different trade offs for different applications.
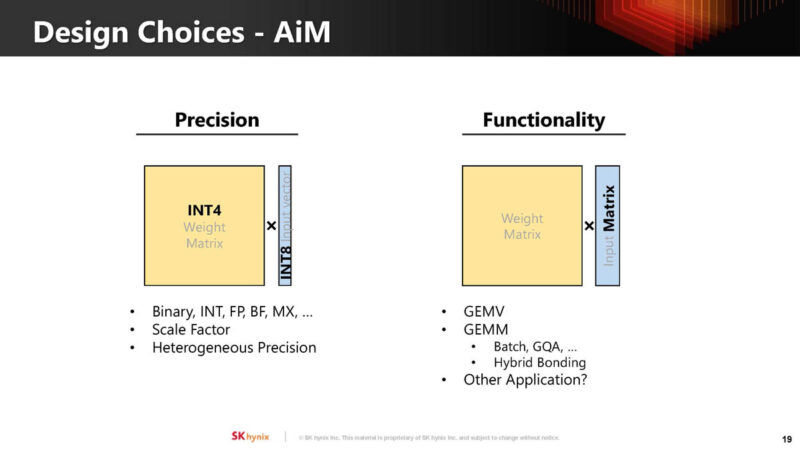
One of the challenges is to arbitrate between normal usage for LPDDR memory, as well as the compute demands. Also, there is a possibility to change the thermal and power requirements of the chips.
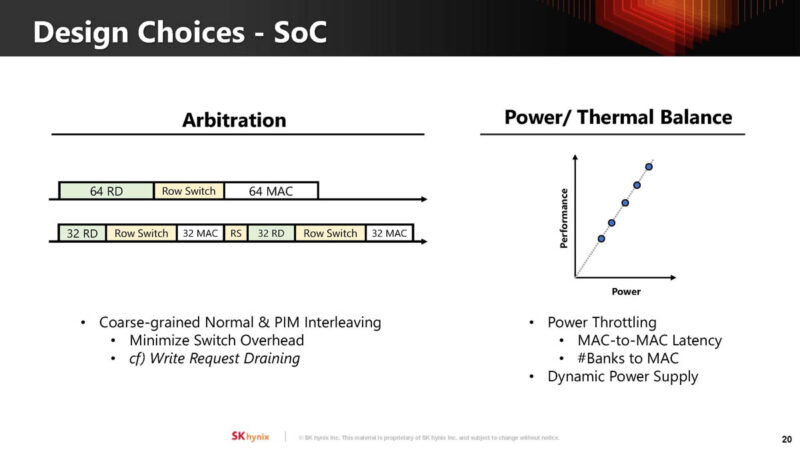
Another challenge is how to program the AiM.
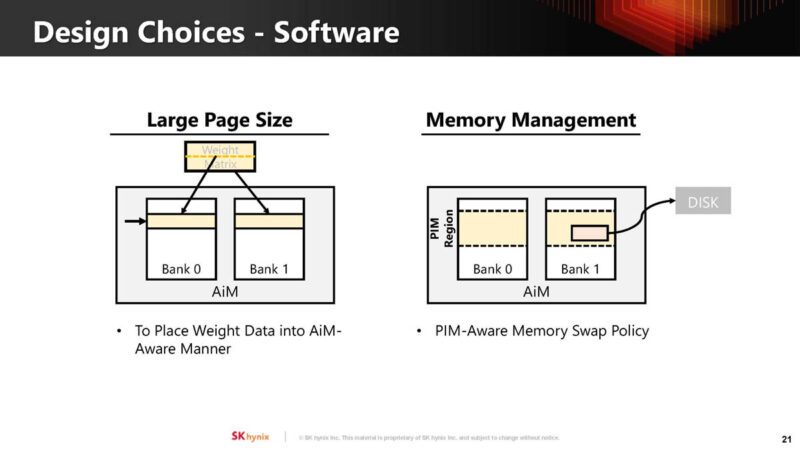
It looks like SK Hynix is expanding the line and types of places where AiM/ AiMX can be used.
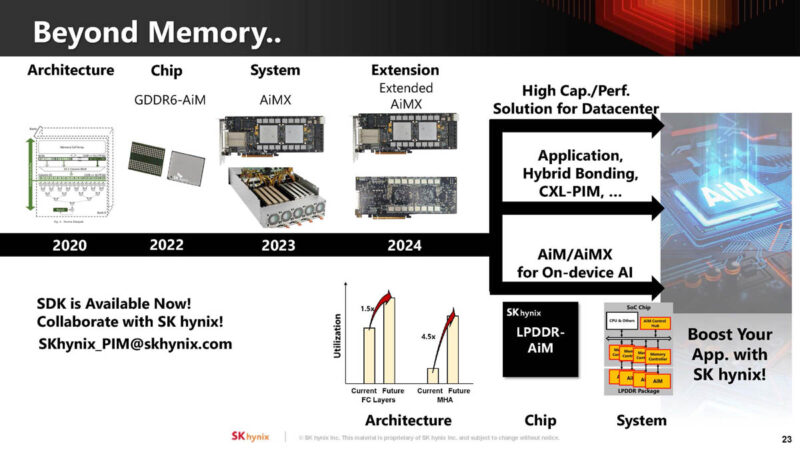
SK Hynix said that in GDDR6 AiM occupies around 20% of the die area.
Final Words
This still feels like something that a major SoC/ Chip vendor is going to have to pick up and integrate before it goes mainstream. In many ways, computing in memory may make sense. We shall see if this one goes from prototype to product in the future.
Join TopAITrends.io on a journey to discover the power of artificial intelligence. Our platform offers a carefully curated directory of AI tools, updated daily to keep you ahead in this rapidly evolving field. Whether you’re automating tasks, exploring creative tools, or looking to enhance your business, our AI solutions cater to all needs. Dive into personalized recommendations, user-driven reviews, and expert content. Discover the future of AI today with TopAITrends.io.